Pulmonary Nodule Detection in CT Images Using Optimal Multilevel Thresholds and Rule-based Filtering
IETE JOURNAL OF RESEARCH(2022)
摘要
Lung cancer is the most common cancer causing the highest mortality rate as per the analysis of previous and recent year worldwide statistics. The main reason behind the high mortality is late detection of its fatal stage. Early detection of lung nodules offers challenging tasks due to nodules' types, size, unfavorable locations, and attachment with the vessels. In this paper, we propose a method of early detection of lung nodules in computed tomography images, which can assist radiologists to locate the suspected nodules. The method consists of three major sections, i.e. the extraction and segmentation of lung parenchyma through clustering algorithm, extraction and highlighting the region of interests through optimal multilevel thresholding using particle swarm optimization, selection and application of 2D features to detect the initial nodule candidates and further iteratively false positive reduction by the analysis of 3D features. We used 84 CT scans with 301 nodules taken from publically available Lung Image Database Consortium - Image Database Resource Initiative (LIDC-IDRI) data set for experiment. In general for all types of nodules, the proposed method achieved an overall sensitivity of 84.05% with a reduced false positive rate of 1.93 per CT scan. Our results have been compared with the methods from literature which show improved false positive rate along with comparable sensitivity.
更多查看译文
关键词
Computer-aided-detection (CADe) system, Computed tomography (CT) images, Lung cancer, Nodule detection, Particle swarm optimization (PSO), Pulmonary nodule, Region of interest (ROI)
AI 理解论文
溯源树
样例
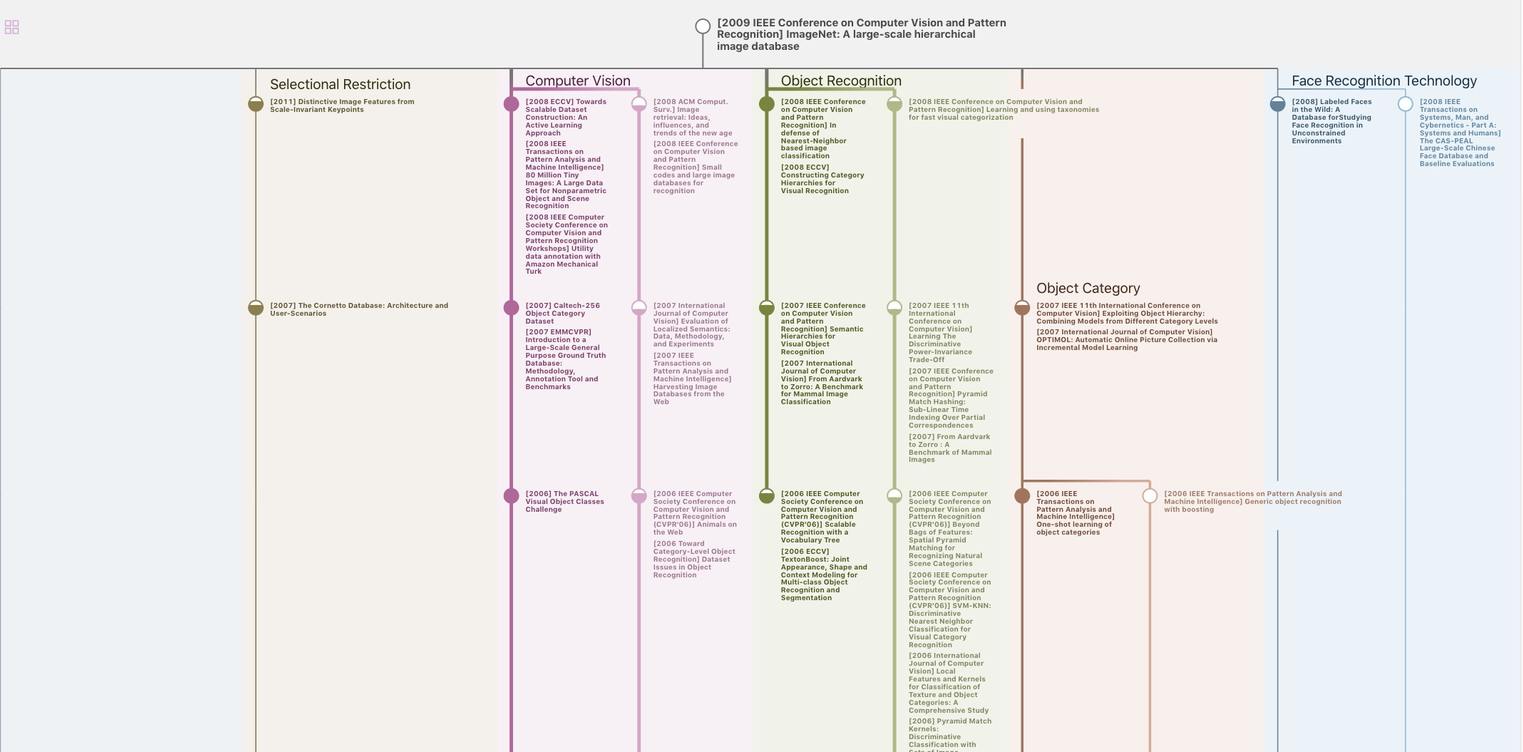
生成溯源树,研究论文发展脉络
Chat Paper
正在生成论文摘要