Domain Adversarial Training For Improving Keyword Spotting Performance Of Esl Speech
2019 IEEE INTERNATIONAL CONFERENCE ON ACOUSTICS, SPEECH AND SIGNAL PROCESSING (ICASSP)(2019)
摘要
A second language (L2) learner usually cannot speak L2 well in both pronunciations and forming-of-words. Hence his/her L2 speech cannot be well recognized by a recognizer trained with native data. Domain adversarial training (DAT), capable of reducing the acoustic mismatch between training and testing, can be useful for improving speech recognition of L2 learners. To get around the ungrammatical L2 speech in scenario-based conversation training, keyword spotting (KWS) is an effective solution by relaxing the language model constraint in decoding. On the acoustic pronunciation side, DAT is investigated in this study for training a neural net-based acoustic model. DAT model is trained with both native and English as second language (ESL) learners' speech to extract more invariant features from native to ESL speech by equalizing their intrinsic difference. The model is jointly optimized for improved senone classification in training. Testing on ESL learners' speech and native English, the DAT model improves recognition performance which is comparable to jointly trained multi-condition model but significantly improves the performance of native speech recognition. In KWS, DAT shows a consistent better performance than the multi-condition training. The improved performance of proposed model is also obtained without increasing its computation complexity or the model size.
更多查看译文
关键词
Domain adversarial training, CALL, ESL, ASR, Keyword spotting
AI 理解论文
溯源树
样例
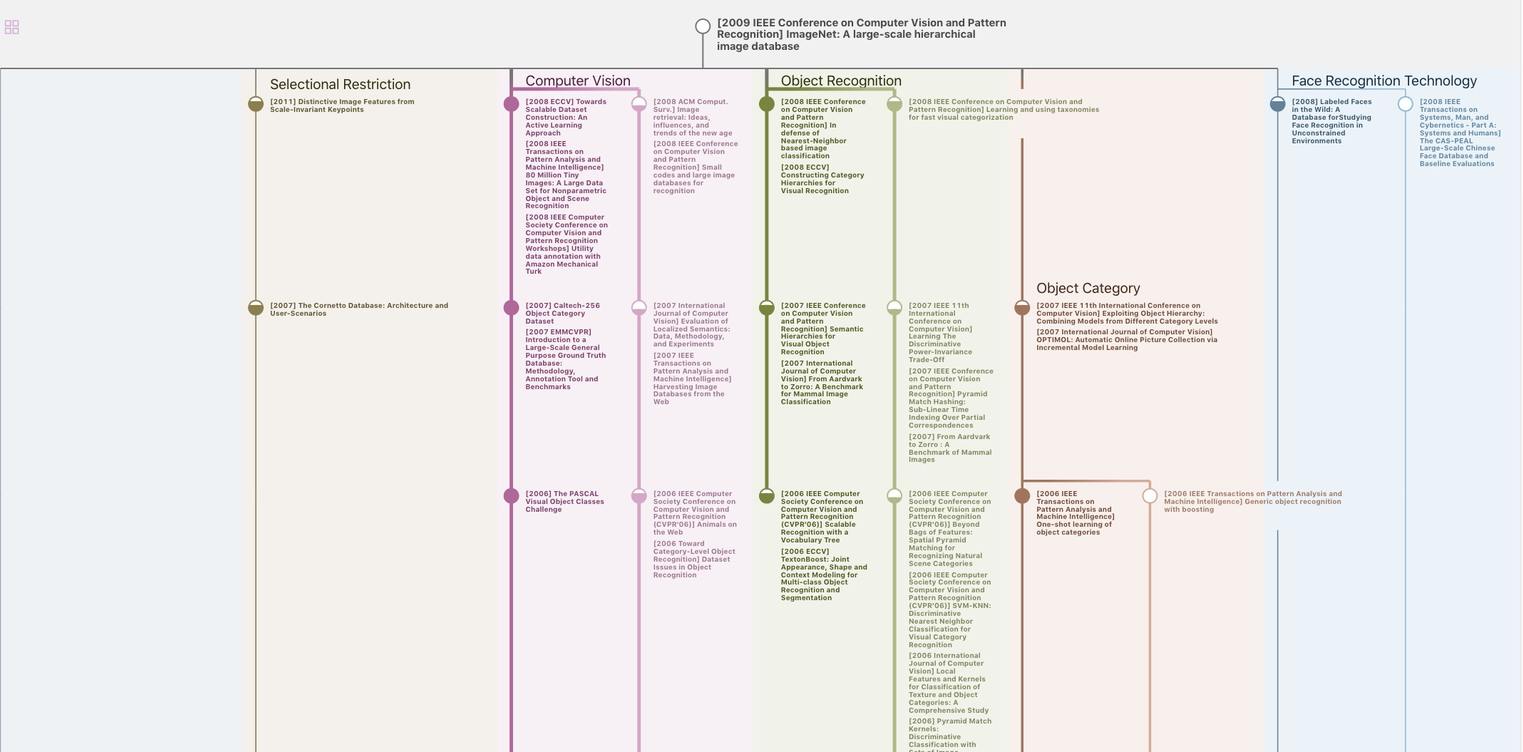
生成溯源树,研究论文发展脉络
Chat Paper
正在生成论文摘要