Learning Disentangled Representation In Latent Stochastic Models: A Case Study With Image Captioning
2019 IEEE INTERNATIONAL CONFERENCE ON ACOUSTICS, SPEECH AND SIGNAL PROCESSING (ICASSP)(2019)
摘要
Multimodal tasks require learning joint representation across modalities. In this paper, we present an approach to employ latent stochastic models for a multimodal task - image captioning. Encoder Decoder models with stochastic latent variables are often faced with optimization issues such as latent collapse preventing them from realizing their full potential of rich representation learning and disentanglement. We present an approach to train such models by incorporating joint continuous and discrete representation in the prior distribution. We evaluate the performance of proposed approach on a multitude of metrics against vanilla latent stochastic models. We also perform a qualitative assessment and observe that the proposed approach indeed has the potential to learn composite information and explain novel combinations not seen in the training data.
更多查看译文
关键词
disentanglement, latent representation, captioning, composition, multimodal, continuous, discrete
AI 理解论文
溯源树
样例
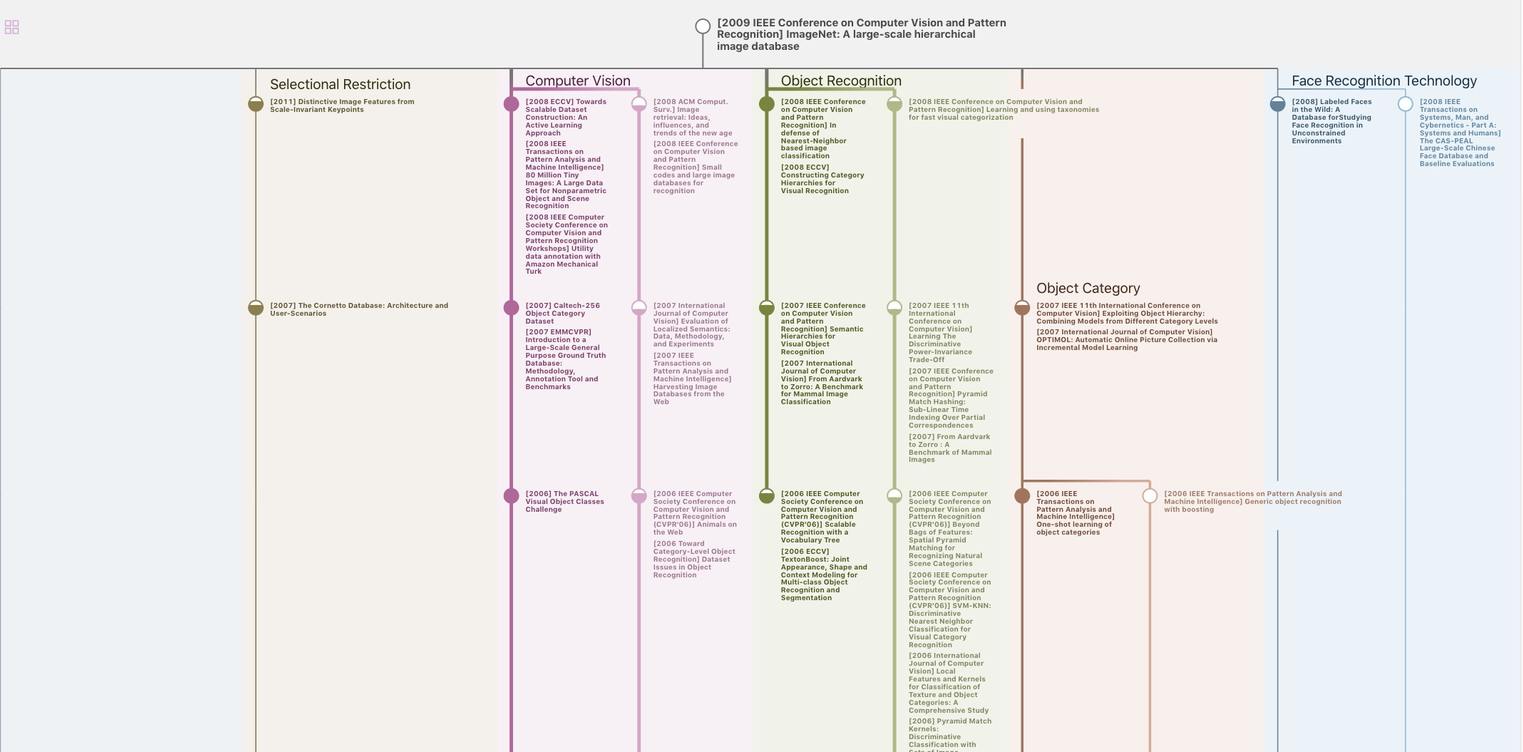
生成溯源树,研究论文发展脉络
Chat Paper
正在生成论文摘要