Efficient Dynamic Malware Analysis For Collecting Http Requests Using Deep Learning
IEICE TRANSACTIONS ON INFORMATION AND SYSTEMS(2019)
摘要
Malware-infected hosts have typically been detected using network-based Intrusion Detection Systems on the basis of characteristic patterns of HTTP requests collected with dynamic malware analysis. Since attackers continuously modify malicious HTTP requests to evade detection, novel HTTP requests sent from new malware samples need to be exhaustively collected in order to maintain a high detection rate. However, analyzing all new malware samples for a long period is infeasible in a limited amount of time. Therefore, we propose a system for efficiently collecting HTTP requests with dynamic malware analysis. Specifically, our system analyzes a malware sample for a short period and then determines whether the analysis should be continued or suspended. Our system identifies malware samples whose analyses should be continued on the basis of the network behavior in their short-period analyses. To make an accurate determination, we focus on the fact that malware communications resemble natural language from the viewpoint of data structure. We apply the recursive neural network, which has recently exhibited high classification performance in the field of natural language processing, to our proposed system. In the evaluation with 42,856 malware samples, our proposed system collected 94% of novel HTTP requests and reduced analysis time by 82% in comparison with the system that continues all analyses.
更多查看译文
关键词
infected host detection, network behavior, sequential data, recursive neural network
AI 理解论文
溯源树
样例
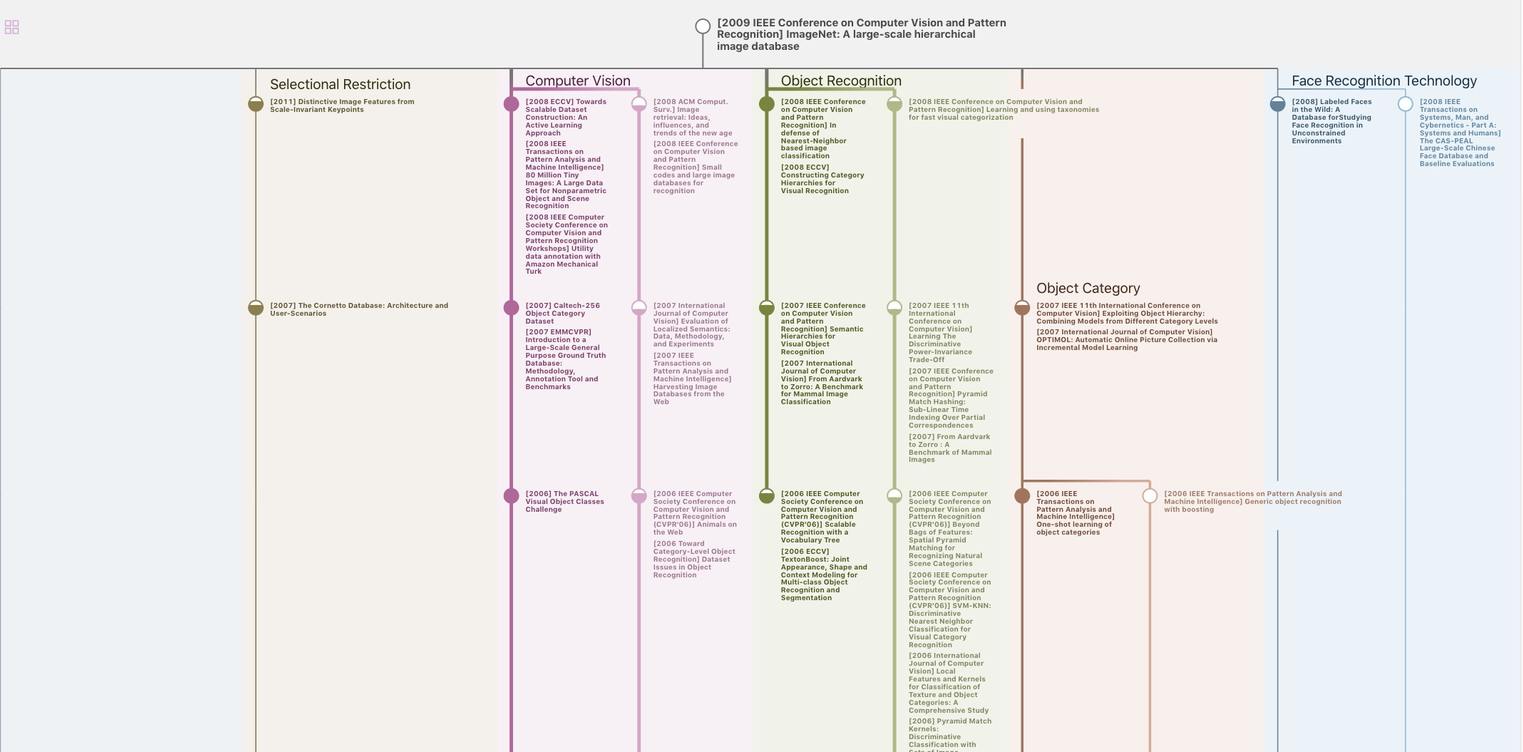
生成溯源树,研究论文发展脉络
Chat Paper
正在生成论文摘要