MP63-04 QUANTITATIVE NUCLEAR FEATURES OF HEMATOXYLIN-EOSIN SPECIMENS FROM INITIAL TRANSURETHRAL RESECTION FOR PREDICTING NON-MUSCLE INVASIVE BLADDER CANCER RECURRENCE
The Journal of urology/The journal of urology(2019)
摘要
You have accessJournal of UrologyBladder Cancer: Basic Research & Pathophysiology III (MP63)1 Apr 2019MP63-04 QUANTITATIVE NUCLEAR FEATURES OF HEMATOXYLIN-EOSIN SPECIMENS FROM INITIAL TRANSURETHRAL RESECTION FOR PREDICTING NON-MUSCLE INVASIVE BLADDER CANCER RECURRENCE Naoto Tokuyama*, Akira Saito, Yosuke Hirasawa, Takeshi Hashimoto, Naoya Satake, Noriko Watanabe, Tatsuo Gondo, Yoshihiro Nakagami, Kazunori Namiki, Masahiko Kuroda, and Yoshio Ohno Naoto Tokuyama*Naoto Tokuyama* More articles by this author , Akira SaitoAkira Saito More articles by this author , Yosuke HirasawaYosuke Hirasawa More articles by this author , Takeshi HashimotoTakeshi Hashimoto More articles by this author , Naoya SatakeNaoya Satake More articles by this author , Noriko WatanabeNoriko Watanabe More articles by this author , Tatsuo GondoTatsuo Gondo More articles by this author , Yoshihiro NakagamiYoshihiro Nakagami More articles by this author , Kazunori NamikiKazunori Namiki More articles by this author , Masahiko KurodaMasahiko Kuroda More articles by this author , and Yoshio OhnoYoshio Ohno More articles by this author View All Author Informationhttps://doi.org/10.1097/01.JU.0000556847.87489.0aAboutPDF ToolsAdd to favoritesDownload CitationsTrack CitationsPermissionsReprints ShareFacebookLinked InTwitterEmail Abstract INTRODUCTION AND OBJECTIVES: In non-muscle invasive bladder cancer (NMIBC), clinical management is often based on risk classification with limited subjective tools. Recent development in artificial intelligence and digital pathology has promoted the use of this technology in cancer diagnosis. Analysis of digitalized hematoxylin-eosin (HE) tissue section images contributes to quantitative evaluation of cancer lesion morphology. We aimed to develop a novel prediction model for intravesical recurrence based on computer measured nuclear morphological features in NMIBC cases. METHODS: We investigated 30 patients diagnosed with NMIBC. A pathologist annotated digitalized HE tissue slides for bladder cancer. Nuclei were segmented from the annotated region of interest (ROI). Following image processing techniques, we assessed 992 nuclear morphological features from each nucleus e.g., nucleus size, roundness, contour length, intra-nucleus texture data. To identify specific features useful for intravesical recurrence prediction, a multiclass stepwise discriminant analysis was done. We performed canonical discriminant analysis to obtain recurrence probability for each patient. We also applied support vector machine (SVM) to 24 cases; we validated the prediction model by testing 6 cases. RESULTS: From the digitalized HE tissue slide images, 317 ROIs were annotated. We examined 347,744 nuclei. Based on stepwise discriminant analysis, we selected 49 specific features. Canonical discriminant analysis showed a predictive accuracy of 100%. Table 1 shows results of the SVM model that used 64 images obtained from 6 patients; the accuracy, sensitivity, and specificity were 84%, 91%, and 73%, respectively. The predictive model showed that 8 and 3 images were false positive and false negative, respectively. CONCLUSIONS: Our results suggest that a novel prediction model can be created by SVM learning of computer extracted nuclear morphological features in NMIBC cases. Source of Funding: none Tokyo, Japan© 2019 by American Urological Association Education and Research, Inc.FiguresReferencesRelatedDetails Volume 201Issue Supplement 4April 2019Page: e897-e898 Advertisement Copyright & Permissions© 2019 by American Urological Association Education and Research, Inc.MetricsAuthor Information Naoto Tokuyama* More articles by this author Akira Saito More articles by this author Yosuke Hirasawa More articles by this author Takeshi Hashimoto More articles by this author Naoya Satake More articles by this author Noriko Watanabe More articles by this author Tatsuo Gondo More articles by this author Yoshihiro Nakagami More articles by this author Kazunori Namiki More articles by this author Masahiko Kuroda More articles by this author Yoshio Ohno More articles by this author Expand All Advertisement PDF downloadLoading ...
