Non-linear Kalman Filters Comparison for Generalised Autoregressive Conditional Heteroscedastic Clutter Parameter Estimation
IET signal processing(2019)
摘要
In this work, the authors analyse the estimation of the generalised autoregressive conditional heteroscedastic (GARCH) process conditional variance based on three non-linear filtering approaches: extended Kalman filter (EKF), unscented Kalman filter and cubature Kalman filter. The authors present a state model for a GARCH process and derive an EKF including second-order non-linear terms for simultaneous estimation of state and parameters. Using synthetic data, the authors evaluate the consistency and the correlation of the innovations for the three filters, by means of numerical simulations. The authors also study the performance of smoothed versions of the non-linear Kalman filters using real clutter data in comparison with a conventional quasi-maximum likelihood estimation method for the GARCH process coefficients. The authors show that with all methods the process coefficients estimates are of the same order and the resulting conditional variances are commensurable. However, the non-linear Kalman filters greatly reduce the computational load. These kind of filters could be used for the radar detector based on a GARCH clutter model that uses an adaptive threshold that demands the conditional variance at each decision instant.
更多查看译文
关键词
radar clutter,autoregressive processes,radar detection,Kalman filters,nonlinear filters,parameter estimation,nonlinear Kalman filters,GARCH process coefficients,unscented Kalman filter,cubature Kalman filter,second-order nonlinear terms,generalised autoregressive conditional heteroscedastic clutter,parameter estimation,GARCH process conditional variance,extended Kalman filter,numerical simulations,radar detector
AI 理解论文
溯源树
样例
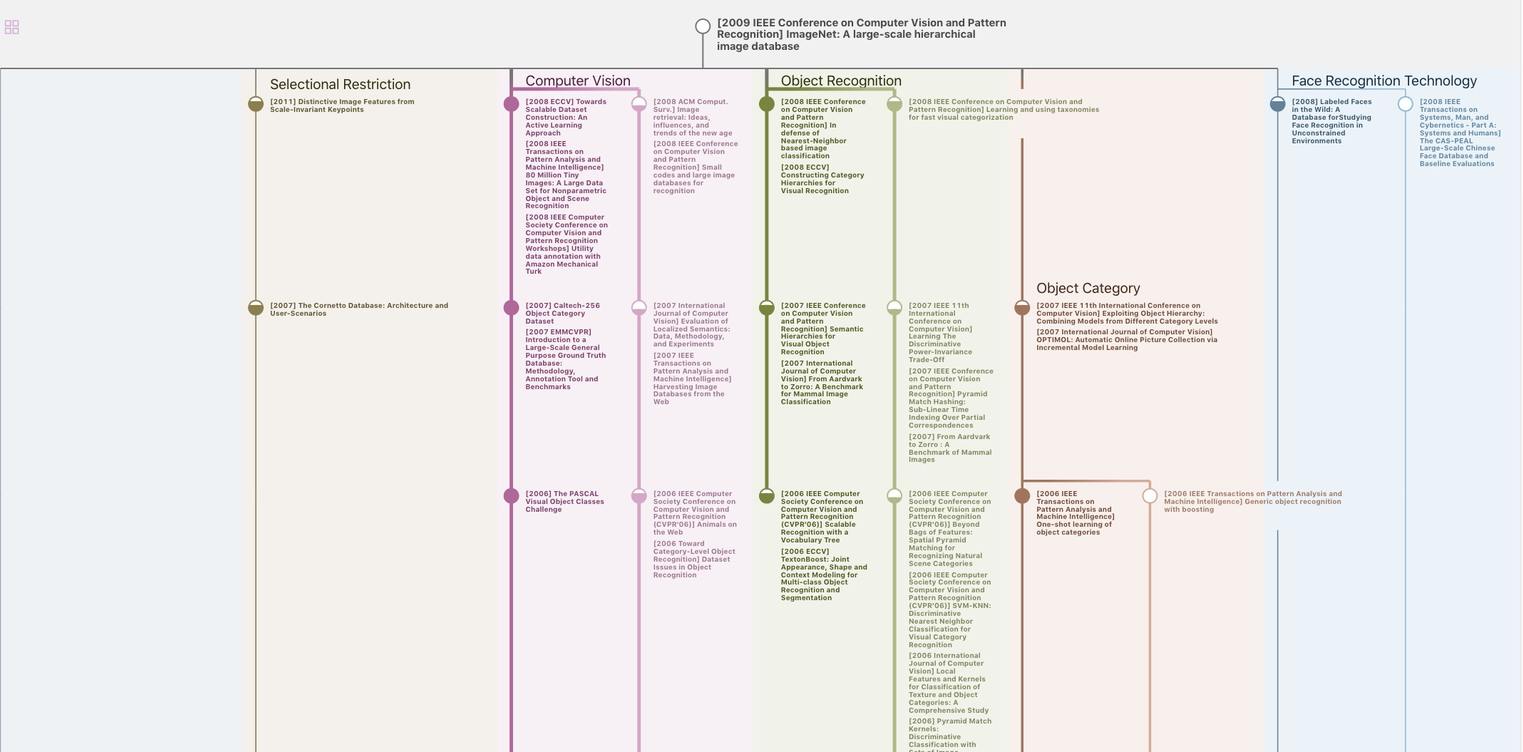
生成溯源树,研究论文发展脉络
Chat Paper
正在生成论文摘要