Identification and Asymptotic Localization of Rumor Sources Using the Method of Types
IEEE Transactions on Network Science and Engineering(2020)
摘要
We are interested in identifying a rumor source on a tree network. We begin with extended star networks under the SI infection model with exponential waiting times. We present and analyze the types center, a highly tractable approximation of the ML source estimate, obtained using the method of types. We empirically show that this approximate ML estimator is exact for some small test cases. We prove that the approximation error is at most logarithmic in infection size on large networks, providing highly efficient source identification (especially compared to the accuracy in similar problems, such as the O(√n) best possible n accuracy estimate in a line network). We also show that the qualitative properties of the types and rumor centers are different on extended star networks. We further propose a heuristic-based generalization of this approach to trees: the relative-leaf counting algorithm. In simulations on regular and nonregular trees, types center's performance is competitive with rumor centrality (which is optimal for d-regular trees), while requiring less computation time. In addition to providing a faster (and sometimes more accurate) alternative on its own, our approach could potentially be used with rumor centrality to improve results with less than twice the total computation time.
更多查看译文
关键词
Network problems,probability and statistics,symbolic and algebraic manipulation,performance evaluation of algorithms and systems,heuristic design,graph and tree search strategies,Trees.
AI 理解论文
溯源树
样例
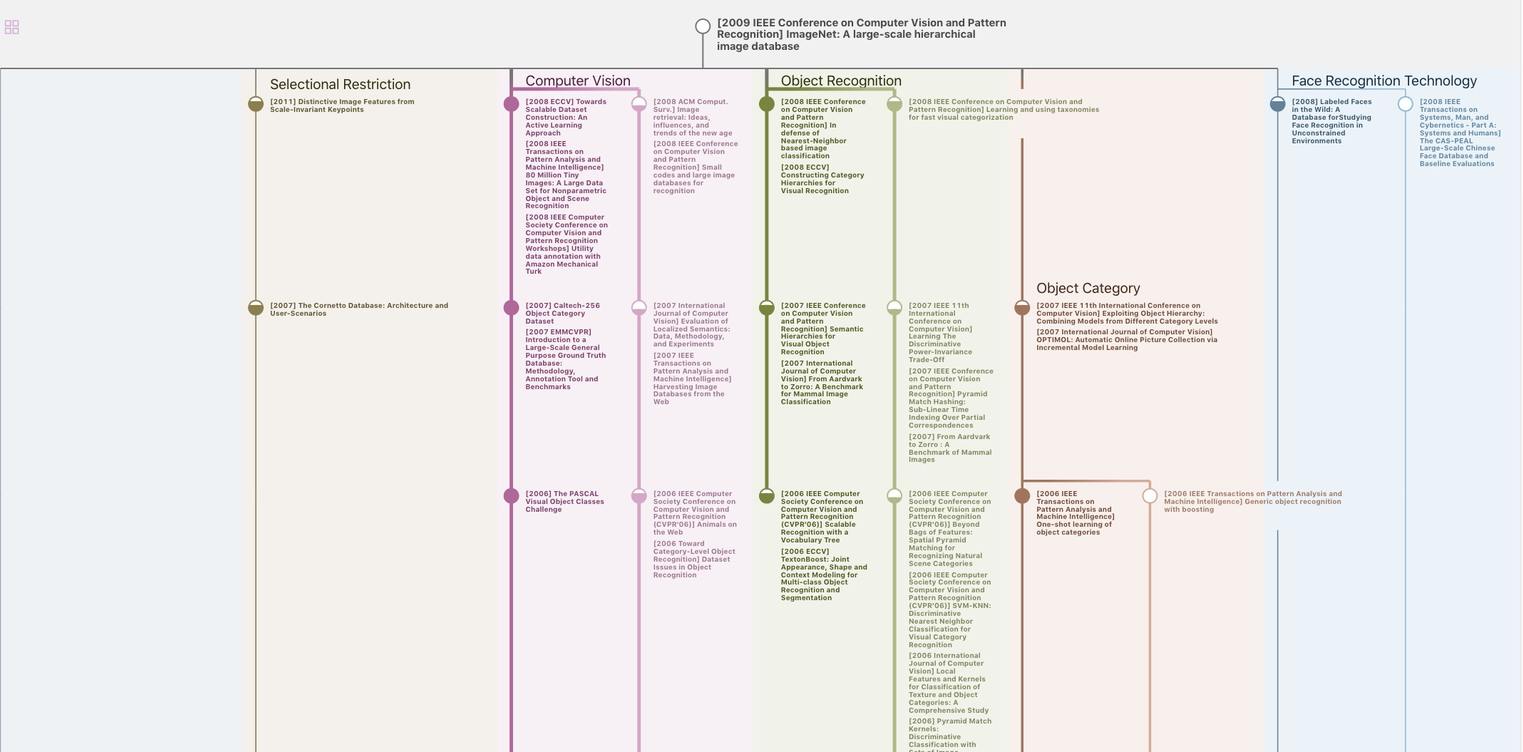
生成溯源树,研究论文发展脉络
Chat Paper
正在生成论文摘要