Multi-Class Gaussian Process Classification Made Conjugate: Efficient Inference via Data Augmentation
35TH UNCERTAINTY IN ARTIFICIAL INTELLIGENCE CONFERENCE (UAI 2019)(2020)
摘要
We propose a new scalable multi-class Gaussian process classification approach building on a novel modified softmax likelihood function. The new likelihood has two benefits: it leads to well-calibrated uncertainty estimates and allows for an efficient latent variable augmentation. The augmented model has the advantage that it is conditionally conjugate leading to a fast variational inference method via block coordinate ascent updates. Previous approaches suffered from a trade-off between uncertainty calibration and speed. Our experiments show that our method leads to well-calibrated uncertainty estimates and competitive predictive performance while being up to two orders faster than the state of the art.
更多查看译文
关键词
Incremental Learning,Robust Learning,Change Detection,Variational Inference,Ensemble Learning
AI 理解论文
溯源树
样例
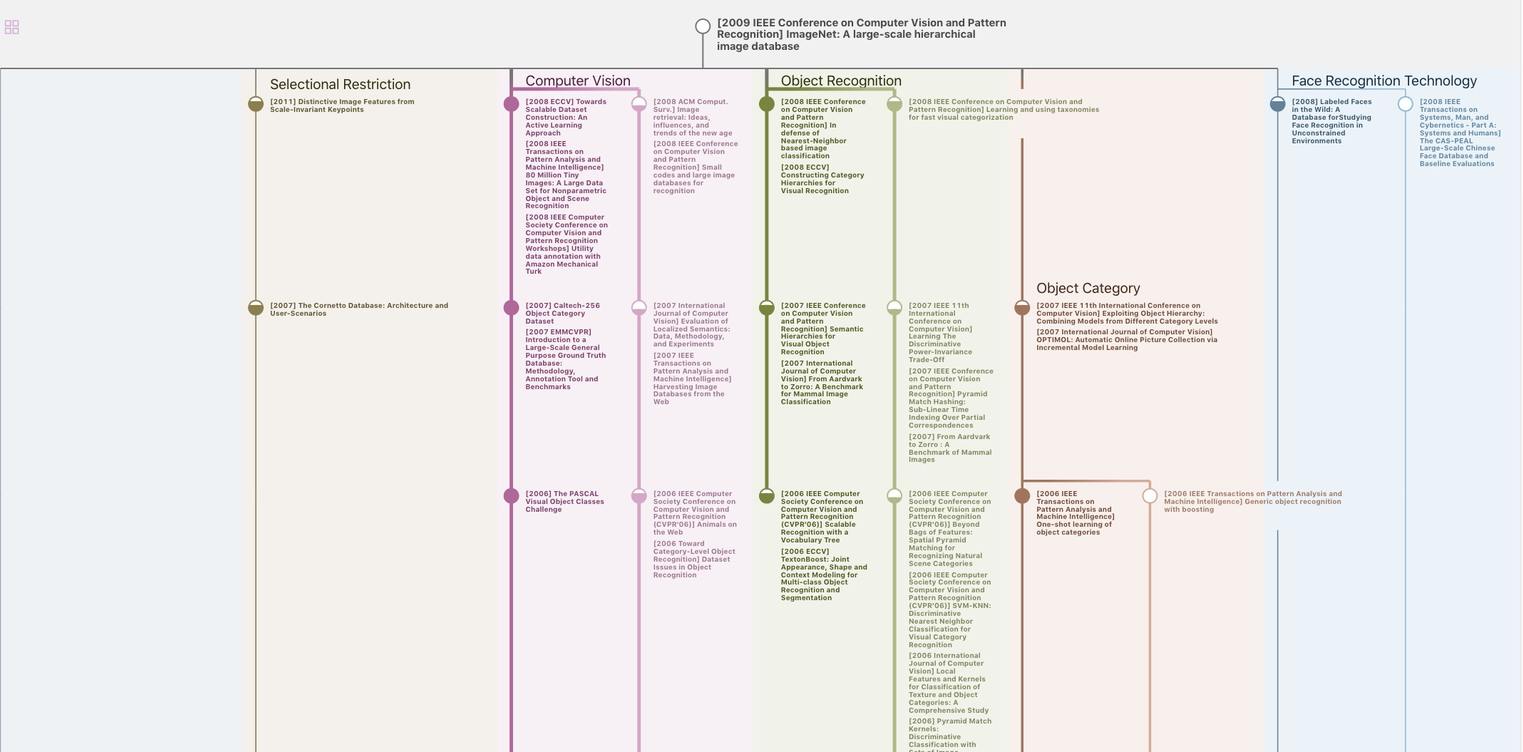
生成溯源树,研究论文发展脉络
Chat Paper
正在生成论文摘要