Comparison Of Data Augmentation And Adaptation Strategies For Code-Switched Automatic Speech Recognition
2019 IEEE INTERNATIONAL CONFERENCE ON ACOUSTICS, SPEECH AND SIGNAL PROCESSING (ICASSP)(2019)
摘要
Code-switching occurs when the speaker alternates between two or more languages or dialects. It is a pervasive phenomenon in most Indic spoken languages. Code-switching poses a challenge in language modeling as it complicates the orthographic realization of text, and generally, there is a shortage of code-switched data. In this paper, we investigate data augmentation and adaptation strategies for language modeling. Using Bengali and English as an example, we study augmenting the code-switched transcripts with separate transliterated Bengali and English corpora. We present results on two speech recognition tasks, namely, voice search and dictation. We show improvements on both tasks with Maximum Entropy ( MaxEnt) and Long Short-Term Memory ( LSTM) language models ( LMs). We also explore different adaptation strategies for MaxEnt LM and LSTM LM, demonstrating that the transliteration-based data-augmented LSTM LM matches the adapted MaxEnt LM which is trained on more Bengali-English data.
更多查看译文
关键词
data augmentation, language model adaptation, code-switched automatic speech recognition
AI 理解论文
溯源树
样例
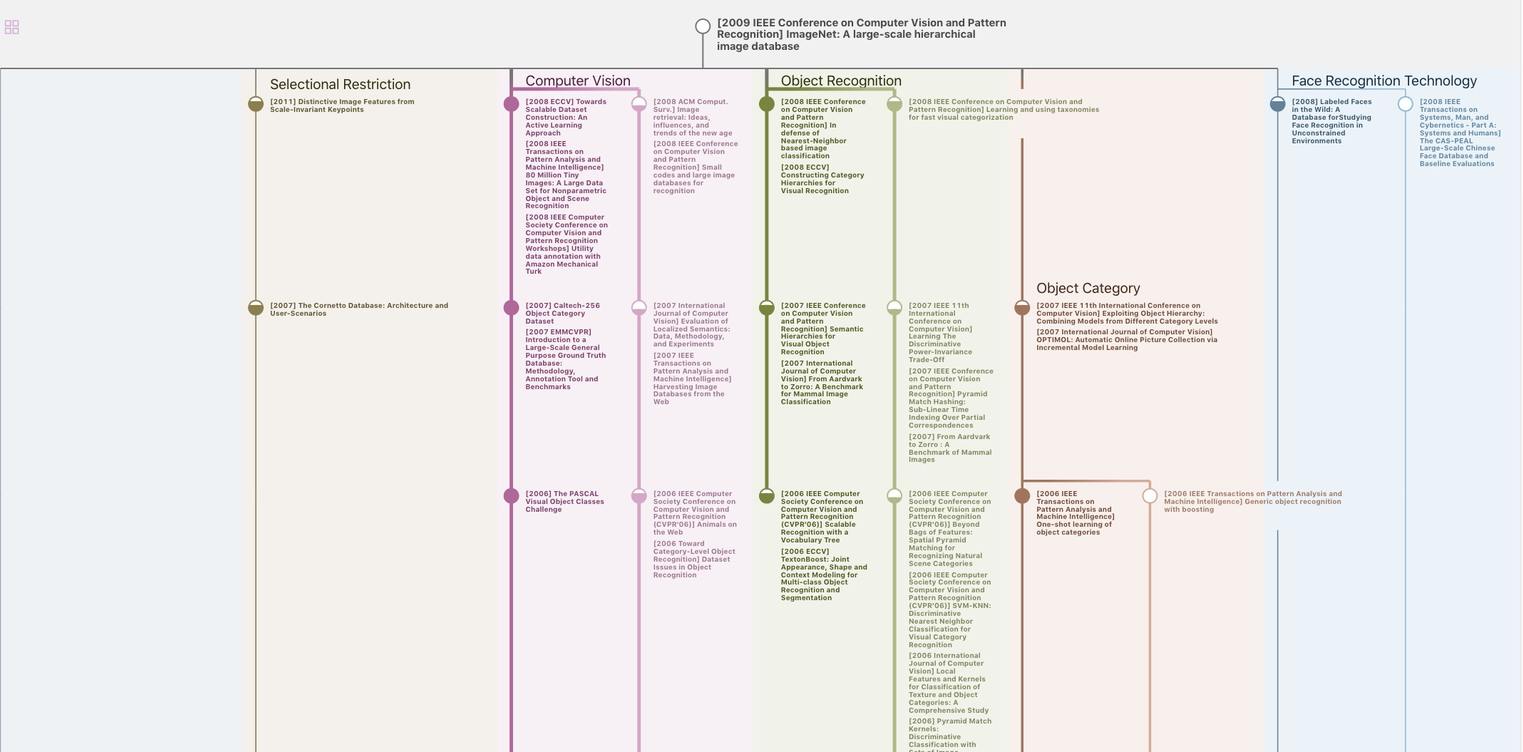
生成溯源树,研究论文发展脉络
Chat Paper
正在生成论文摘要