Error Bounds For Spectral Clustering Over Samples From Spherical Gaussian Mixture Models
2019 IEEE INTERNATIONAL CONFERENCE ON ACOUSTICS, SPEECH AND SIGNAL PROCESSING (ICASSP)(2019)
摘要
Spectral clustering has been one of the most popular methods for clustering multivariate data and has been widely used in image processing and data mining. Despite its considerable empirical success, the theoretical properties of spectral clustering are not yet fully developed. In this paper, we derive upper bounds for the clustering error of spectral clustering for data samples generated from spherical Gaussian mixture models. In our analysis, first, the graph Laplacian calculated from samples is approximated by a reference graph Laplacian which has good spectral properties. Second, we use the Davis-Kahan perturbation theorem to provide an upper bound for the sum of squared distances between each projected data point and its cluster center. Finally, we leverage theoretical results of Meila's to prove an upper bound for the clustering error from the upper bound for the sum of squared distances.
更多查看译文
关键词
Spectral clustering, Gaussian Mixture Models, Optimal clusterings, Error bounds
AI 理解论文
溯源树
样例
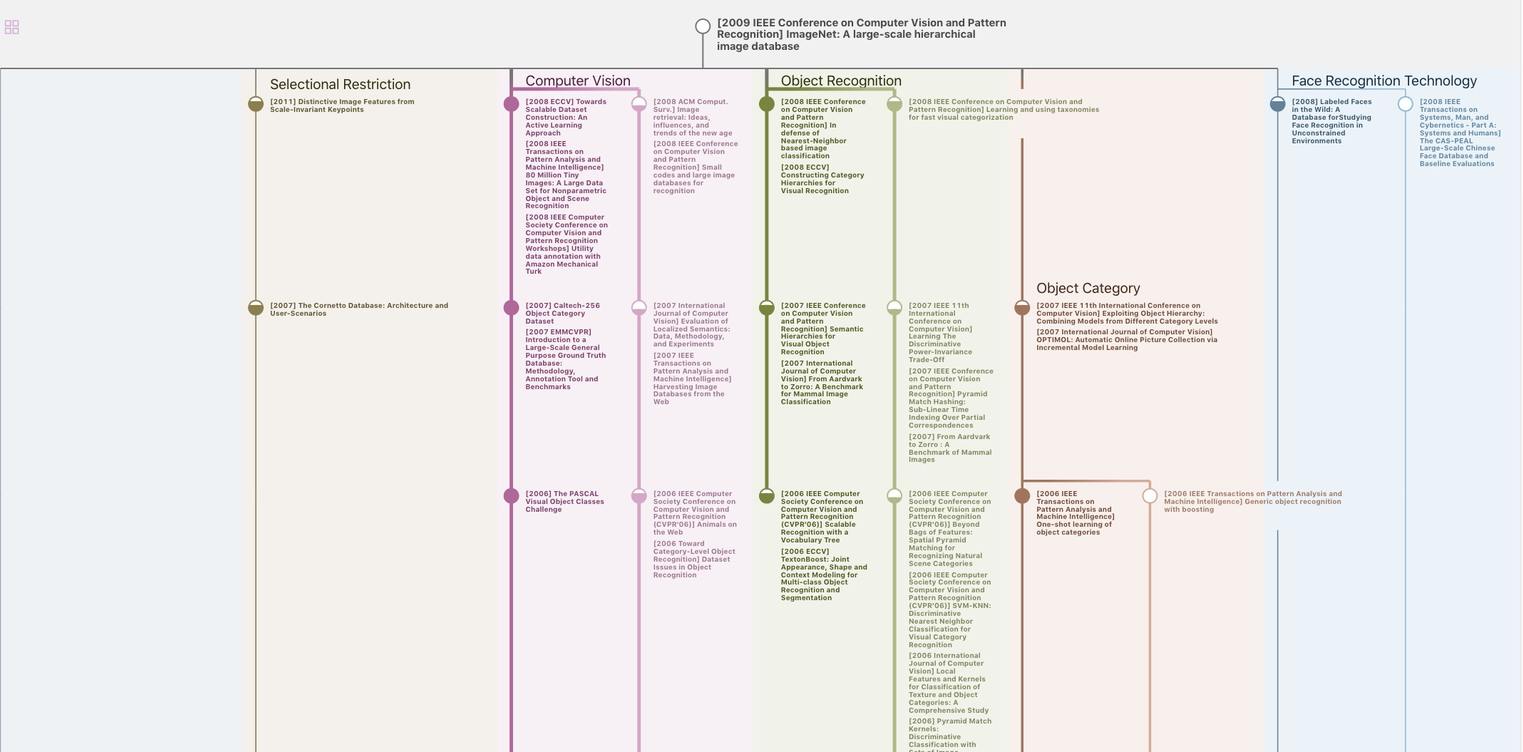
生成溯源树,研究论文发展脉络
Chat Paper
正在生成论文摘要