A novel clustering algorithm based on the natural reverse nearest neighbor structure
Information Systems(2019)
摘要
Cluster analysis plays an important role in identifying the natural structure of the target dataset. It has been widely used in many fields, such as pattern recognition, machine learning, image segmentation, document clustering and so on. There are many different methods to conduct cluster analysis. Namely, most real datasets are non-spherical and have complex shapes. Although these methods are widely used to deal with clustering tasks, they are susceptible to noise and arbitrary shapes. Thus, we propose a novel clustering algorithm (called RNN-NSDC) in this paper, which is based on the natural reverse nearest neighbor structure. Firstly, we apply the reverse nearest neighbors in the algorithm to extract core objects. Secondly, our algorithm uses the neighbor structure information of core objects to cluster. And excluding noise effects, core sets can well represent the structure of clusters. Therefore, the RNN-NSDC can obtain the optimal cluster numbers for the datasets which contain clusters of outliers and arbitrary shapes. To verify the efficiency and accuracy of the RNN-NSDC, synthetic datasets and real datasets are used for experiments. The results indicate the superiority of the RNN-NSDC compared with K-means, DBSCAN, DPC, SNNDPC, DCore and NaNLORE.
更多查看译文
关键词
Clustering,Density core,Natural neighbor,Reverse-nearest neighbor
AI 理解论文
溯源树
样例
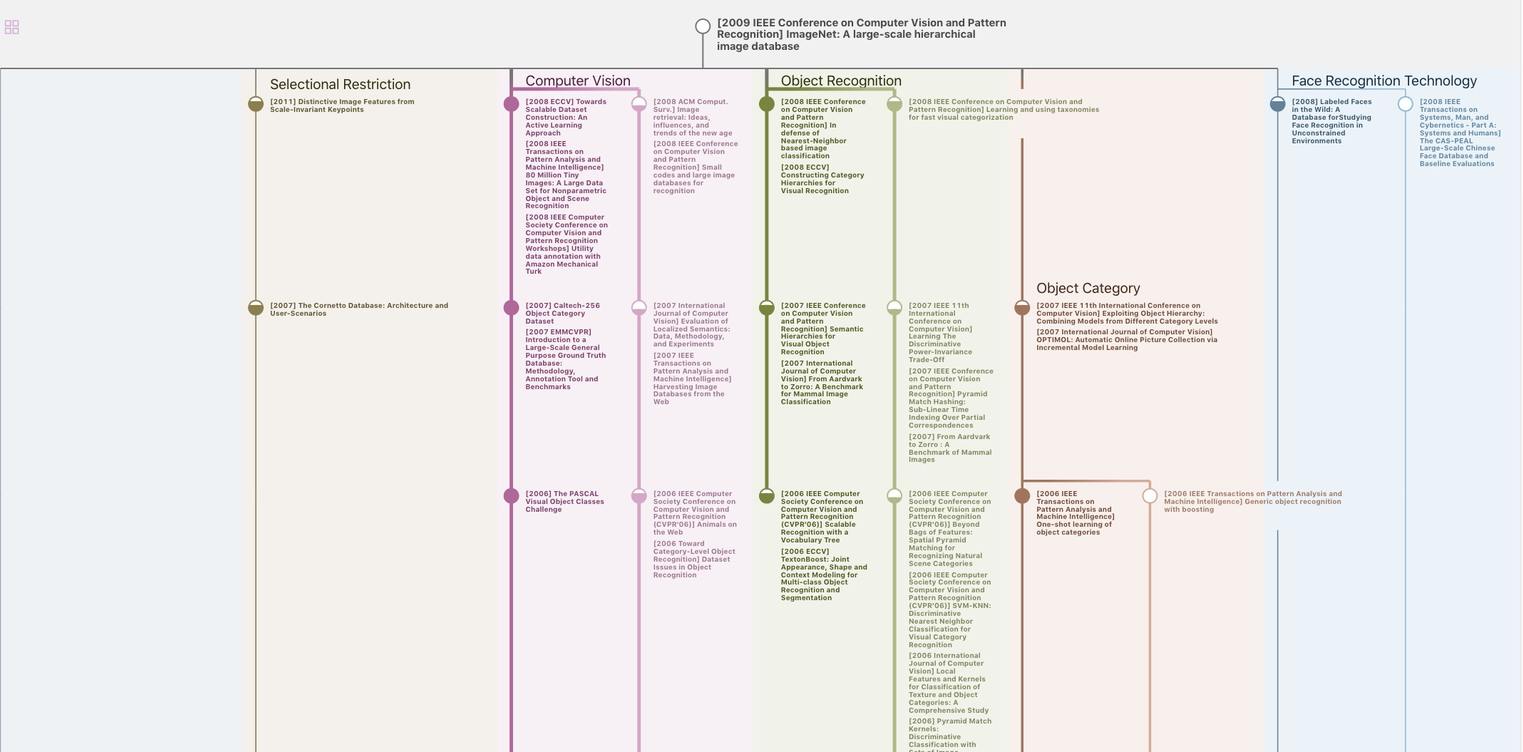
生成溯源树,研究论文发展脉络
Chat Paper
正在生成论文摘要