Information Theoretic Lower Bound Of Restricted Isometry Property Constant
2019 IEEE INTERNATIONAL CONFERENCE ON ACOUSTICS, SPEECH AND SIGNAL PROCESSING (ICASSP)(2019)
摘要
Compressed sensing seeks to recover an unknown sparse vector from undersampled rate measurements. Since its introduction, there have been enormous works on compressed sensing that develop efficient algorithms for sparse signal recovery. The restricted isometry property (RIP) has become the dominant tool used for the analysis of exact reconstruction from seemingly undersampled measurements. Although the upper bound of the RIP constant has been studied extensively, as far as we know, the result is missing for the lower bound. In this work, we first present a tight lower bound for the RIP constant, filling the gap there. The lower bound is at the same order as the upper bound for the RIP constant. Moreover, we also show that our lower bound is close to the upper bound by numerical simulations. Our bound on the RIP constant provides an information-theoretic lower bound about the sampling rate for the first time, which is the essential question for practitioners.
更多查看译文
关键词
Compressed sensing, sparse recovery, restricted isometry property, Gaussian random matrix, upper and lower bound
AI 理解论文
溯源树
样例
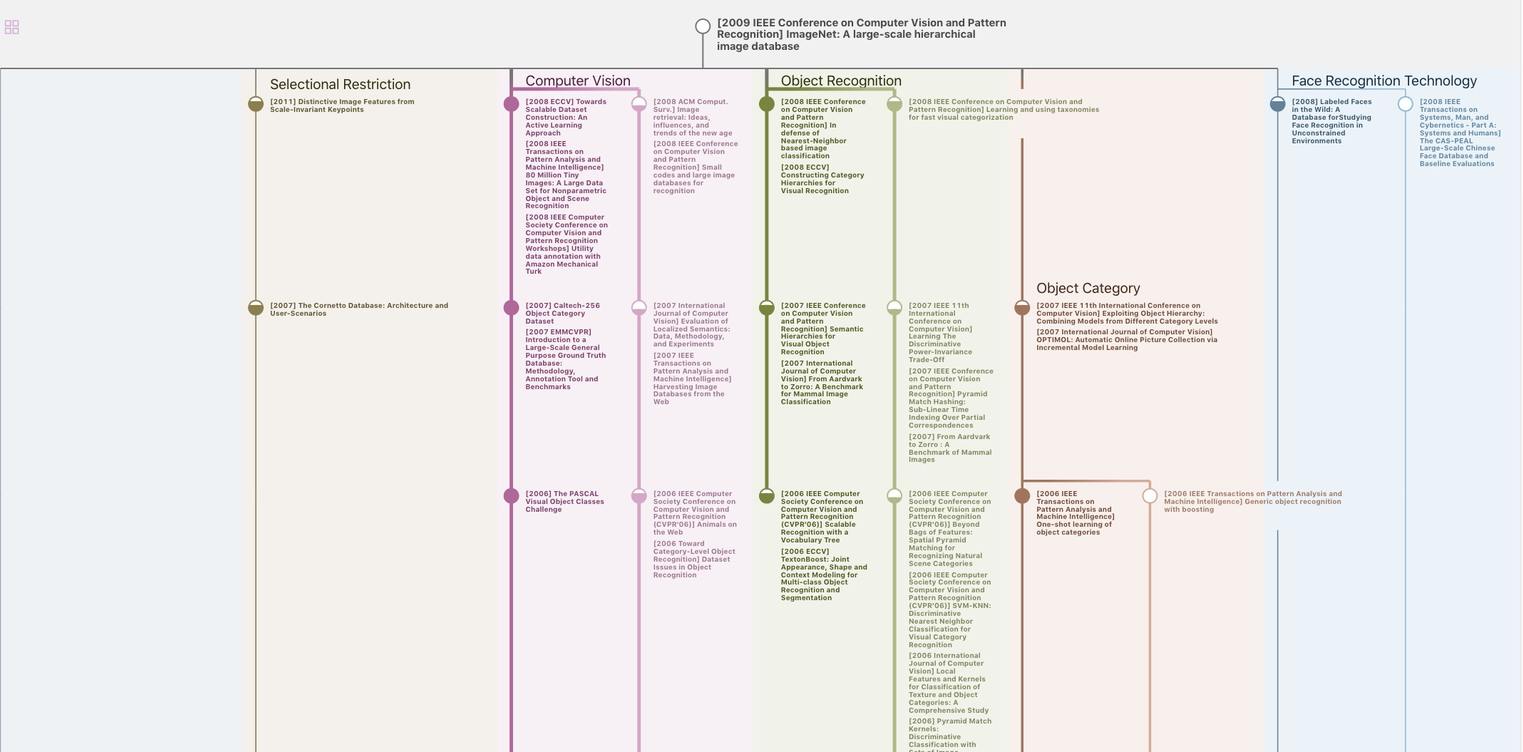
生成溯源树,研究论文发展脉络
Chat Paper
正在生成论文摘要