A Neural Approach to Entity Linking on Wikidata.
european conference on information retrieval(2019)
摘要
We tackle Named Entity Disambiguation (NED) by comparing entities in short sentences with Wikidata graphs. Creating a context vector from graphs through deep learning is a challenging problem that has never been applied to NED. Our main contribution is to present an experimental study of recent neural techniques, as well as a discussion about which graph features are most important for the disambiguation task. In addition, a new dataset (Wikidata-Disamb) is created to allow a clean and scalable evaluation of NED with Wikidata entries, and to be used as a reference in future research. In the end our results show that a Bi-directional Long Short-TermMemory (Bi-LSTM) encoding of the graph triplets performs best, improving upon the baseline models and scoring an F1 value of 91.6% on the Wikidata-Disamb test set (The dataset and the code (with configurations) for this paper can be found at https://github.com/contextscout/ned-graphs).
更多查看译文
关键词
Named Entity Disambiguation, Graphs, Wikidata, RNN, GCN
AI 理解论文
溯源树
样例
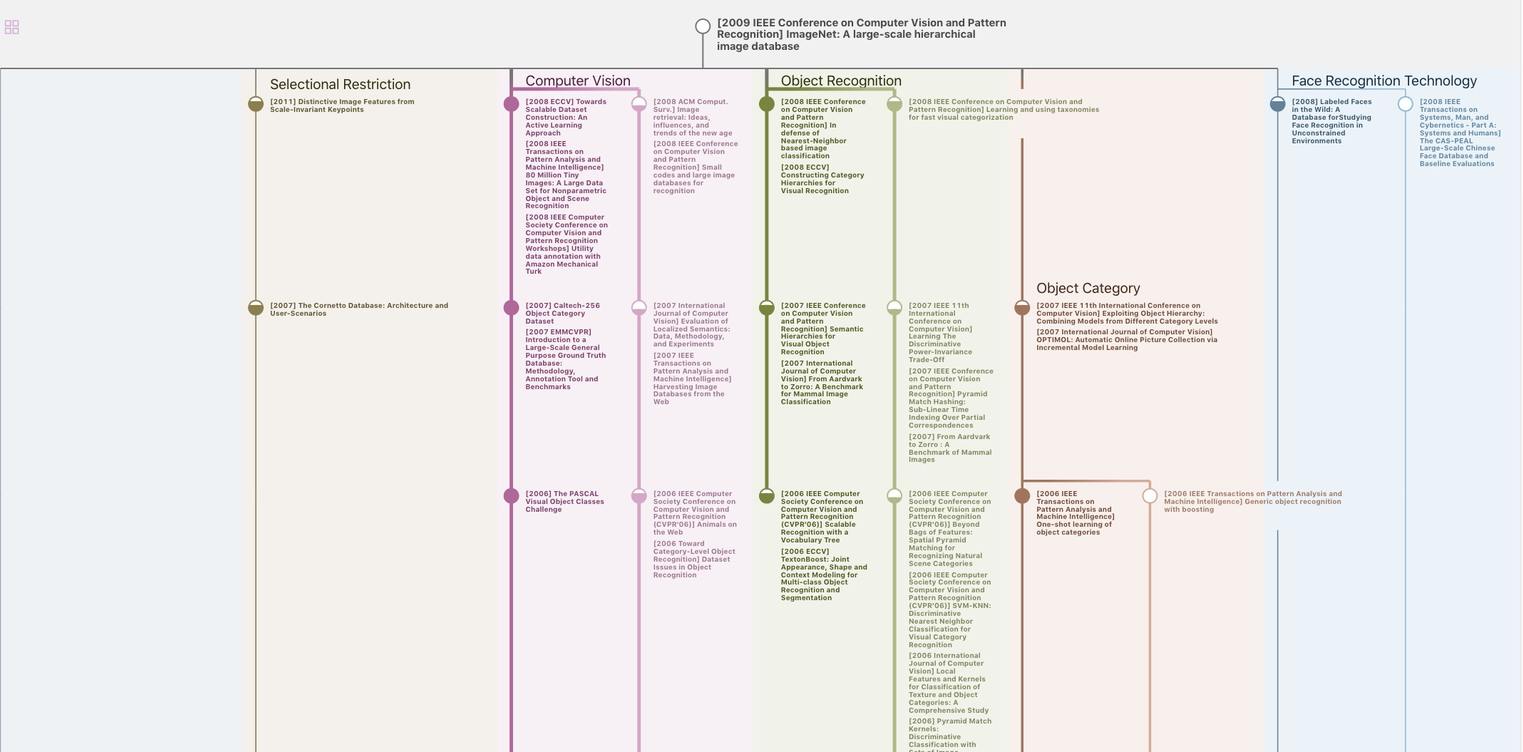
生成溯源树,研究论文发展脉络
Chat Paper
正在生成论文摘要