Choice of link and variance function for generalized linear mixed models: a case study with binomial response in proteomics
COMMUNICATIONS IN STATISTICS-THEORY AND METHODS(2020)
摘要
Non-normality is a common phenomenon in data from agricultural and biological research, especially in molecular data (for example; -omics, RNAseq, flow cytometric data, etc.). For over half a century, the leading paradigm called for using analysis of variance (ANOVA) after applying a data transformation. The introduction of generalized linear mixed models (GLMM) provides a new way of analyzing non-normal data. Selecting an apt link function in GLMM can be quite influential, however, and is as critical as selecting an appropriate transformation for ANOVA. In this paper, we assess the performance of different parametric link families available in literature. Then, we propose a new estimation method for selecting an appropriate link function with a suitable variance function in a quasi-likelihood framework. We apply these methods to a proteomics data set, showing that GLMMs provide a very flexible framework for analyzing these kinds of data.
更多查看译文
关键词
Proteomics,quasi-likelihood,GLMM,ANOVA,link function,variance function
AI 理解论文
溯源树
样例
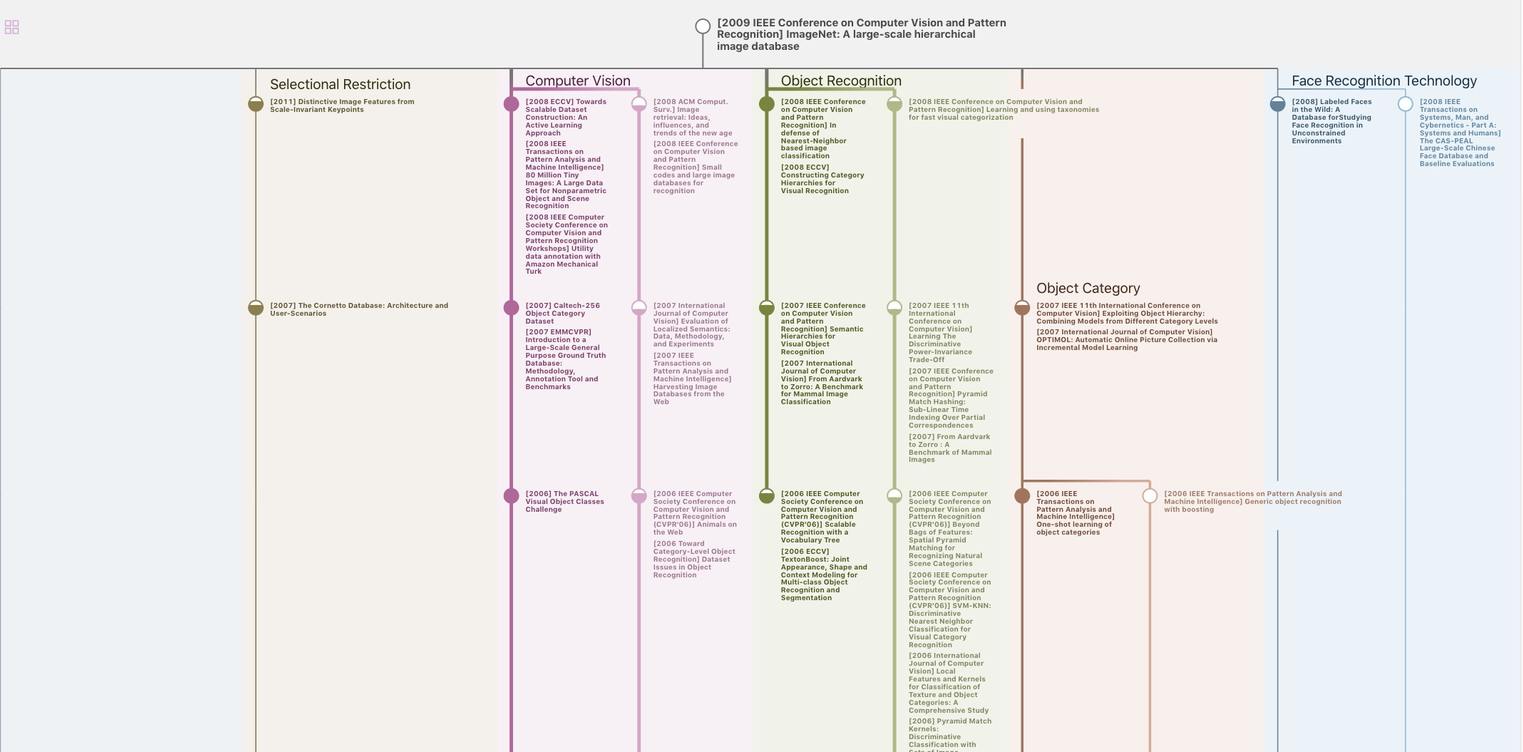
生成溯源树,研究论文发展脉络
Chat Paper
正在生成论文摘要