Compressing unstructured mesh data from simulations using machine learning
International Journal of Data Science and Analytics(2019)
摘要
The amount of data output from a computer simulation has grown to terabytes and petabytes as increasingly complex simulations are being run on massively parallel systems. As we approach exaflop computing in the next decade, it is expected that the I/O subsystem will not be able to write out these large volumes of data. In this paper, we explore the use of machine learning to compress the data before it is written out. Despite the computational constraints that limit us to using very simple learning algorithms, our results show that machine learning is a viable option for compressing unstructured data. We demonstrate that by simply using a better sampling algorithm to generate the training set, we can obtain more accurate results compared to random sampling, but at no extra cost. Further, by carefully selecting and incorporating points with high prediction error, we can improve reconstruction accuracy without sacrificing the compression rate.
更多查看译文
关键词
Regression,Compression,Computer simulations,Mesh data
AI 理解论文
溯源树
样例
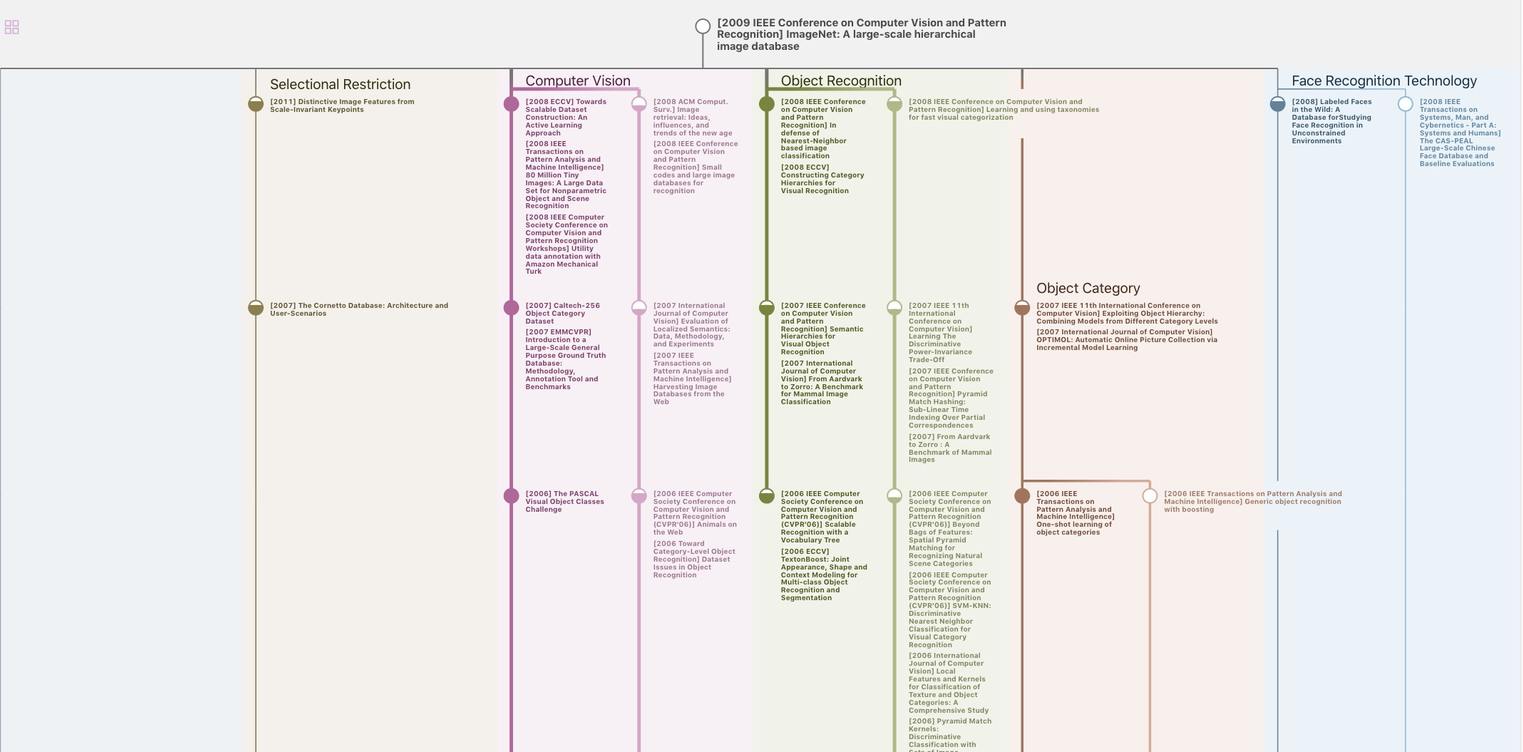
生成溯源树,研究论文发展脉络
Chat Paper
正在生成论文摘要