Data-Driven Preference Learning Methods for Value-Driven Multiple Criteria Sorting with Interacting Criteria
INFORMS JOURNAL ON COMPUTING(2021)
摘要
The learning of predictive models for data-driven decision support has been a prevalent topic in many fields. However, construction of models that would capture interactions among input variables is a challenging task. In this paper, we present a new preference learning approach for multiple criteria sorting with potentially interacting criteria. It employs an additive piecewise-linear value function as the basic preference model, which is augmented with components for handling the interactions. To construct such a model from a given set of assignment examples concerning reference alternatives, we develop a convex quadratic programming model. Because its complexity does not depend on the number of training samples, the proposed approach is capable for dealing with data-intensive tasks. To improve the generalization of the constructed model on new instances and to overcome the problem of overfitting, we employ the regularization techniques. We also propose a few novel methods for classifying nonreference alternatives in order to enhance the applicability of our approach to different data sets. The practical usefulness of the proposed approach is demonstrated on a problem of parametric evaluation of research units, whereas its predictive performance is studied on several monotone classification problems. The experimental results indicate that our approach compares favourably with the classical UTilites Additives DIScriminantes (UTADIS) method and the Choquet integral-based sorting model. Summary of Contribution. The paper tackles vital challenges at the intersections of multiple criteria decision analysis and machine learning, showing how computationally advanced techniques can be used for faithfully representing human preferences and dealing with complex decision problems. Specifically, we propose a novel preference learning method for multiple criteria sorting problems. The introduced approach incorporates convex quadratic programming to construct a value-based preference model based on large sets of preference statements. In this way, we extend the applicability of decision analysis methods to preferences derived from historical data or observation of users' behavior in addition to the preference judgments explicitly revealed by the decision-makers. The method's practical usefulness is illustrated on a variety of real-world datasets from fields such as higher education, medicine, human resources, and housing market. Its potential for supporting better decision-making is enhanced by both an interpretable form of the assumed model handling interactions between criteria as well as a high predictive performance demonstrated in the extensive computational experiments.
更多查看译文
关键词
preference learning,decision analysis,sorting,ordinal classification,additive value function,interacting criteria
AI 理解论文
溯源树
样例
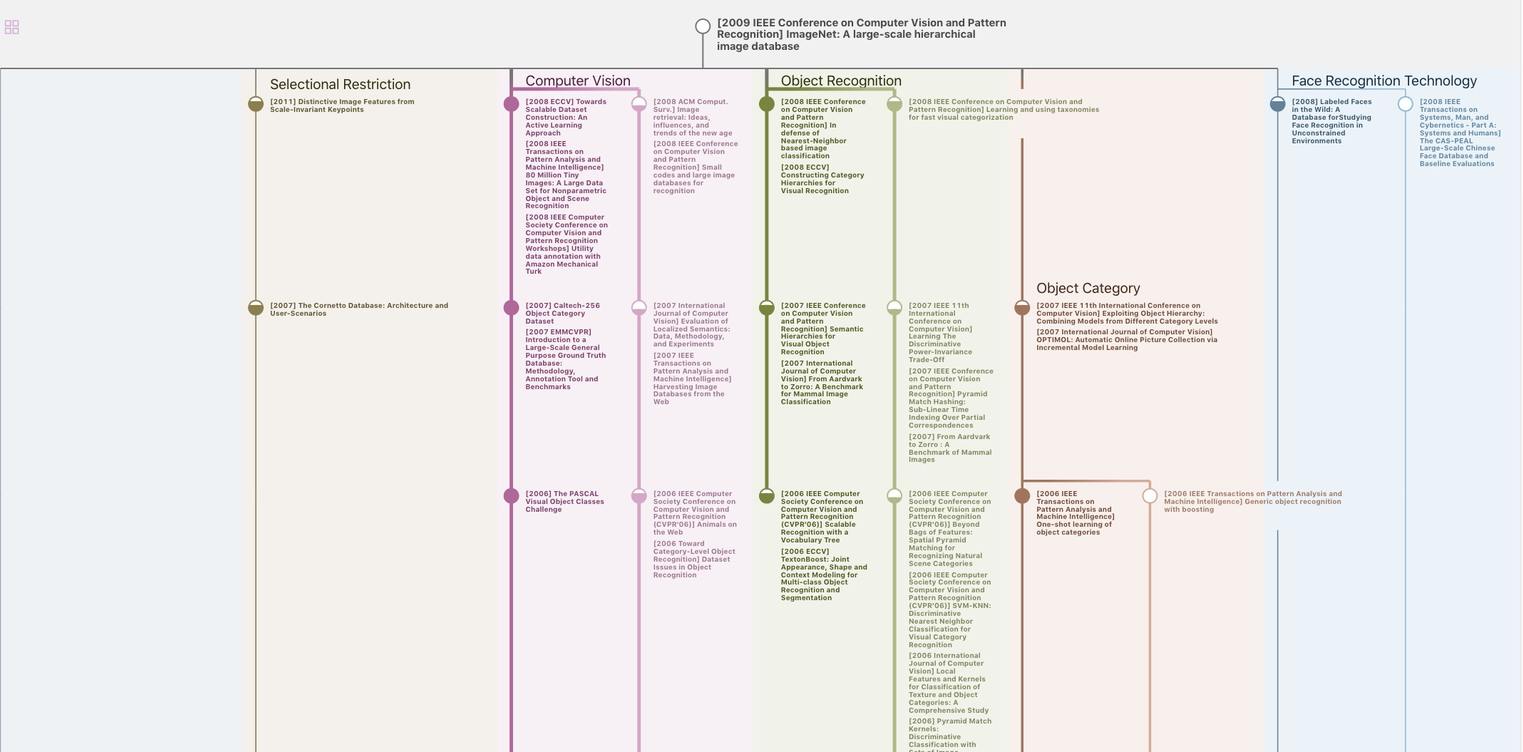
生成溯源树,研究论文发展脉络
Chat Paper
正在生成论文摘要