A Novel Hybrid Sequential Model for Review-Based Rating Prediction.
pacific-asia conference on knowledge discovery and data mining(2019)
摘要
Nowadays, the online interactions between users and items become diverse, and may include textual reviews as well as numerical ratings. Reviews often express various opinions and sentiments, which can alleviate the sparsity problem of recommendations to some extent. In this paper, we address the personalized review-based rating prediction problem, namely, leveraging users' historical reviews and corresponding ratings to predict their future ratings for items they have not interacted with before. While much effort has been devoted to this challenging problem mainly to investigate how to jointly model natural text and user personalization, most of them ignored sequential characteristics hidden in users' review and rating sequences. To bridge this gap, we propose a novel Hybrid Review-based Sequential Model (HRSM) to capture future trajectories of users and items. This is achieved by feeding both users' and items' review sequences to a Long Short-Term Memory (LSTM) model that captures dynamics, in addition to incorporating a more traditional low-rank factorization that captures stationary states. The experimental results on real public datasets demonstrate that our model outperforms the state-of-the-art baselines.
更多查看译文
关键词
Recommender systems, Rating prediction, Review analysis, Sequential model
AI 理解论文
溯源树
样例
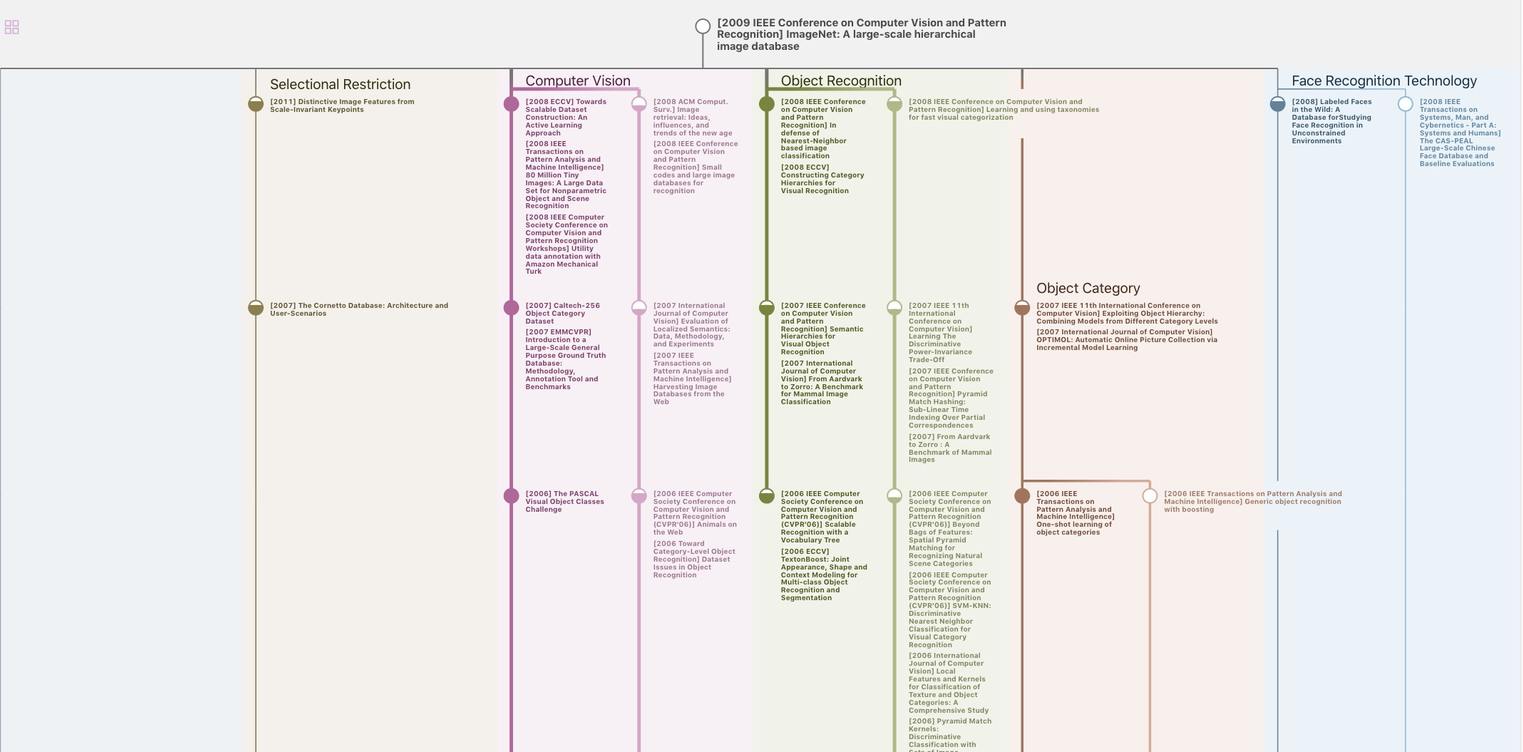
生成溯源树,研究论文发展脉络
Chat Paper
正在生成论文摘要