Learning Jumping Skills From Human With A Fast Reinforcement Learning Framework
2018 IEEE 8TH ANNUAL INTERNATIONAL CONFERENCE ON CYBER TECHNOLOGY IN AUTOMATION, CONTROL, AND INTELLIGENT SYSTEMS (IEEE-CYBER)(2018)
摘要
Dynamic policies of locomotion control, which is one of the key problems for legged robots, remains great challenges, especially for newly arising bionic robots. In this paper, we propose a framework based on reinforcement learning to learn jumping skills from human demonstrations for a single legged robot. This approach adopts guided policy search (GPS) algorithms to apply trajectory optimization to direct strategy learning avoiding falling into the local optimum and uses differential dynamic programming to generate suitable bootstrap samples with the single legged hopping robot. The parameterized joint trajectories are learned from human demonstrations, and optimal control policies are established efficiently in an up to 8-dimensional parameter space, therefore the legged robot is controlled to jump as graceful as human beings do. The proposed algorithm is tested in our physical simulation based single leg platform which includes a completely rigid robot without any compliance mechanisms. Experiments demonstrate this approach works efficiently with relative small samples and the robot can learn to jump pretty well within only a few iterations.
更多查看译文
关键词
RL, GPS, robot, physical simulation
AI 理解论文
溯源树
样例
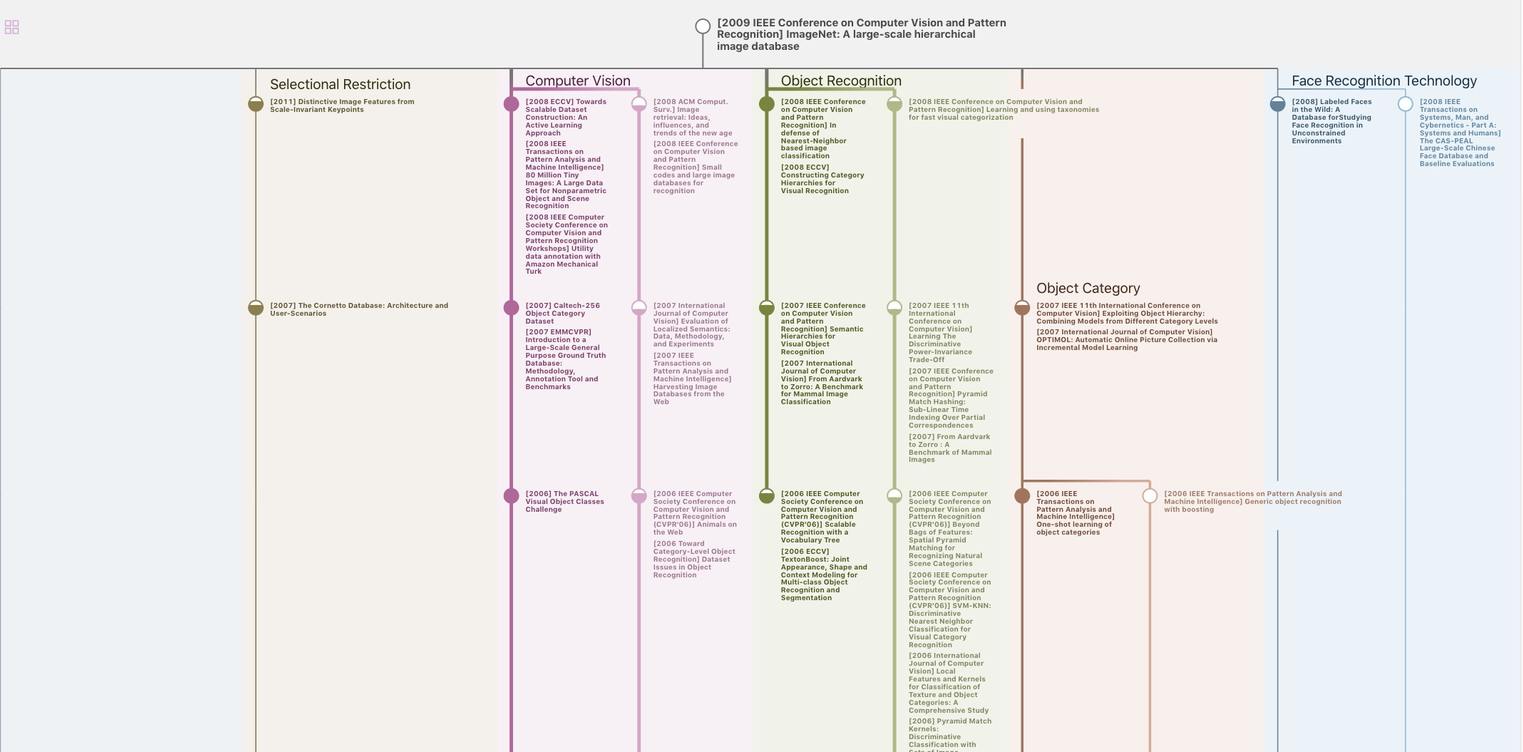
生成溯源树,研究论文发展脉络
Chat Paper
正在生成论文摘要