Selective Training: A Strategy for Fast Backpropagation on Sentence Embeddings.
pacific-asia conference on knowledge discovery and data mining(2019)
摘要
Representation or embedding based machine learning models, such as language models or convolutional neural networks have shown great potential for improved performance. However, for complex models on large datasets training time can be extensive, approaching weeks, which is often infeasible in practice. In this work, we present a method to reduce training time substantially by selecting training instances that provide relevant information for training. Selection is based on the similarity of the learned representations over input instances, thus allowing for learning a non-trivial weighting scheme from multi-dimensional representations. We demonstrate the efficiency and effectivity of our approach in several text classification tasks using recursive neural networks. Our experiments show that by removing approximately one fifth of the training data the objective function converges up to six times faster without sacrificing accuracy.
更多查看译文
关键词
Selective training, Machine learning, Neural network, Recursive models
AI 理解论文
溯源树
样例
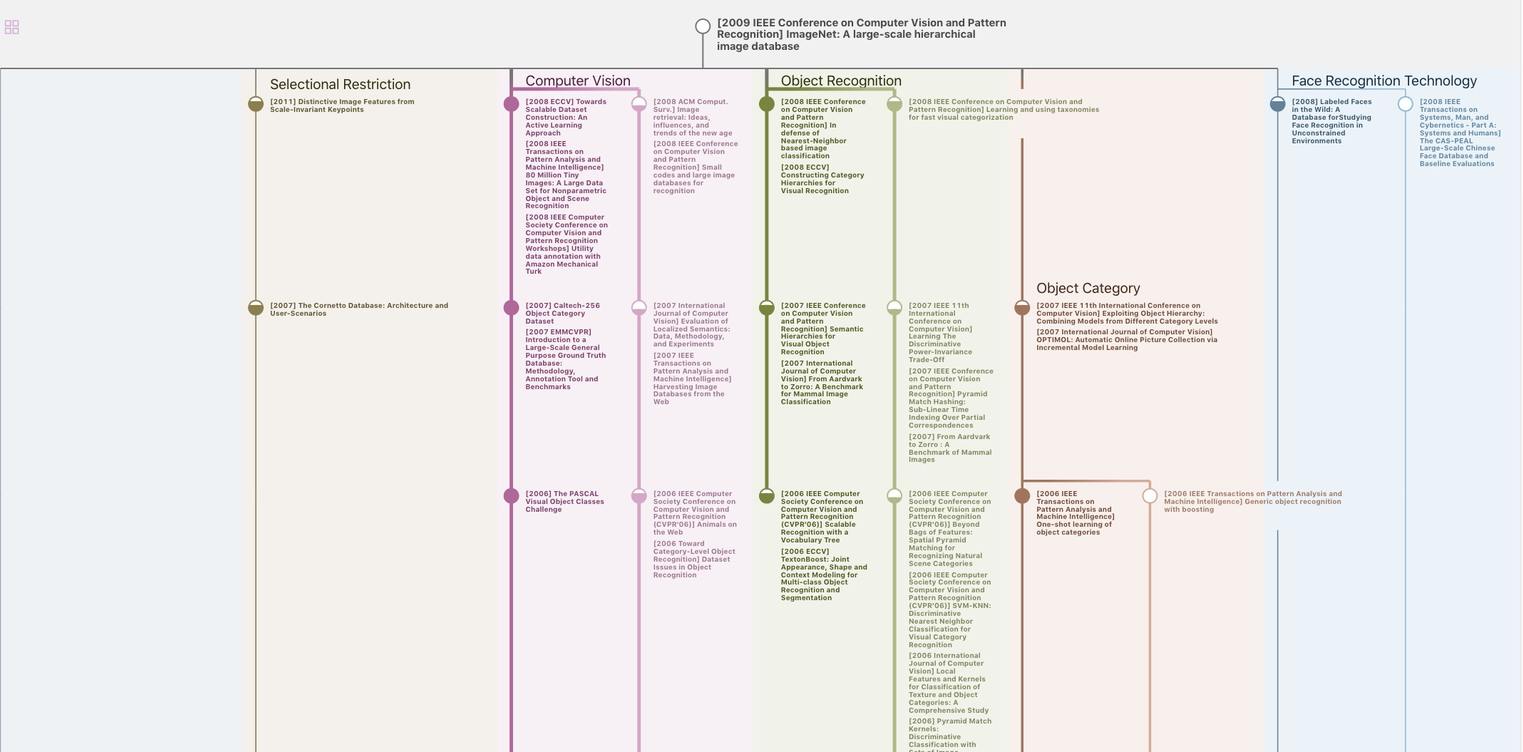
生成溯源树,研究论文发展脉络
Chat Paper
正在生成论文摘要