Development of data-driven models for prediction of daily global horizontal irradiance in northwest China
Journal of Cleaner Production(2019)
摘要
Global horizontal irradiance (Hg) data are the primary information for the design and evaluation of solar systems, which can generate clean thermal or electrical energy for agricultural and industrial production. However, Hg measurements are highly costly and time-consuming, which makes Hg data unavailable in most regions of the world. Therefore, numerous empirical and data-driven models have been established to predict Hg using other commonly available meteorological data. The present study proposed three data-driven models, i.e., extreme learning machine (ELM), random forests (RF) as well as the hybrid genetic algorithm and backpropagation neural networks (GANN), for accurate prediction of daily Hg in Northwest China. Daily minimum, maximum air temperature, sunshine duration and relative humidity were used to train and assess the models. The performance of the three data-driven models were also compared against two widely used empirical models (Angstrom–Prescott and Ogelman models). The results demonstrated that the estimated Hg had statistically significant correlations (P < 0.001) with the measured values. A sensitivity analysis indicated that sunshine duration was the most influential variable on Hg prediction in the three data-driven models. Generally, the data-driven models provided better estimates, compared with the two empirical models. The ELM model had the closest daily Hg estimates, followed by the GANN and RF models, with average global performance index (GPI) of 1.516, 1.261 and 0.000, respectively. Thus, the ELM model is highly recommended for daily Hg estimation in Northwest China.
更多查看译文
关键词
Global horizontal irradiance,Random forests,Extreme learning machine,Modeling,Global performance index
AI 理解论文
溯源树
样例
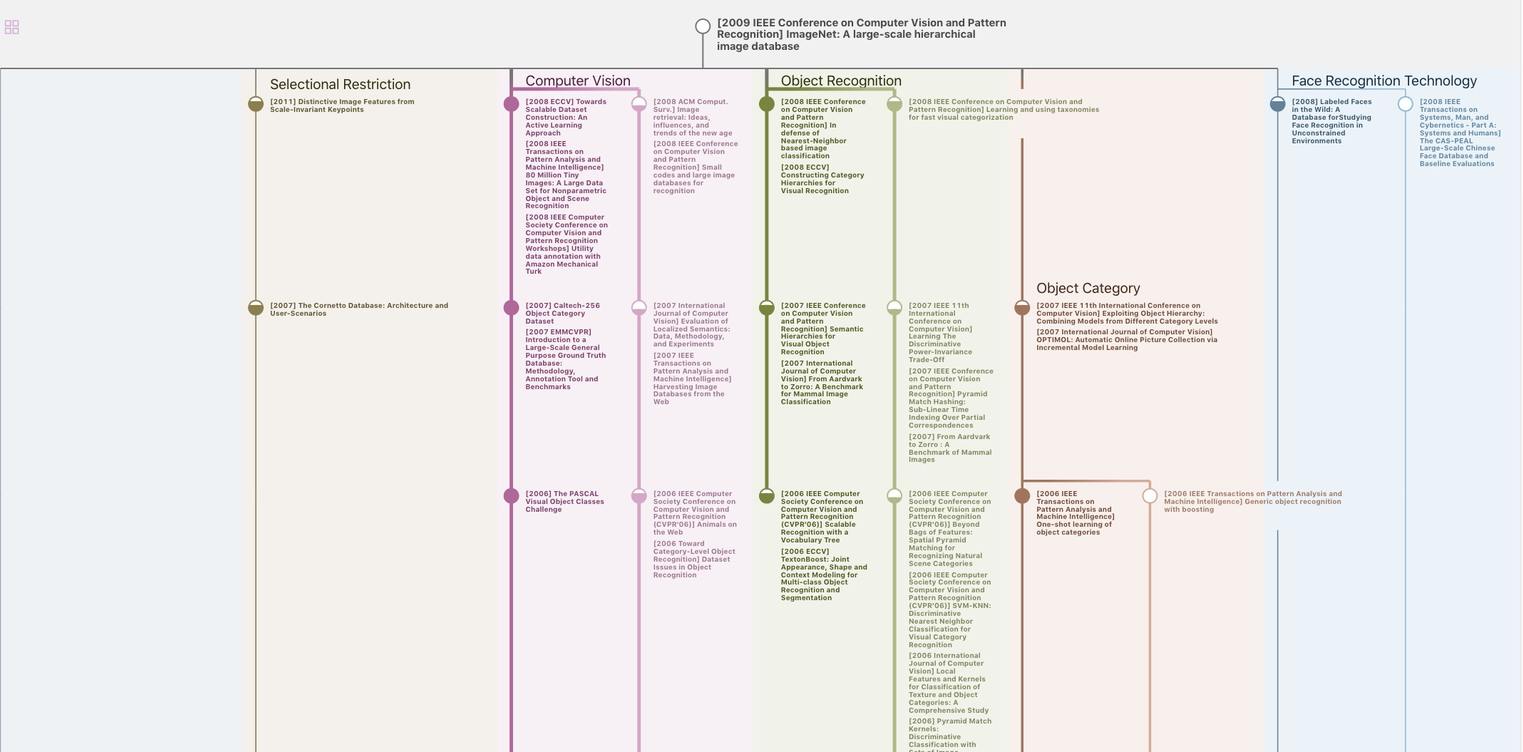
生成溯源树,研究论文发展脉络
Chat Paper
正在生成论文摘要