Deep Learning System for Travel Speed Predictions on Multiple Arterial Road Segments
TRANSPORTATION RESEARCH RECORD(2019)
摘要
Accurate travel speed prediction is a critical tool for incidence response management. The complex dynamics of transport systems render model-based prediction extremely challenging. However, the large amounts of available vehicle speed data contain the complex interdependencies of the target travel speed; the data itself can be used to generate accurate predictions using deep learning methods. In this work, a deep learning methodology involving feature generation, model development, and model deployment is presented. The authors demonstrate the high performance of deep learning methods (relative to more traditional benchmarks) in predicting travel speeds from 5-30 min in advance, for a challenging arterial road network. In this study, different deep learning architectures that exploit both spatial and temporal information for several time frames are compared and analyzed. Finally, the authors demonstrate the integration of their deep learning method into a visualization system that can be directly applied for vehicle speed prediction in real time. The model-selection analysis and data-to-visualization framework in this manuscript provide a step towards decision support for incident management; for practical implementation, the predictive power of deep learning models under incident conditions should continue to be investigated and improved.
更多查看译文
AI 理解论文
溯源树
样例
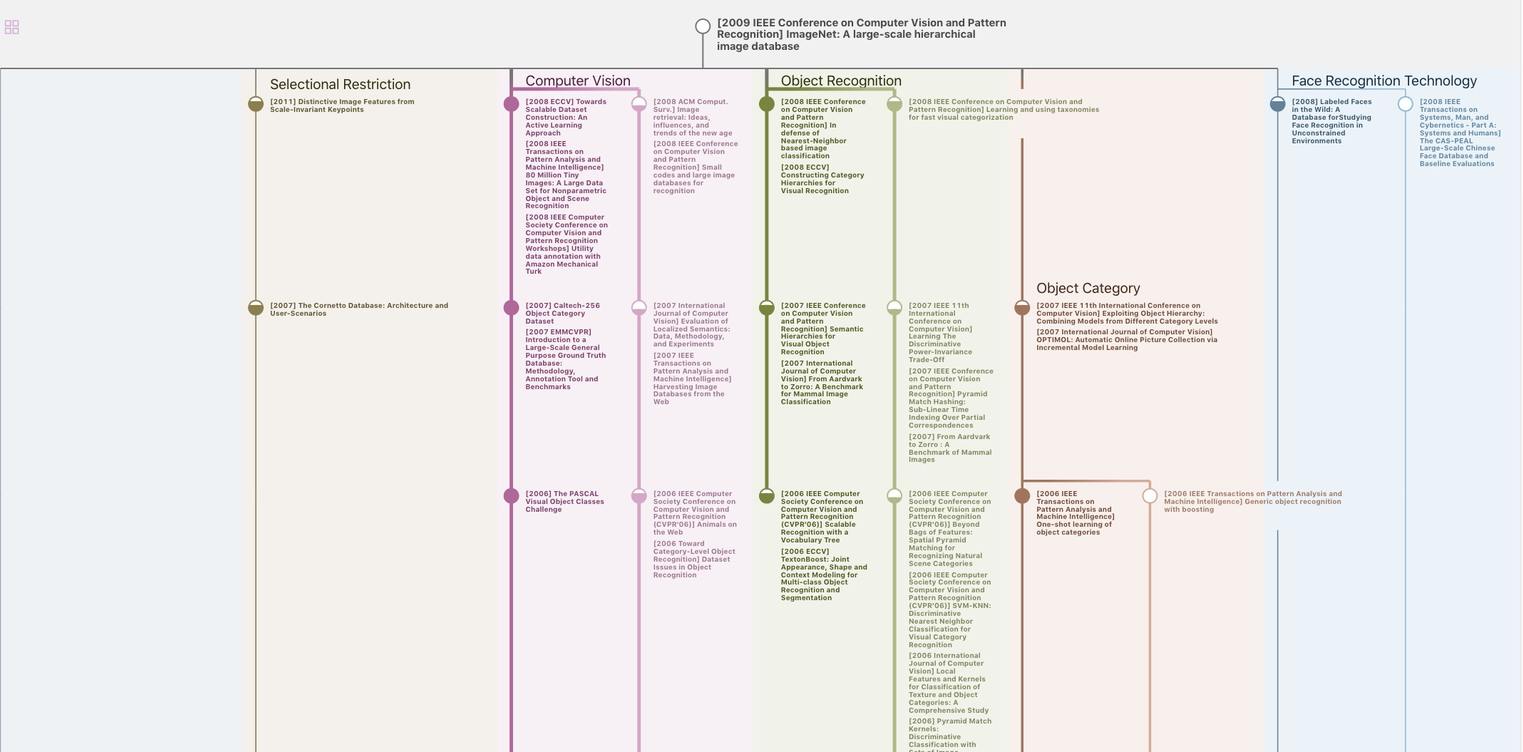
生成溯源树,研究论文发展脉络
Chat Paper
正在生成论文摘要