Changepoint detection versus reinforcement learning: Separable neural substrates approximate different forms of Bayesian inference
bioRxiv(2019)
摘要
Adaptive behavior in even the simplest decision-making tasks requires predicting future events in an environment that is generally nonstationary. As an inductive problem, this prediction requires a commitment to the statistical process underlying environmental change. This challenge can be formalized in a Bayesian framework as a question of choosing a generative model for the task dynamics. Previous learning models assume, implicitly or explicitly, that nonstationarity follows either a continuous diffusion process or a discrete changepoint process. Each approach is slow to adapt when its assumptions are violated. A new mixture of Bayesian experts framework proposes separable brain systems approximating inference under different assumptions regarding the statistical structure of the environment. This model explains data from a laboratory foraging task, in which rats experienced a change in reward contingencies after pharmacological disruption of dorsolateral (DLS) or dorsomedial striatum (DMS). The data and model suggest DLS learns under a diffusion prior whereas DMS learns under a changepoint prior. The combination of these two systems offers a new explanation for how the brain handles inference in an uncertain environment.
更多查看译文
AI 理解论文
溯源树
样例
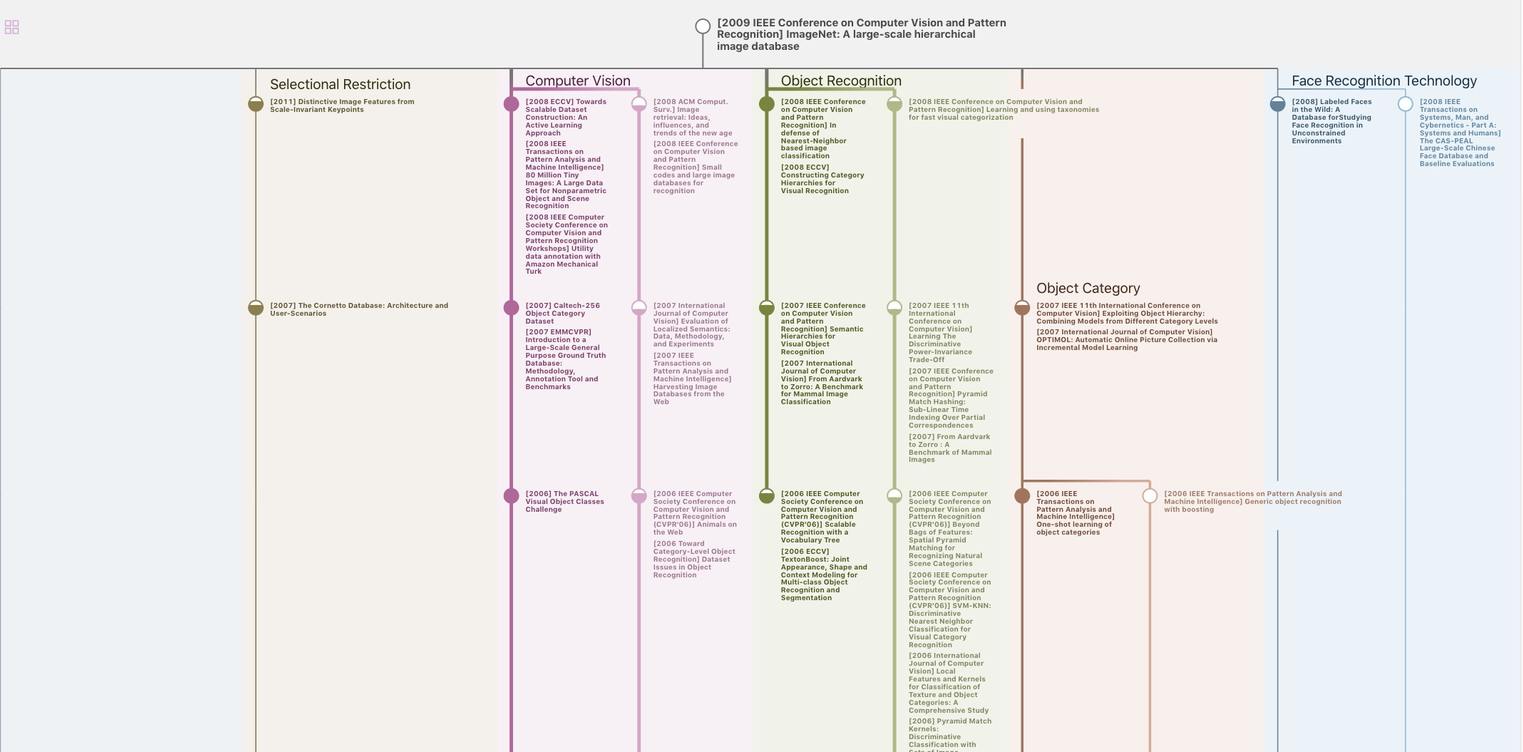
生成溯源树,研究论文发展脉络
Chat Paper
正在生成论文摘要