Bayesian Experimental Design Without Posterior Calculations: an Adversarial Approach
Bayesian analysis(2023)
摘要
Most computational approaches to Bayesian experimental design require making posterior calculations repeatedly for a large number of potential designs and/or simulated datasets. This can be expensive and prohibit scaling up these methods to models with many parameters, or designs with many unknowns to select. We introduce an efficient alternative approach without posterior calculations, based on optimising the expected trace of the Fisher information, as discussed by Walker (2016). We illustrate drawbacks of this approach, including lack of invariance to reparameterisation and encouraging designs in which one parameter combination is inferred accurately but not any others. We show these can be avoided by using an adversarial approach: the experimenter must select their design while a critic attempts to select the least favourable parameterisation. We present theoretical properties of this approach and show it can be used with gradient based optimisation methods to find designs efficiently in practice.
更多查看译文
关键词
automatic differentiation,game theory,geostatistical regression,Hyva?rinen score,minimax optimisation,pharmacokinetics
AI 理解论文
溯源树
样例
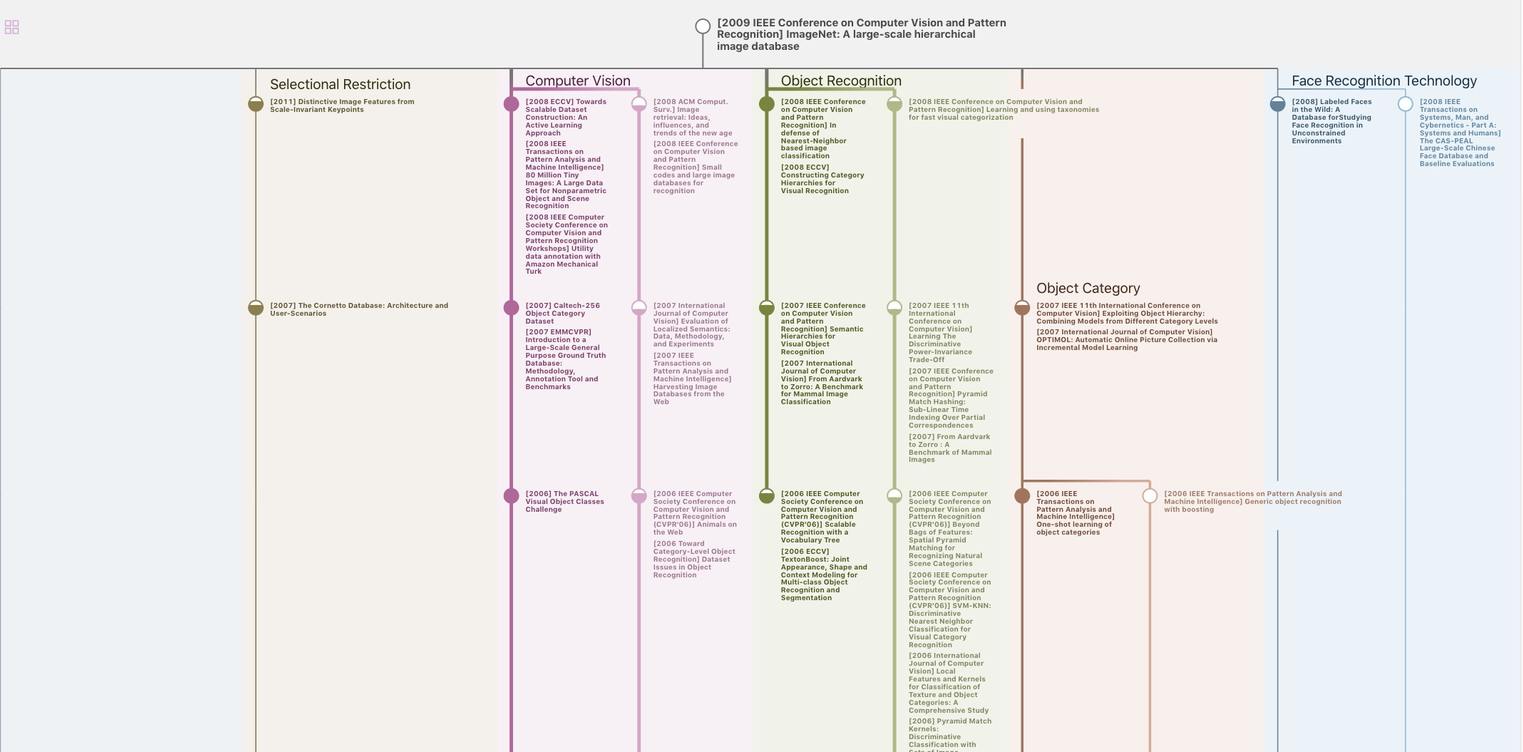
生成溯源树,研究论文发展脉络
Chat Paper
正在生成论文摘要