Robust ℓ2−Hypergraph and Its Applications
Information Sciences(2019)
摘要
Hypergraph, an important learning tool to modulate high-order data correlations, has a wide range of applications in machine learning and computer vision. The key issue of the hypergraph-based applications is to construct an informative hypergraph, in which the hyperedges effectively represent the high-order data correlations. In practice, the real-world data is usually sampled from a union of non-linear manifolds. Due to the issues of noise and data corruptions, many data samples deviate from the underlying data manifolds. To construct an informative hypergraph that represents real-world data distribution well, we propose a hypergraph model (ℓ2-Hypergraph). Our model generates each hyperedge by solving an affine subspace ridge regression problem, where the samples with non-zero representation coefficients are used for hyperege generation. Specifically, to be robust to sparse noise and corruptions, a sparse constraint is imposed on data errors. We have conducted image clustering and classification experiments on real-world datasets. The experimental results demonstrate that our hypergraph model is superior to the existing hypergraph construction methods in both accuracy and robustness to sparse noise.
更多查看译文
关键词
Hypergraph,Hyperedge,Representation coefficients,Ridge regression
AI 理解论文
溯源树
样例
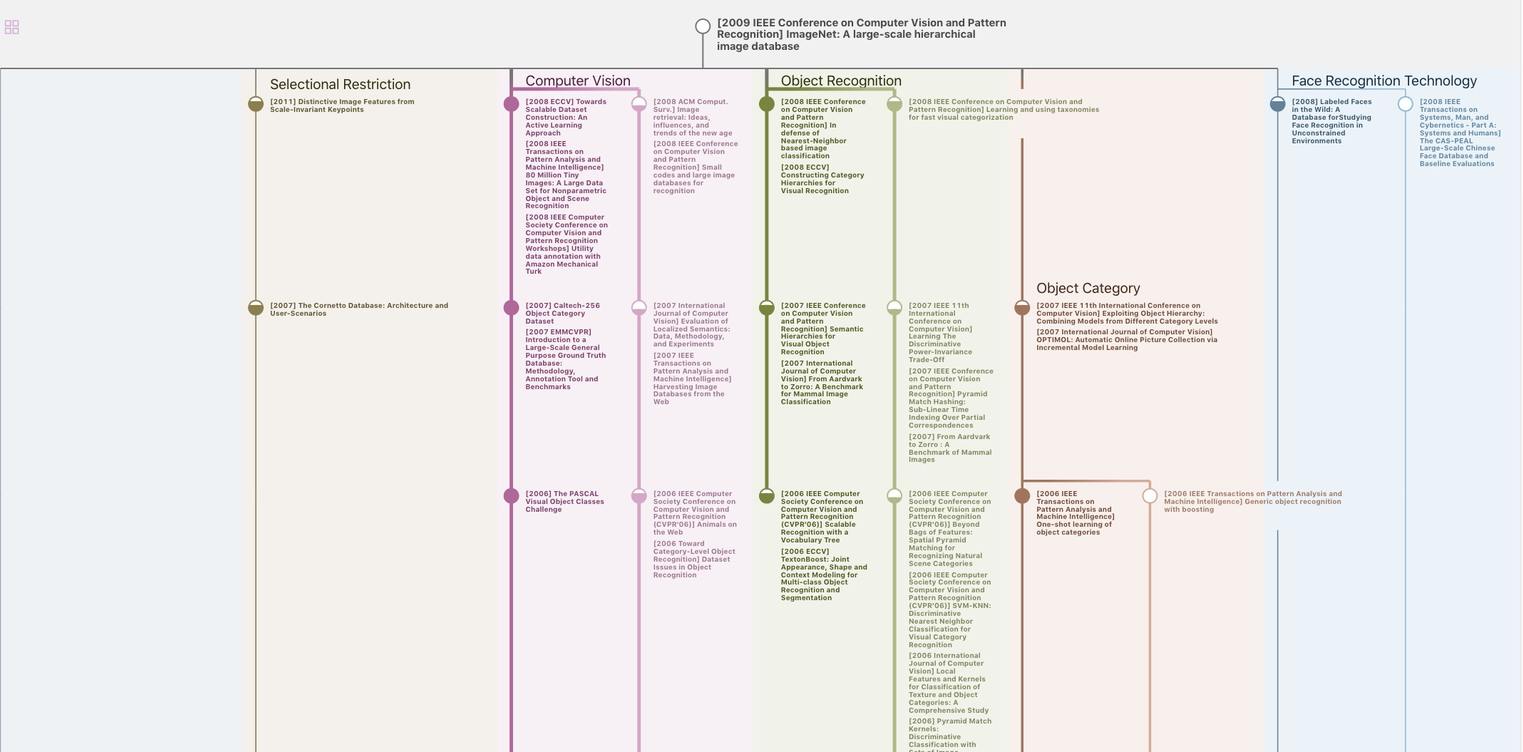
生成溯源树,研究论文发展脉络
Chat Paper
正在生成论文摘要