Using deep learning and hyperspectral imaging to predict total viable count (TVC) in peeled Pacific white shrimp
Journal of Food Measurement and Characterization(2019)
摘要
In this study, deep learning method coupled with near-infrared (NIR) hyperspectral imaging (HSI) technique was used for nondestructively determining total viable count (TVC) of peeled Pacific white shrimp. Firstly, stacked auto-encoders (SAE) was conducted as a big data analytical method to extract 20 deep hyperspectral features from NIR hyperspectral image (900–1700 nm) of peeled shrimp stored at 4 °C, and the extracted features were used to predict TVC by fully-connected neural network (FNN). The SAE–FNN method obtained high prediction accuracy for determining TVC, with R P 2 = 0.927. Additionally, TVC spatial distribution of peeled shrimp during storage could be visualized via applying the established SAE–FNN model. The results demonstrate that SAE–FNN combined with HSI technique has a potential for non-destructive prediction of TVC in peeled shrimp, which supply a novel method for the hygienic quality and safety inspections of shrimp product.
更多查看译文
关键词
Hyperspectral image, Microbial spoilage, Deep learning, Stacked auto-encoders, Fully-connected neural network, Nondestructive detection method
AI 理解论文
溯源树
样例
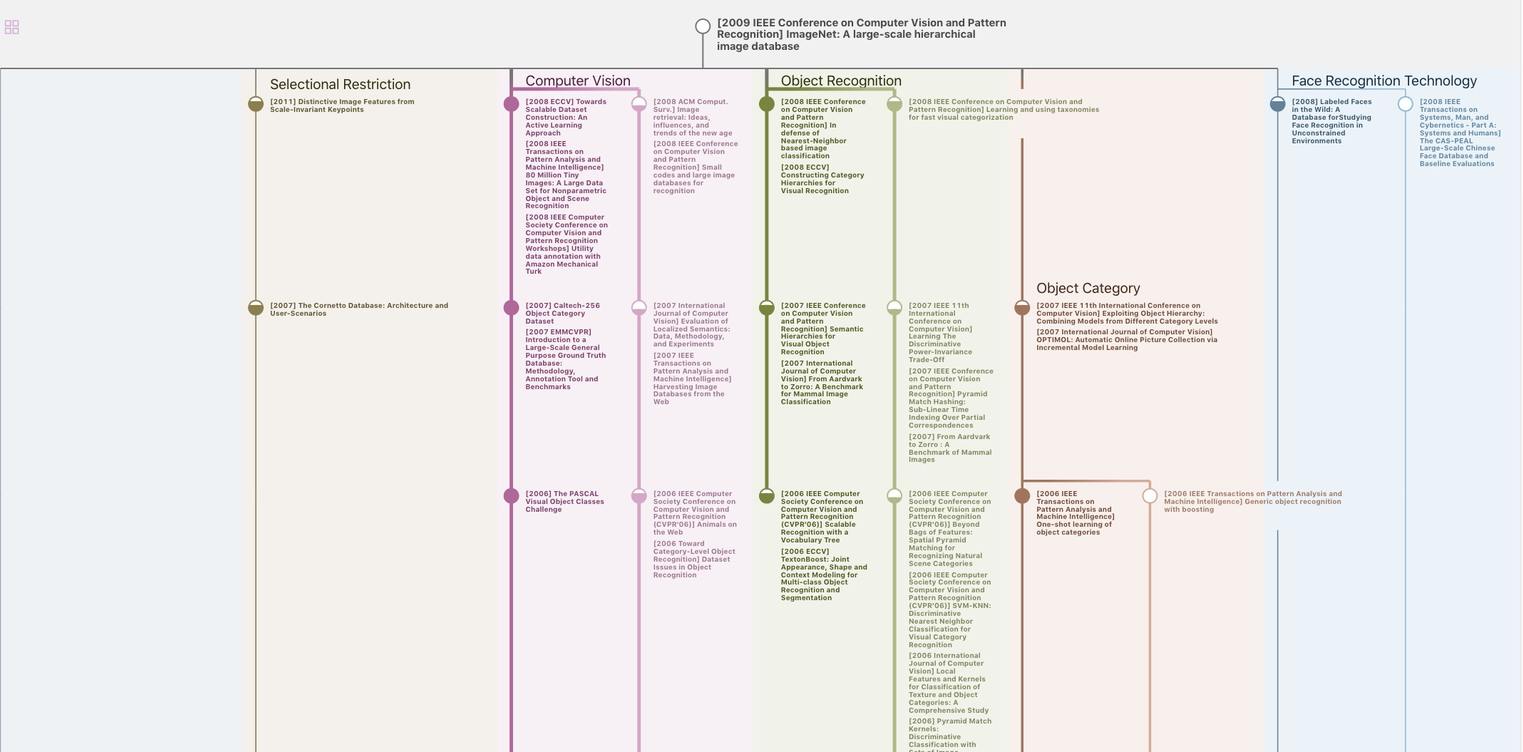
生成溯源树,研究论文发展脉络
Chat Paper
正在生成论文摘要