Image Restoration by Combined Order Regularization with Optimal Spatial Adaptation.
arXiv: Image and Video Processing(2020)
摘要
Total Variation (TV) and related extensions have been popular in image restoration due to their robust performance and wide applicability. While the original formulation is still relevant after two decades of extensive research, its extensions that combine derivatives of first and second orders are now being explored for better performance, with examples being Combined Order TV (COTV) and Total Generalized Variation (TGV). As an improvement over such multi-order convex formulations, we propose a novel non-convex regularization functional which adaptively combines Hessian-Schatten (HS) norm and first order TV (TV1) functionals with spatially varying weight. This adaptive weight itself is controlled by another regularization term; the total cost becomes the sum of this adaptively weighted HS-TV1 term, the regularization term for the adaptive weight, and the data-fitting term. The reconstruction is obtained by jointly minimizing w.r.t. the required image and the adaptive weight. We construct a block coordinate descent method for this minimization with proof of convergence, which alternates between minimization w.r.t. the required image and the adaptive weights. We derive exact computational formula for minimization w.r.t. the adaptive weight, and construct an ADMM algorithm for minimization w.r.t. to the required image. We compare the proposed method with existing regularization methods, and a recently proposed Deep GAN method using image recovery examples including MRI reconstruction and microscopy deconvolution.
更多查看译文
关键词
Image restoration,Minimization,TV,Image reconstruction,Magnetic resonance imaging,Microscopy,Magnetic force microscopy,Total variation,image restoration,multi-order regularization,Hessian-Schatten norm,spatially adaptive regularization,magnetic resonance imaging,total internal reflection fluorescence microscopy
AI 理解论文
溯源树
样例
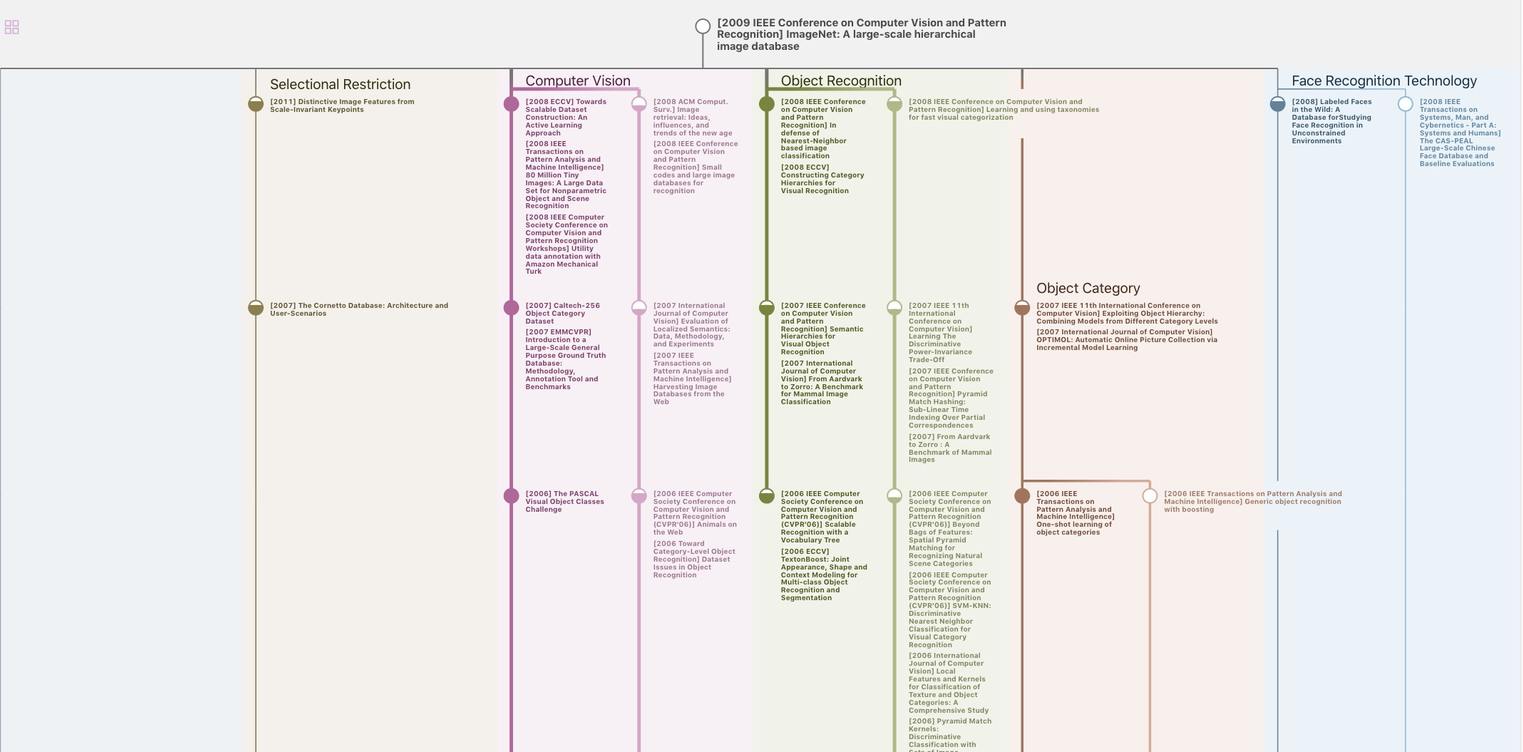
生成溯源树,研究论文发展脉络
Chat Paper
正在生成论文摘要