Low-Rank Estimation Based Evolutionary Clustering For Community Detection In Temporal Networks
2019 IEEE INTERNATIONAL CONFERENCE ON ACOUSTICS, SPEECH AND SIGNAL PROCESSING (ICASSP)(2019)
摘要
Many real-world systems can be represented by networks. One common approach to characterizing the organization of networks is community detection. A lot of work has been conducted in community detection of static networks. However, most real systems are time dependent and modeled by temporal networks with a structure that evolves across time. In this paper, a low-rank approximation based evolutionary clustering approach is introduced to detect and track the community structure of temporal networks. The proposed approach provides robustness to outliers and results in smoothly evolving cluster assignments through joint low-rank approximation and subspace learning. Moreover, a cost function is introduced to track changes in the community structure across time. The performance of the proposed approach is validated on both simulated and real temporal networks.
更多查看译文
关键词
Temporal networks, community detection, evolutionary spectral clustering, low-rank approximation
AI 理解论文
溯源树
样例
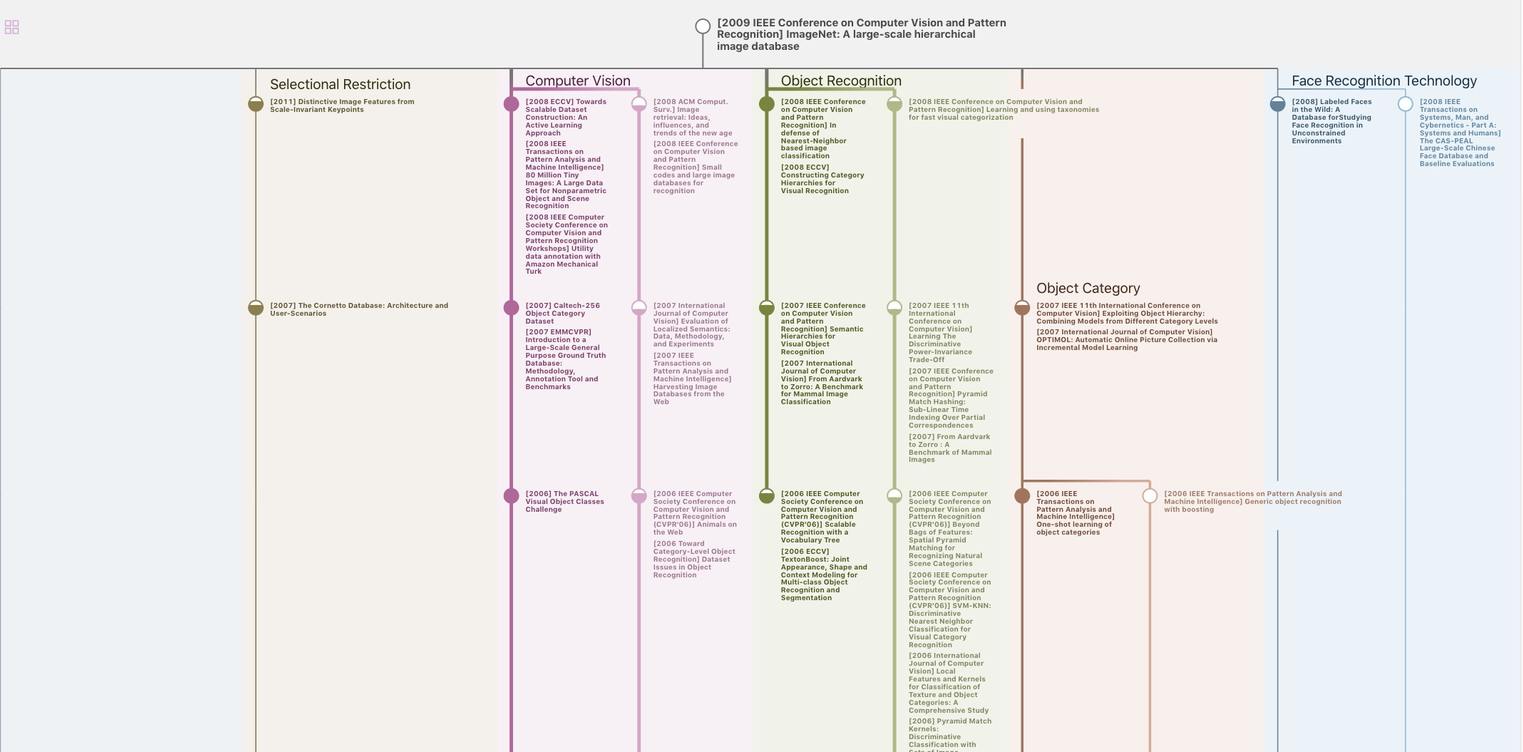
生成溯源树,研究论文发展脉络
Chat Paper
正在生成论文摘要