Decoupling Category-Wise Independence And Relevance With Self-Attention For Multi-Label Image Classification
2019 IEEE INTERNATIONAL CONFERENCE ON ACOUSTICS, SPEECH AND SIGNAL PROCESSING (ICASSP)(2019)
摘要
Multi-label image classification has achieved remarkable progress thanks to deep convolutional neural networks (CNNs). In this paper, we propose a Decouple Network (DecoupleNet) which is an end-to-end CNN-based framework able to trade off class-level feature independence and relevance during training. The proposed DecoupleNet is able to decouple category-wise independence and relevance with image-level supervision. We design a category-wise space-to-depth module with a spatial pooling strategy to exploit more meaningful convolutional features. They are integrated with class-wise correlated information which is automatically learned via a new self-attention mechanism. We conduct extensive experiments on two large-scale benchmarks: the MS-COCO and the NUS-WIDE, where the proposed DecoupleNet obtains impressive performance compared favorably against the state-of-the-art methods on multi-label image classification.
更多查看译文
关键词
Multi-label image classification, self-attention, convolutional neural network
AI 理解论文
溯源树
样例
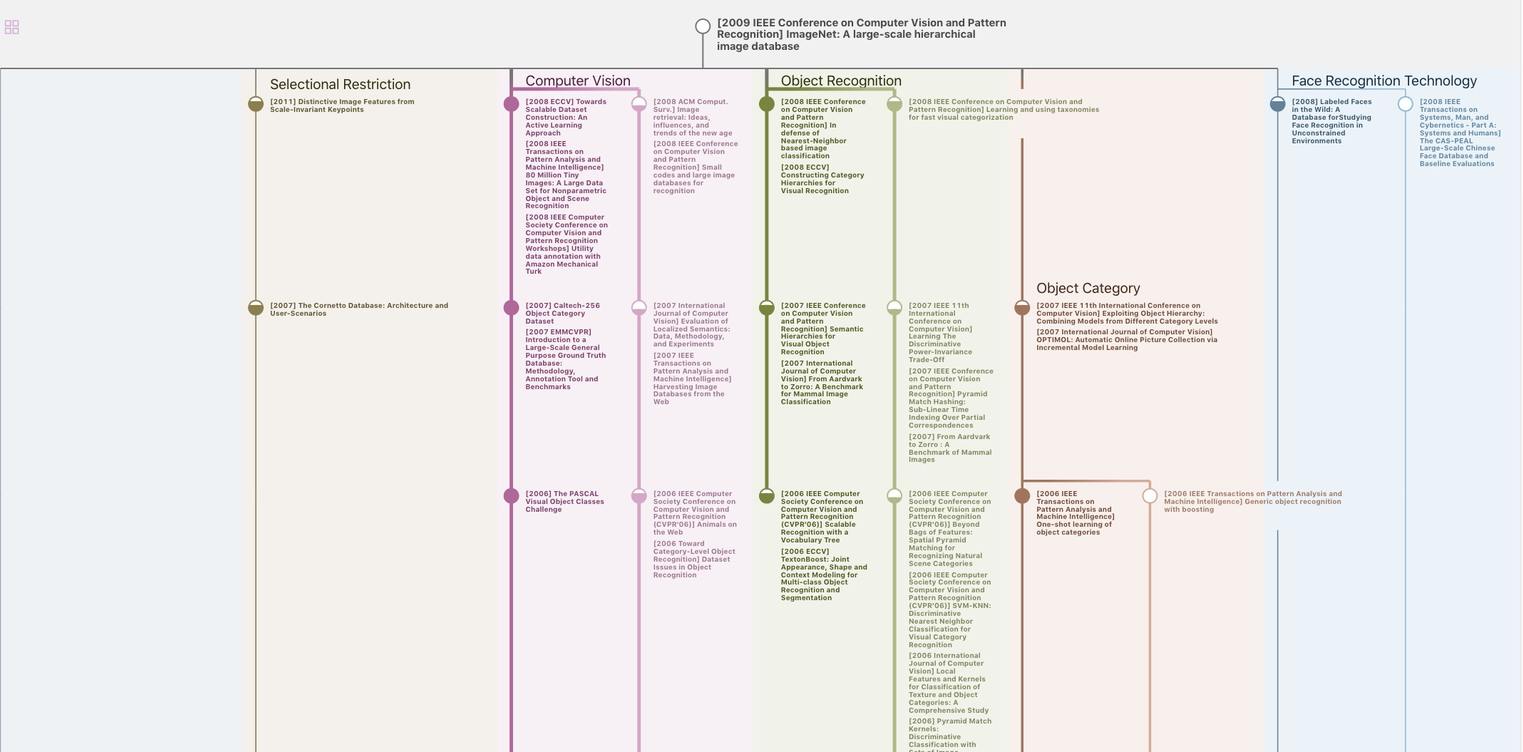
生成溯源树,研究论文发展脉络
Chat Paper
正在生成论文摘要