Adversarial Attack against Modeling Attack on PUFs
Proceedings of the 56th Annual Design Automation Conference 2019(2019)
摘要
The Physical Unclonable Function (PUF) has been proposed for the identification and authentication of devices and cryptographic key generation. A strong PUF provides an extremely large number of device-specific challenge-response pairs (CRP) which can be used for identification. Unfortunately, the CRP mechanism is vulnerable to modeling attack, which uses machine learning (ML) algorithms to predict PUF responses with high accuracy. Many methods have been developed to strengthen strong PUFs with complicated hardware; however, recent studies show that they are still vulnerable by leveraging GPU-accelerated ML algorithms.
In this paper, we propose to deal with the problem from a different approach. With a slightly modified CRP mechanism, a PUF can provide poison data such that an accurate model of the PUF under attack cannot be built by ML algorithms. Experimental results show that the proposed method provides an effective countermeasure against modeling attacks on PUF. In addition, the proposed method is compatible with hardware strengthening schemes to provide even better protection for PUFs.
更多查看译文
关键词
Adversarial attack, Machine learning, Modeling attack, Physical unclonable function (PUF)
AI 理解论文
溯源树
样例
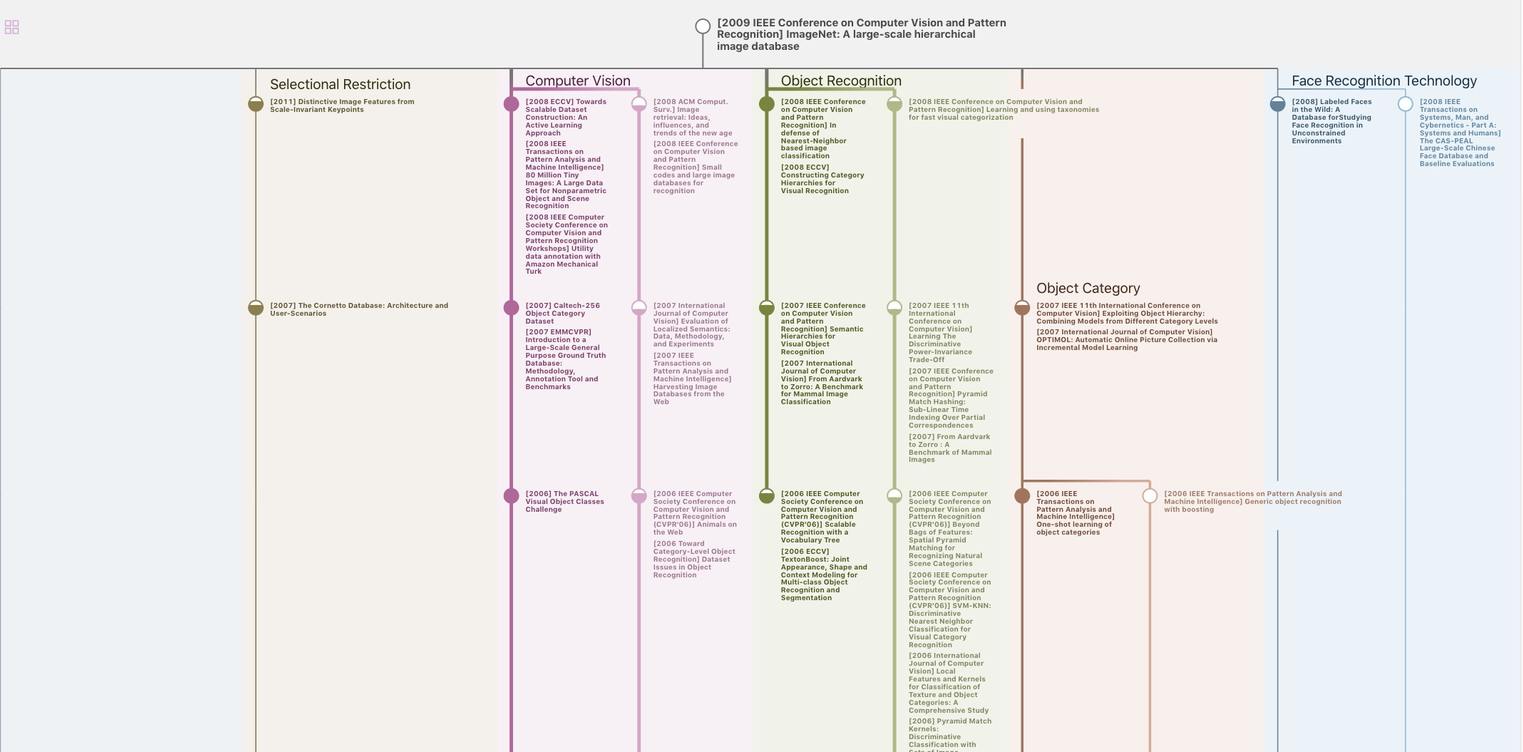
生成溯源树,研究论文发展脉络
Chat Paper
正在生成论文摘要