A Shallow Neural Network Based Short Text Classifier For Medical Community Question Answering System
2018 IEEE 8TH ANNUAL INTERNATIONAL CONFERENCE ON CYBER TECHNOLOGY IN AUTOMATION, CONTROL, AND INTELLIGENT SYSTEMS (IEEE-CYBER)(2018)
摘要
The medical community question answering system (MCQA) which is a novel medical communicating platform is becoming popular due to the large amount of people seeking for solution of health problem. Not all medical questions would get timely answers. Similar question recommendation is a common approach to solve this problem. While the large amount of Q&A pairs in MCQA, classifying for a new problem effectively reduces the size of candidate problem set and improve the efficiency of retrieval for its similar problems. In this paper we proposed a shallow, CNN classification model (SCCM) based on characters which not only narrowing the text feature vector dimensions, but also solving spelling mistakes in text. We crawls and constructs a real Chinese medical Q&A dataset and conducts experimental verification. The results of experiments show that our SCCM can automatically extract the key features in the text and improve the accuracy of classification.
更多查看译文
关键词
medical community question answering system,MCQA,health problem,shallow CNN classification model,text feature vector dimensions,question recommendation,shallow neural network based short text classifier,candidate problem set,Q&A pairs
AI 理解论文
溯源树
样例
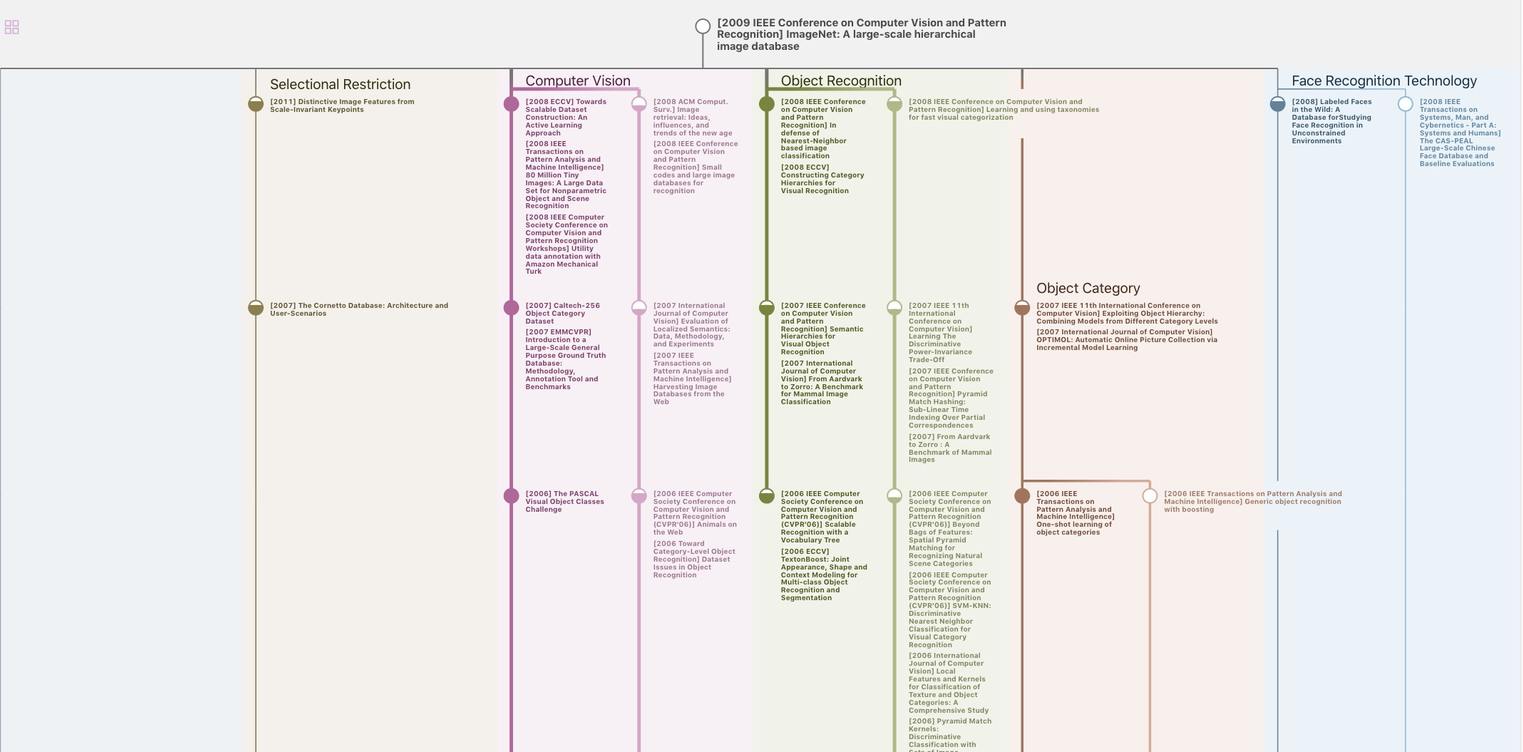
生成溯源树,研究论文发展脉络
Chat Paper
正在生成论文摘要