An Improved Random Forest Algorithm for Predicting Employee Turnover
MATHEMATICAL PROBLEMS IN ENGINEERING(2019)
摘要
Employee turnover is considered a major problem for many organizations and enterprises. The problem is critical because it affects not only the sustainability of work but also the continuity of enterprise planning and culture. Therefore, human resource departments are paying greater attention to employee turnover seeking to improve their understanding of the underlying reasons and main factors. To address this need, this study aims to enhance the ability to forecast employee turnover and introduce a new method based on an improved random forest algorithm. The proposed weighted quadratic random forest algorithm is applied to employee turnover data with high-dimensional unbalanced characteristics. First, the random forest algorithm is used to order feature importance and reduce dimensions. Second, the selected features are used with the random forest algorithm and the F-measure values are calculated for each decision tree as weights to build the prediction model for employee turnover. In the area of employee turnover forecasting, compared with the random forest, C4.5, Logistic, BP, and other algorithms, the proposed algorithm shows significant improvement in terms of various performance indicators, specifically recall and F-measure. In the experiment using the employee dataset of a branch of a communications company in China, the key factors influencing employee turnover were identified as monthly income, overtime, age, distance from home, years at the company, and percent of salary increase. Among them, monthly income and overtime were the two most important factors. The study offers a new analytic method that can help human resource departments predict employee turnover more accurately and its experimental results provide further insights to reduce employee turnover intention.
更多查看译文
关键词
improved random forest algorithm,employee
AI 理解论文
溯源树
样例
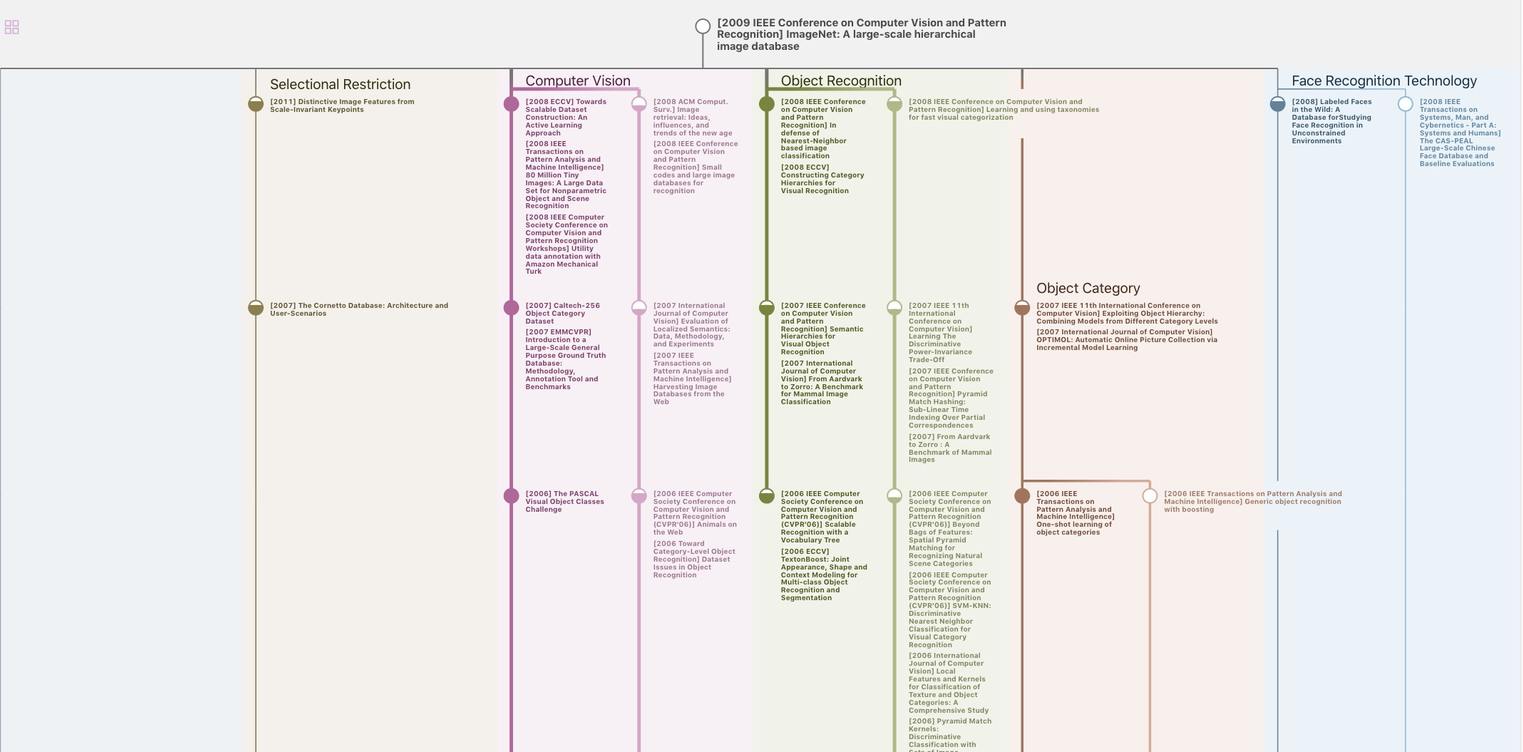
生成溯源树,研究论文发展脉络
Chat Paper
正在生成论文摘要