An Approximated Proximal Alternating Method for Non-convex and Non-smooth Optimization
arXiv: Computer Vision and Pattern Recognition(2017)
摘要
In this paper, an approximated proximal alternating method (APAM) is proposed for solving non-convex and non-smooth problems. Our APAM approximately solves the subproblems to certain inexactness so that it requires neither computing/estimating the Lipschitz constants nor calculating exact solutions. With a special designed error condition, APAM is proved to globally converge to a critical point of the problem, which as far as we know, is so far the best convergence result in non-convex optimization. Moreover, two practical strategies for checking the inexactness criterion are theoretically analyzed, which are more valid than the commonly used criteria in practice. Our algorithm APAM is applied to non-convex dictionary learning problem on both synthetic and real-world data. The experimental results with detailed analyses and discussions are given to help verify the efficiency of APAM.
更多查看译文
AI 理解论文
溯源树
样例
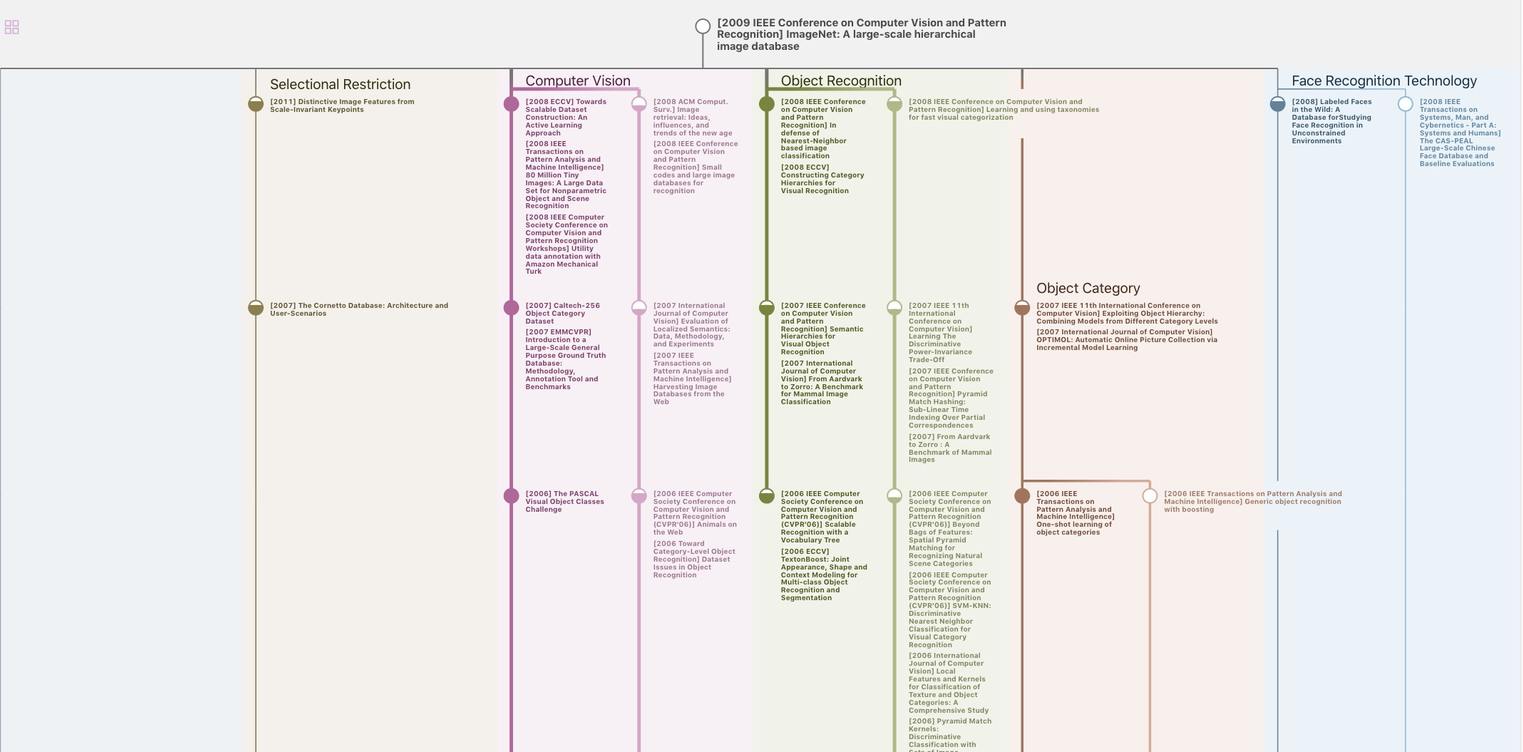
生成溯源树,研究论文发展脉络
Chat Paper
正在生成论文摘要