Adaptation Of Multiple Sound Source Localization Neural Networks With Weak Supervision And Domain-Adversarial Training
2019 IEEE INTERNATIONAL CONFERENCE ON ACOUSTICS, SPEECH AND SIGNAL PROCESSING (ICASSP)(2019)
摘要
Despite the recent success of deep neural network-based approaches in sound source localization, these approaches suffer the limitations that the required annotation process is costly, and the mismatch between the training and test conditions undermines the performance. This paper addresses the question of how models trained with simulation can be exploited for multiple sound source localization in real scenarios by domain adaptation. In particular, two domain adaptation methods are investigated: weak supervision and domain-adversarial training. Our experiments show that the weak supervision with the knowledge of the number of sources can significantly improve the performance of an unadapted model. However, the domain-adversarial training does not yield significant improvement for this particular problem.
更多查看译文
关键词
Sound source localization, DOA estimation, domain adaptation, weakly-supervised learning
AI 理解论文
溯源树
样例
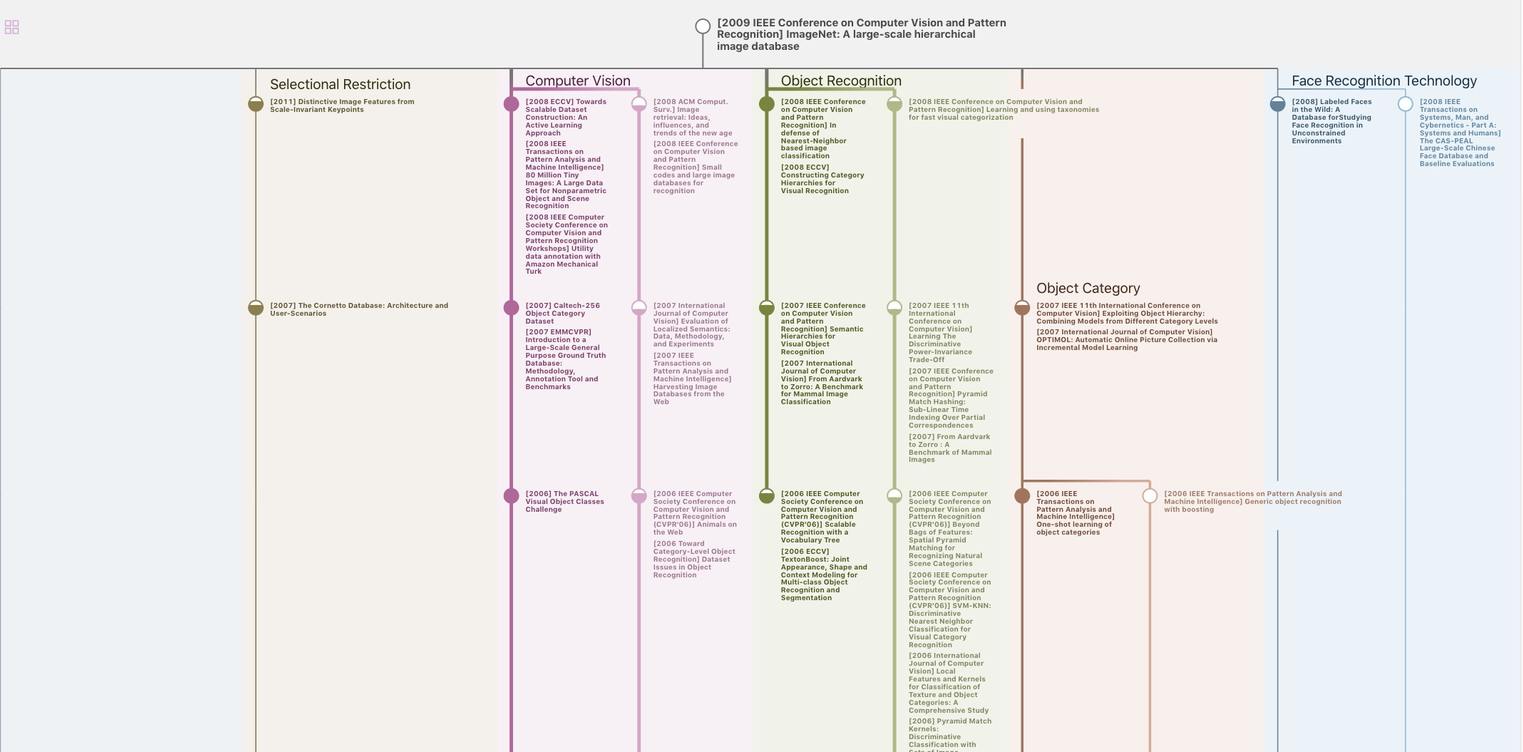
生成溯源树,研究论文发展脉络
Chat Paper
正在生成论文摘要