EMT: A Tail-Oriented Method for Specific Domain Knowledge Graph Completion
pacific-asia conference on knowledge discovery and data mining(2019)
摘要
The basic unit of knowledge graph is triplet, including head entity, relation and tail entity. Centering on knowledge graph, knowledge graph completion has attracted more and more attention and made great progress. However, these models are all verified by open domain data sets. When applied in specific domain case, they will be challenged by practical data distributions. For example, due to poor presentation of tail entities caused by their relation-oriented feature, they can not deal with the completion of enzyme knowledge graph. Inspired by question answering and rectilinear propagation of lights, this paper puts forward a tail-oriented method - Embedding for Multi-Tails knowledge graph (EMT). Specifically, it first represents head and relation in question space; then, finishes projection to answer one by tail-related matrix; finally, gets tail entity via translating operation in answer space. To overcome time-space complexity of EMT, this paper includes two improved models: EMT(^v) and EMT(^s). Taking some optimal translation and composition models as baselines, link prediction and triplets classification on an enzyme knowledge graph sample and Kinship proved our performance improvements, especially in tails prediction.
更多查看译文
关键词
Knowledge graph, Knowledge graph completion, Specific domain knowledge graph, Embedding, Tail-oriented
AI 理解论文
溯源树
样例
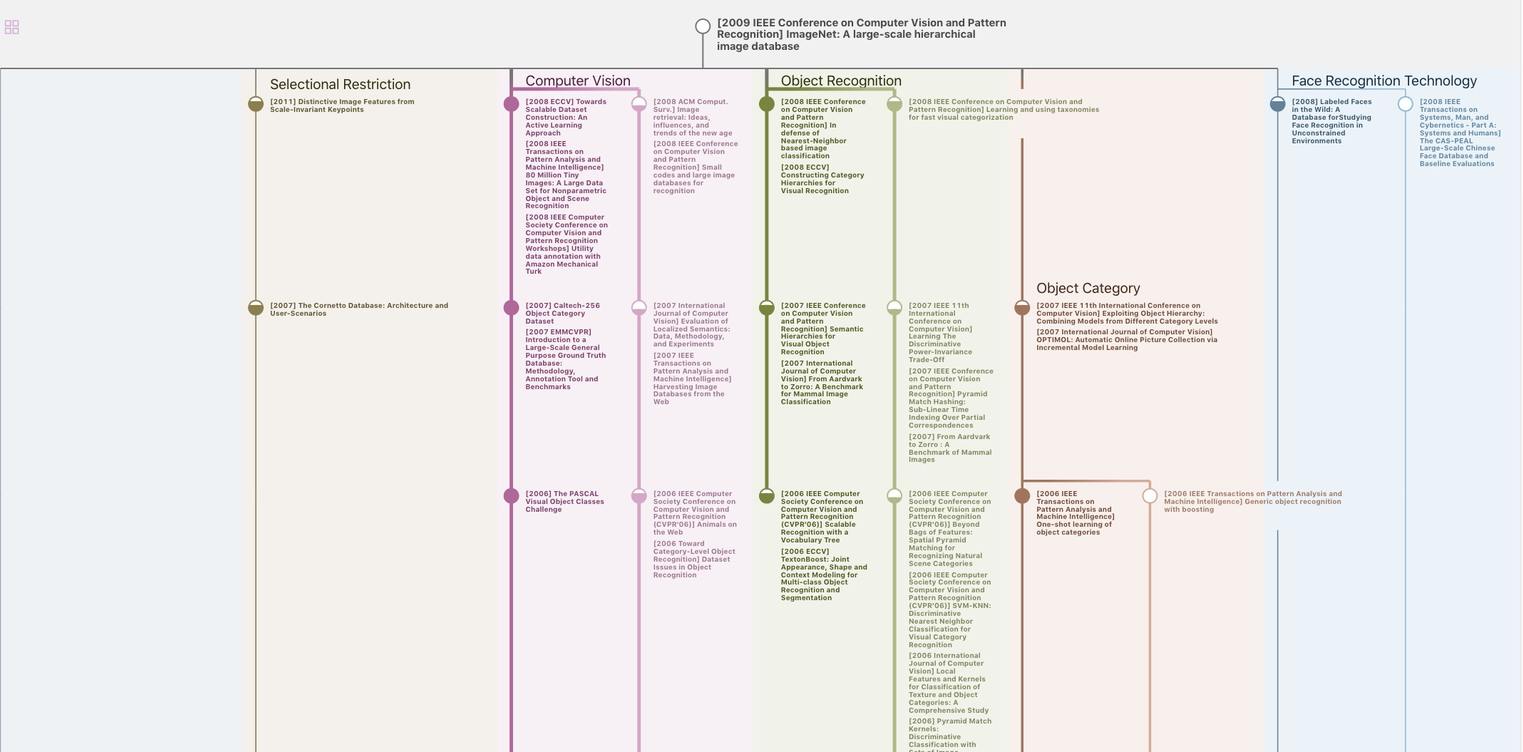
生成溯源树,研究论文发展脉络
Chat Paper
正在生成论文摘要