Layout-Aware Subfigure Decomposition For Complex Figures In The Biomedical Literature
2019 IEEE INTERNATIONAL CONFERENCE ON ACOUSTICS, SPEECH AND SIGNAL PROCESSING (ICASSP)(2019)
摘要
Published scientific figure is a valuable information resource, but often occur as composite images. The ImageCLEF meeting presented a shared evaluation in 2016 to use machine learning to split these composite figures into components automatically. We adapted an existing high-performance object detection method to analyze the substructure of published biomedical figures by developing a novel multi-branch output convolution neural network to predict irregular panel layouts and provide augmented training data to drive learning. Our system has an accuracy of 86.8% on the 2016 ImageCLEF Medical dataset and 83.1% on a new dataset derived from open access papers from the INTACT database of molecular interactions.
更多查看译文
关键词
subfigure decomposition, convolutional neural network, biomedical data, compound figures
AI 理解论文
溯源树
样例
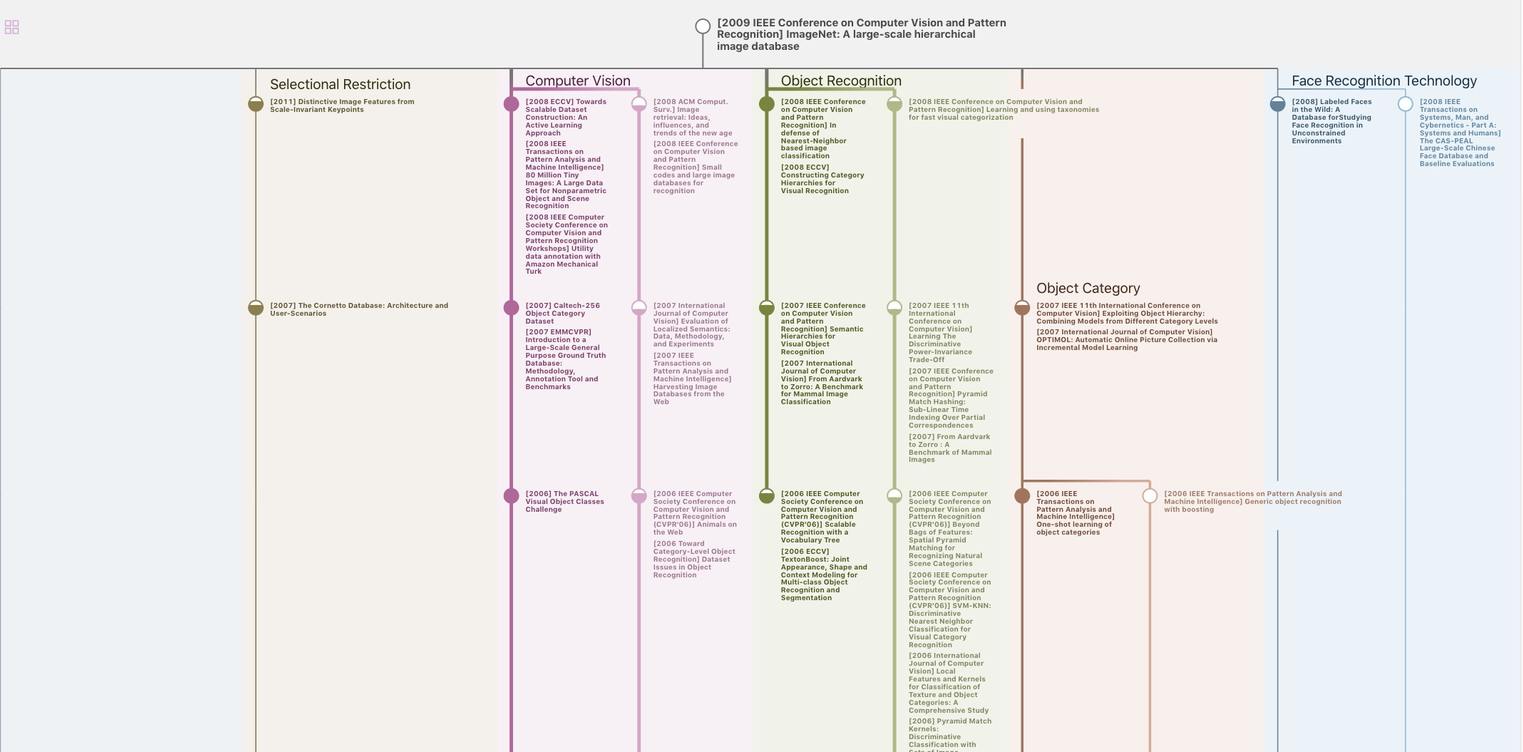
生成溯源树,研究论文发展脉络
Chat Paper
正在生成论文摘要