Modeling the Language of Life – Deep Learning Protein Sequences
bioRxiv(2019)
摘要
One common task in Computational Biology is the prediction of aspects of protein function and structure from their amino acid sequence. For 26 years, most state-of-the-art approaches toward this end have been marrying machine learning and evolutionary information resulting from related proteins retrieved at increasing cost from ever growing sequence databases. This search is often so time-consuming to prevent analyzing entire proteomes. On top, evolutionary information is less powerful for smaller families, e.g. for proteins from the Dark Proteome. Here, we introduced a novel way to represent protein sequences as continuous vectors (embeddings) by utilizing the deep bi-directional language model ELMo that effectively captured the biophysical properties of protein sequences from unlabeled big data (UniRef50). After training, this knowledge was transferred for single protein sequences along with other relevant sequence features. We referred to these new embeddings as SeqVec and demonstrated their effectiveness by training comparably simple neural networks on existing data sets for two completely different prediction tasks. For the per-residue level, we predicted secondary structure (for NetSurfP-2.0 data set: Q3=79%±1, Q8=68%±1) and disorder (MCC=0.59±0.03). For the per-protein level, we predicted subcellular localization in ten classes (for DeepLoc dataset: Q10=68%±1) and distinguished membrane-bound from water-soluble proteins (Q2= 87%±1). All results built upon the new tool SeqVec derived from single protein sequences. Where the lightning-fast HHblits needed on average 0.5 - 5 minutes to generate the evolutionary information for a single protein, our SeqVec created the vector representation on average in 0.027 seconds.
更多查看译文
关键词
Machine Learning,Language Modeling,Sequence Embedding,Secondary structure prediction,Localization prediction,Transfer Learning,Deep Learning
AI 理解论文
溯源树
样例
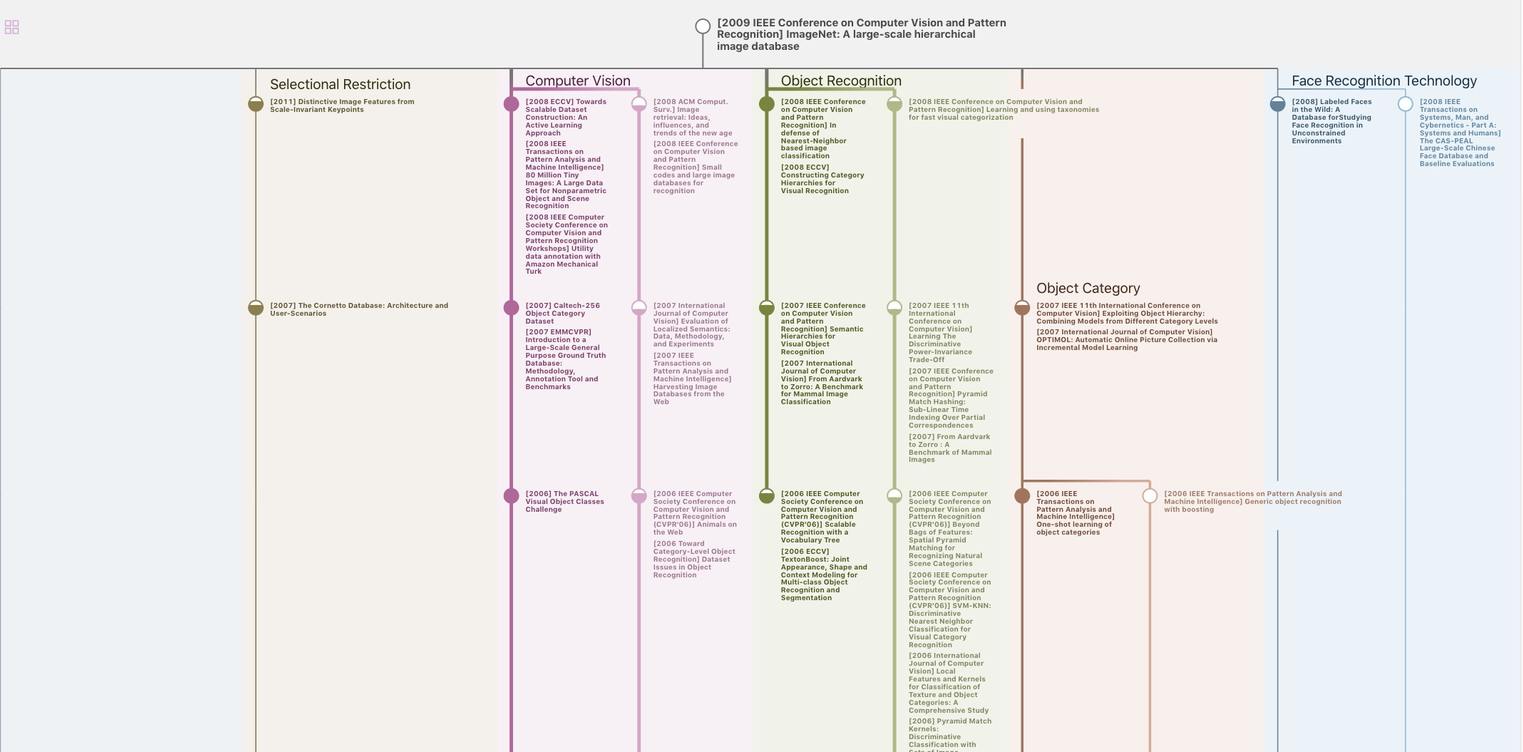
生成溯源树,研究论文发展脉络
Chat Paper
正在生成论文摘要