Latent Gaussian-Multinomial Generative Model for Annotated Data
pacific-asia conference on knowledge discovery and data mining(2019)
摘要
Traditional generative models annotate images by multiple instances independently segmented, but these models have been becoming prohibitively expensive and time-consuming along with the growth of Internet data. Focusing on the annotated data, we propose a latent Gaussian-Multinomial generative model (LGMG), which generates the image-annotations using a multimodal probabilistic models. Specifically, we use a continuous latent variable with prior of Normal distribution as the latent representation summarizing the high-level semantics of images, and a discrete latent variable with prior of Multinomial distribution as the topics indicator for annotation. We compute the variational posteriors from a mapping structure among latent representation, topics indicator and image-annotation. The stochastic gradient variational Bayes estimator on variational objective is realized by combining the reparameterization trick and Monte Carlo estimator. Finally, we demonstrate the performance of LGMG on LabelMe in terms of held-out likelihood, automatic image annotation with the state-of-the-art models.
更多查看译文
关键词
Annotated data, Gaussian-Multinomial, Multimodal generative models, Latent representation, Topics indicator
AI 理解论文
溯源树
样例
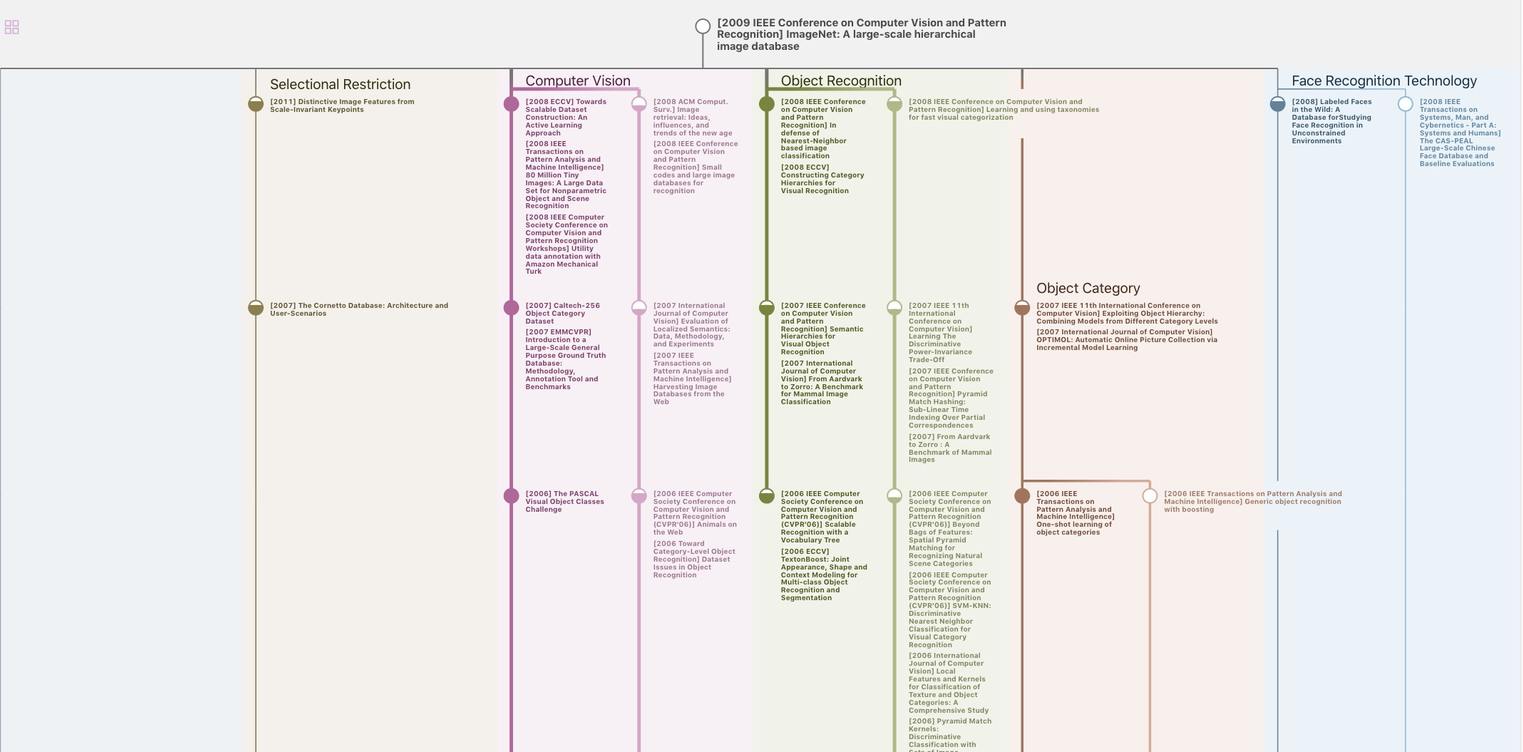
生成溯源树,研究论文发展脉络
Chat Paper
正在生成论文摘要