Few-Shot Adversarial Learning of Realistic Neural Talking Head Models
2019 IEEE/CVF INTERNATIONAL CONFERENCE ON COMPUTER VISION (ICCV 2019)(2019)
摘要
Several recent works have shown how highly realistic human head images can be obtained by training convolutional neural networks to generate them. In order to create a personalized talking head model, these works require training on a large dataset of images of a single person. However, in many practical scenarios, such personalized talking head models need to be learned from a few image views of a person, potentially even a single image. Here, we present a system with such few-shot capability. It performs lengthy meta-learning on a large dataset of videos, and after that is able to frame few- and one-shot learning of neural talking head models of previously unseen people as adversarial training problems with high capacity generators and discriminators. Crucially, the system is able to initialize the parameters of both the generator and the discriminator in a person-specific way, so that training can be based on just a few images and done quickly, despite the need to tune tens of millions of parameters. We show that such an approach is able to learn highly realistic and personalized talking head models of new people and even portrait paintings.
更多查看译文
关键词
person-specific way,realistic neural talking head models,highly realistic human head images,convolutional neural networks training,personalized talking head model,image views,adversarial training problems,high capacity generators,few-shot adversarial learning
AI 理解论文
溯源树
样例
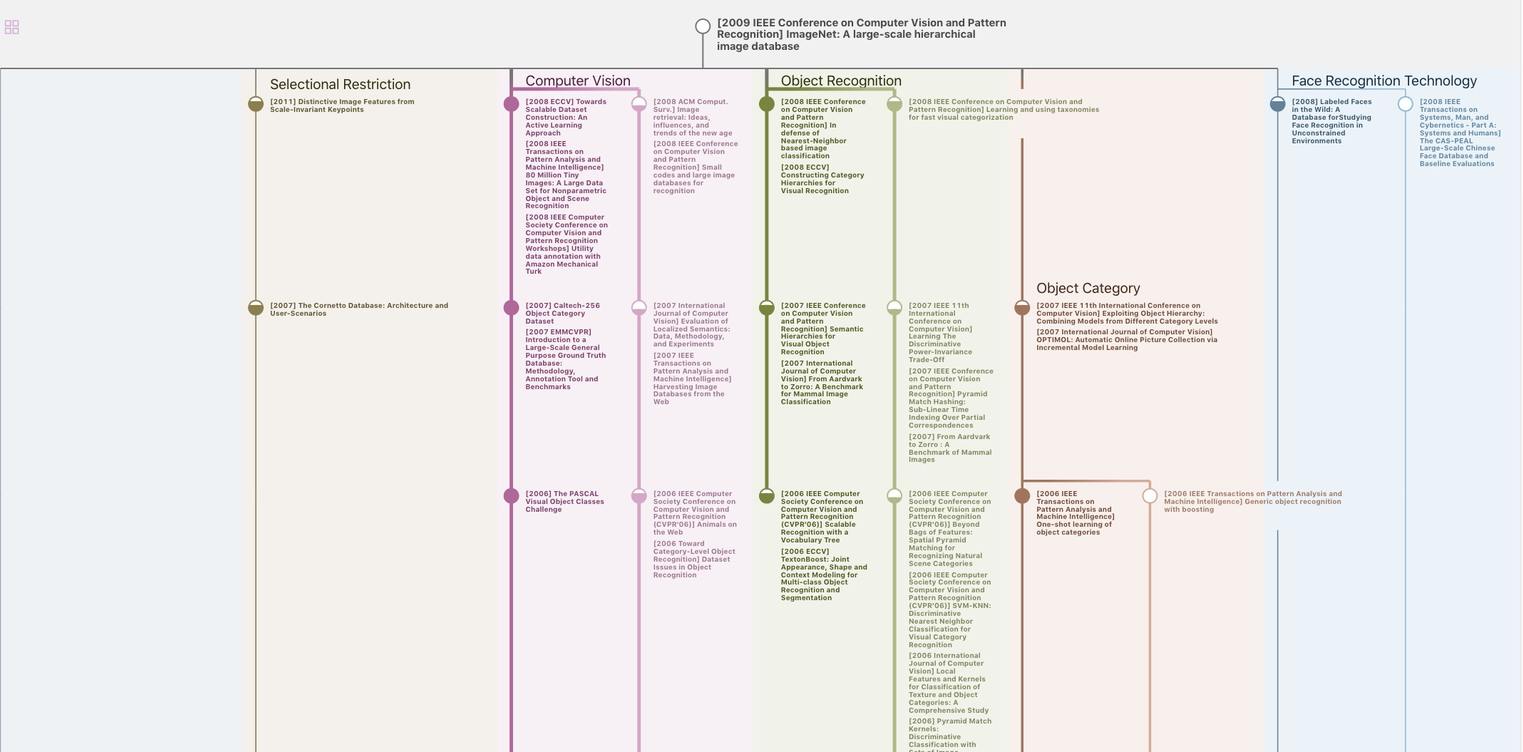
生成溯源树,研究论文发展脉络
Chat Paper
正在生成论文摘要