A Multi-Attributed Graph-Based Approach for Text Data Modeling and Event Detection in Twitter.
2019 11th International Conference on Communication Systems & Networks (COMSNETS)(2019)
摘要
The popularity of the microblogging sites like Twitter is increasing exponentially in which users are allowed to post short messages (aka tweets) using a maximum of 280 characters, mainly for news sharing and events updates. Besides, textual data, Twitter data also contains multi-dimensional connections among the users if they follow each other or have common followers/followees. Similarly, multi-dimensional connections exist among the tweets if they contain common hashtags, mentions, etc. In recent years, Word2Vec is being extensively used to analyze textual data, and it has shown promising results in many domains. In this paper, we propose a multi-attributed graph-based approach for text data modeling and event detection in Twitter. To this end, we generate a multi-attributed social graph (MASG), in which nodes, representing tweets, are labelled with numeric vectors obtained through Word2Vec model, and edges represent the structural relationships among the tweets and they can also be labeled with numeric vectors. Thereafter, MASG is converted into a similarity graph using a distance function, and Markov clustering algorithm is applied over the similarity graph to identify different clusters, where each cluster corresponds to a particular event. The proposed approach is evaluated over real-world Twitter datasets using standard evaluation metrics including TPR and FPR. It is also compared with some baseline methods and performs significantly better.
更多查看译文
关键词
Social Network Analysis,Text Data Modeling,Word2Vec,Multi-Attributed Social Graph,Markov Clustering,Event Detection
AI 理解论文
溯源树
样例
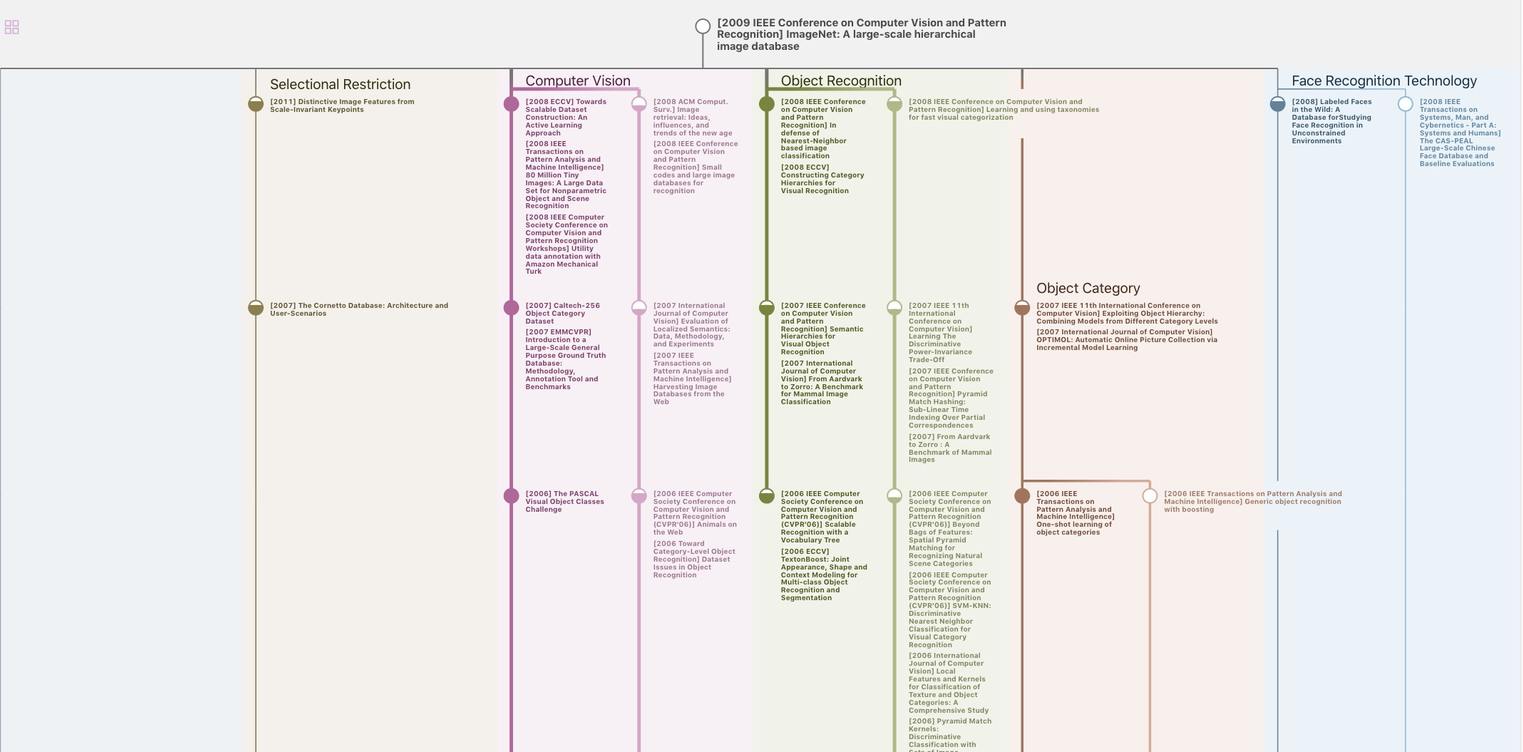
生成溯源树,研究论文发展脉络
Chat Paper
正在生成论文摘要