Multiple Reference Points based Decomposition for Multi-objective Feature Selection in Classification: Static and Dynamic Mechanisms
IEEE Transactions on Evolutionary Computation(2020)
摘要
Feature selection is an important task in machine learning that has two main objectives: 1) reducing dimensionality and 2) improving learning performance. Feature selection can be considered a multiobjective problem. However, it has its problematic characteristics, such as a highly discontinuous Pareto front, imbalance preferences, and partially conflicting objectives. These characteristics are not easy for existing evolutionary multiobjective optimization (EMO) algorithms. We propose a new decomposition approach with two mechanisms (static and dynamic) based on multiple reference points under the multiobjective evolutionary algorithm based on decomposition (MOEA/D) framework to address the above-mentioned difficulties of feature selection. The static mechanism alleviates the dependence of the decomposition on the Pareto front shape and the effect of the discontinuity. The dynamic one is able to detect regions in which the objectives are mostly conflicting, and allocates more computational resources to the detected regions. In comparison with other EMO algorithms on 12 different classification datasets, the proposed decomposition approach finds more diverse feature subsets with better performance in terms of hypervolume and inverted generational distance. The dynamic mechanism successfully identifies conflicting regions and further improves the approximation quality for the Pareto fronts.
更多查看译文
关键词
Feature extraction,Heuristic algorithms,Optimization,Task analysis,Approximation algorithms,Sociology,Statistics
AI 理解论文
溯源树
样例
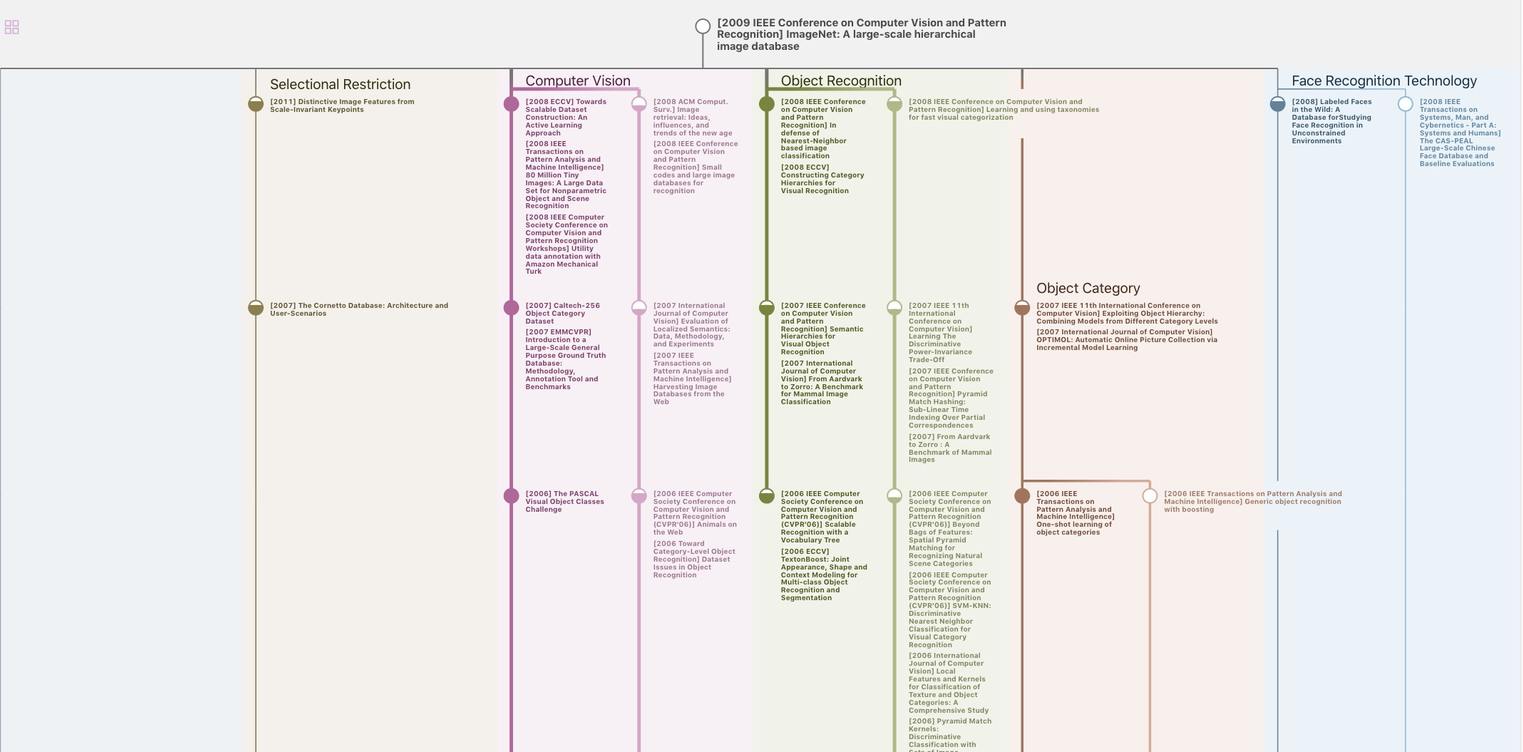
生成溯源树,研究论文发展脉络
Chat Paper
正在生成论文摘要