Anomaly Detection In Single Subject Vs Group Using Manifold Learning
2019 IEEE INTERNATIONAL CONFERENCE ON ACOUSTICS, SPEECH AND SIGNAL PROCESSING (ICASSP)(2019)
摘要
This paper compares several linear and non-linear multivariate models for the detection of abnormal patterns in neuroimaging data, when comparing a single subject to a normal control group. The proposed methods learn the manifold spanned by the normal controls using non-linear dimension reduction techniques. The image of a subject is projected on the control group manifold either via a standard projection or through an embedding/reconstruction scheme. A comparison of the reconstruction with the subject's original neuroimaging data allows for the detection of abnormal patterns by way of statistical tests on the residuals. The different abnormality detection methods are assessed on synthetic data and real (MRI) neuroimaging data. The importance of non-linear modeling of the manifold in the reduced-dimension subspace is highlighted, as well as robustness to large abnormalities.
更多查看译文
关键词
Anomaly detection, subject vs group comparison, manifold learning, PCA, Isomap, LLE
AI 理解论文
溯源树
样例
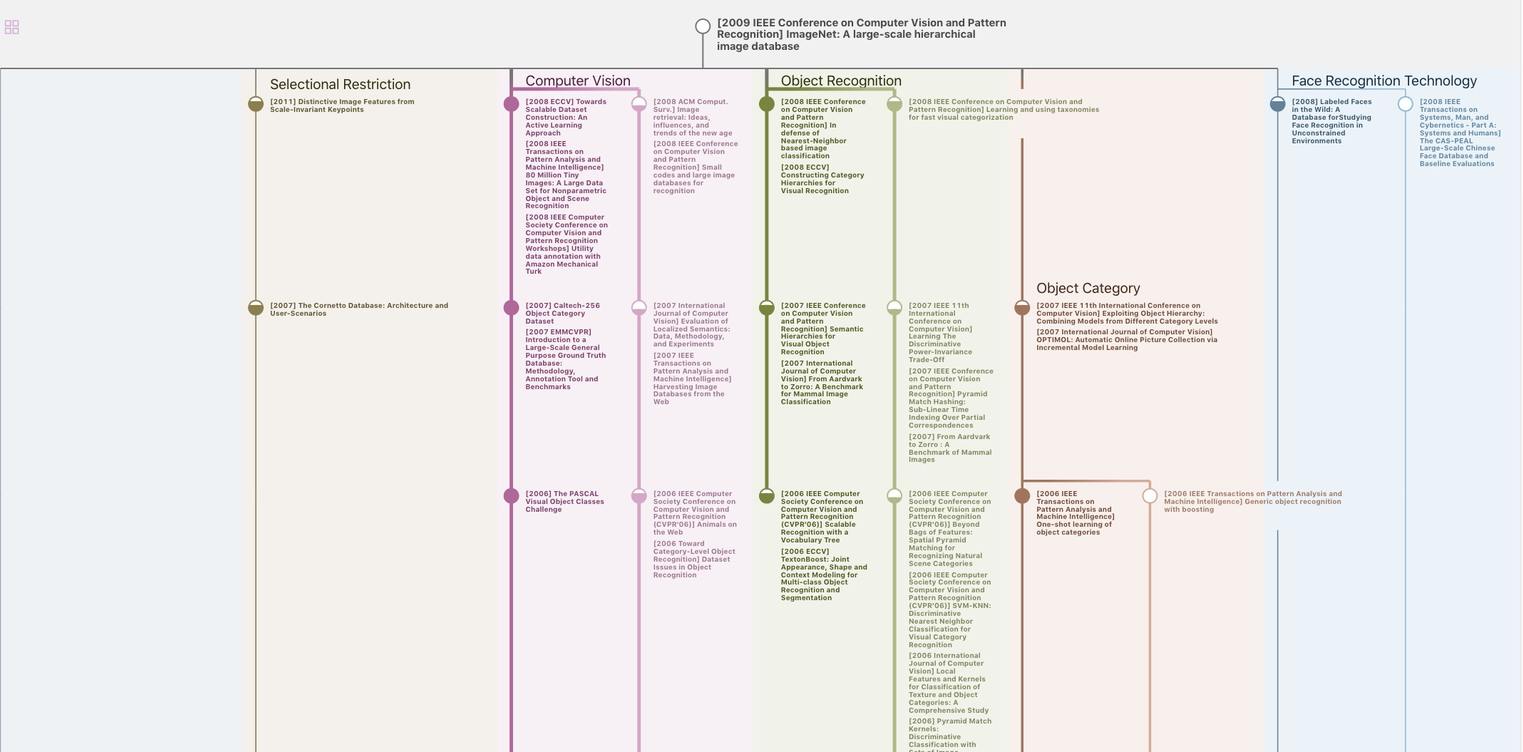
生成溯源树,研究论文发展脉络
Chat Paper
正在生成论文摘要