Identifying Mobility of Drug Addicts with Multilevel Spatial-Temporal Convolutional Neural Network.
pacific-asia conference on knowledge discovery and data mining(2019)
摘要
Human identification according to their mobility patterns is of great importance for a wide spectrum of spatial-temporal based applications. For example, detecting drug addicts from normal residents in public security area. However, extracting and classifying user behaviors in massive amount of moving records is not trivial because of three challenges: (1) the complex transition records with noisy data; (2) the heterogeneity and sparsity of spatiotemporal trajectory features; and (3) extremely imbalanced data distribution of real world data. In this paper, we propose MST-CNN, a multi-level convolutional neural network with spatial and temporal features. We first embed the multiple factors on single trajectory level and then generate a behavior matrix to capture the user's mobility patterns. Finally, a CNN module is used to extract various features with different filters and classify user type. We perform experiments on real-life mobility datasets provided by public security office, and extensive evaluation results demonstrate that our method obtains significant improvement performance in identification accuracy and outperform all baseline methods.
更多查看译文
关键词
Convolutional neural network, Spatiotemporal embedding, Human trajectory pattern, Addict identification
AI 理解论文
溯源树
样例
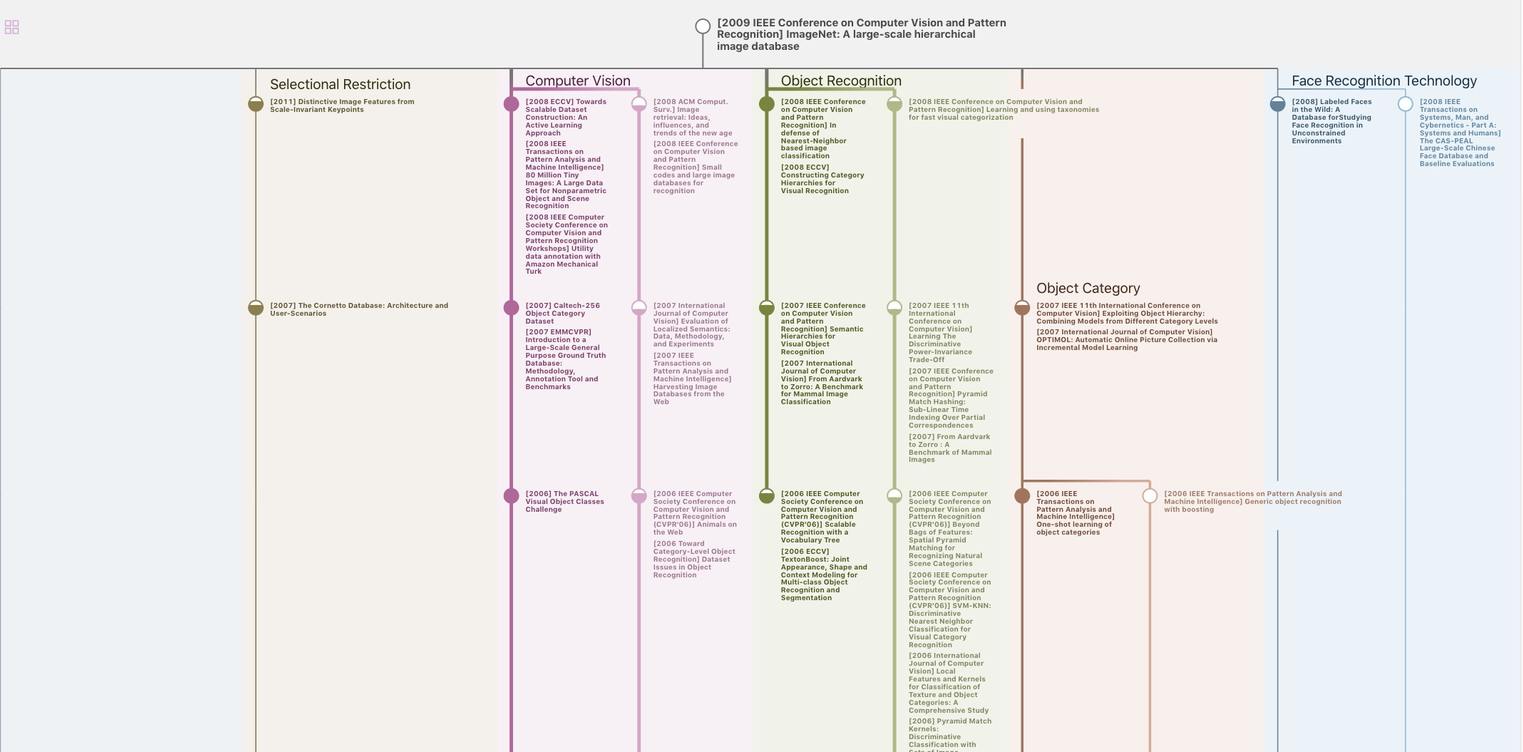
生成溯源树,研究论文发展脉络
Chat Paper
正在生成论文摘要