更多查看译文
AI 理解论文
溯源树
样例
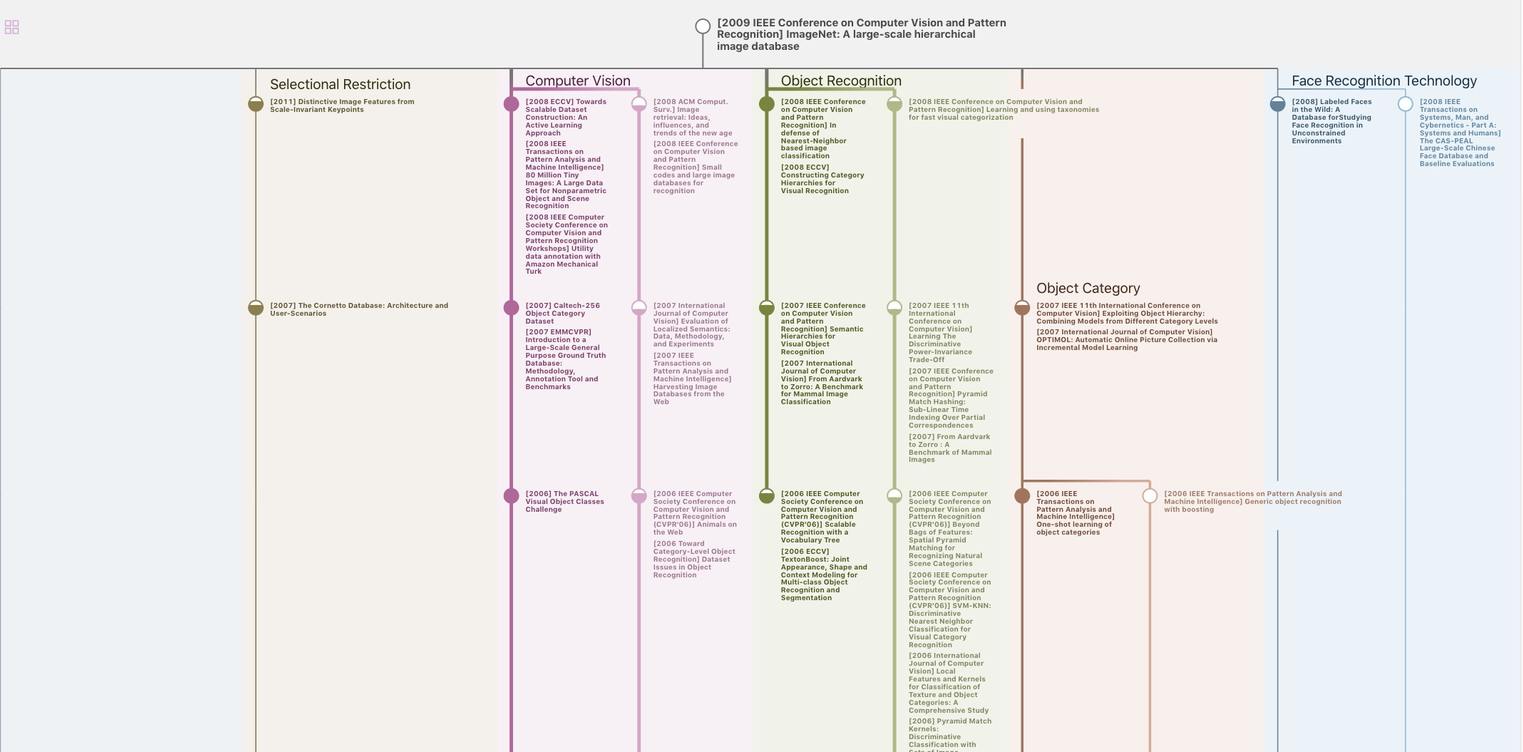
生成溯源树,研究论文发展脉络
Chat Paper
正在生成论文摘要