Domain Adaptation for Real-Time Student Performance Prediction.
arXiv: Computers and Society(2019)
摘要
Increasingly fast development and update cycle of online course contents, and diverse demographics of students in each online classroom, make student performance prediction in real-time (before the course finishes) and/or on curriculum without specific historical performance data available interesting topics for both industrial research and practical needs. In this research, we tackle the problem of real-time student performance prediction with on-going courses in a domain adaptation framework, which is a system trained on studentsu0027 labeled outcome from one set of previous coursework but is meant to be deployed on another. In particular, we first introduce recently-developed GritNet architecture which is the current state of the art for student performance prediction problem, and develop a new emph{unsupervised} domain adaptation method to transfer a GritNet trained on a past course to a new course without any (studentsu0027 outcome) label. Our results for real Udacity studentsu0027 graduation predictions show that the GritNet not only emph{generalizes} well from one course to another across different Nanodegree programs, but enhances real-time predictions explicitly in the first few weeks when accurate predictions are most challenging.
更多查看译文
AI 理解论文
溯源树
样例
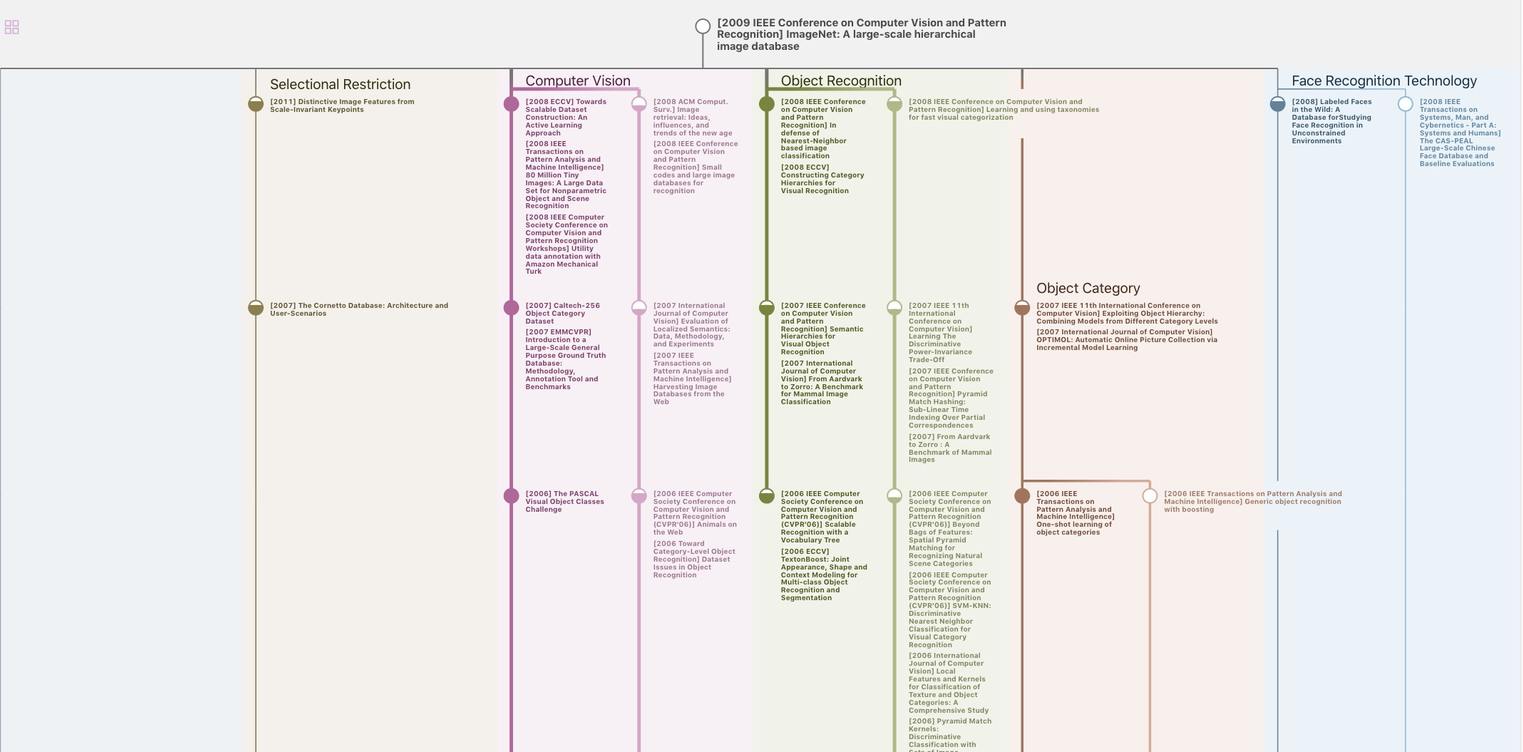
生成溯源树,研究论文发展脉络
Chat Paper
正在生成论文摘要