On-Chip Memory Technology Design Space Explorations for Mobile Deep Neural Network Accelerators
Proceedings of the 56th Annual Design Automation Conference 2019(2019)
摘要
Deep neural network (DNN) inference tasks have become ubiquitous workloads on mobile SoCs and demand energy-efficient hardware accelerators. Mobile DNN accelerators are heavily area-constrained, with only minimal on-chip SRAM, which results in heavy use of inefficient off-chip DRAM. With diminishing returns from conventional silicon technology scaling, emerging memory technologies that offer better area density than SRAM can boost accelerator efficiency by minimizing costly off-chip DRAM accesses. This paper presents a detailed design space exploration (DSE) of technology-system co-design for systolic-array accelerators. We focus on practical/mature on-chip memory technologies, including SRAM, eDRAM, MRAM, and 3D vertical RRAM (VRRAM). The DSE employs state-of-the-art optimizations (e.g., model compression and optimized buffer scheduling), and evaluates results on important models including ResNet-50, MobileNet, and Faster-RCNN. Compared to an SRAM/DRAM baseline, MRAM-based accelerators show up to 4.68× energy benefits (57% area overhead), while a 3D VRRAM-based design achieves 2.22× energy benefits (33% area reduction).
更多查看译文
关键词
off-chip DRAM,demand energy-efficient hardware accelerators,eDRAM,3D vertical RRAM,ResNet-50,MobileNet,faster-RCNN,3D VRRAM-based design,MRAM-based accelerators,on-chip memory technologies,systolic-array accelerators,technology-system co-design,DSE,area density,conventional silicon technology scaling,on-chip SRAM,mobile DNN accelerators,mobile SoCs,ubiquitous workloads,deep neural network inference tasks,mobile deep neural network accelerators,chip memory technology design space explorations
AI 理解论文
溯源树
样例
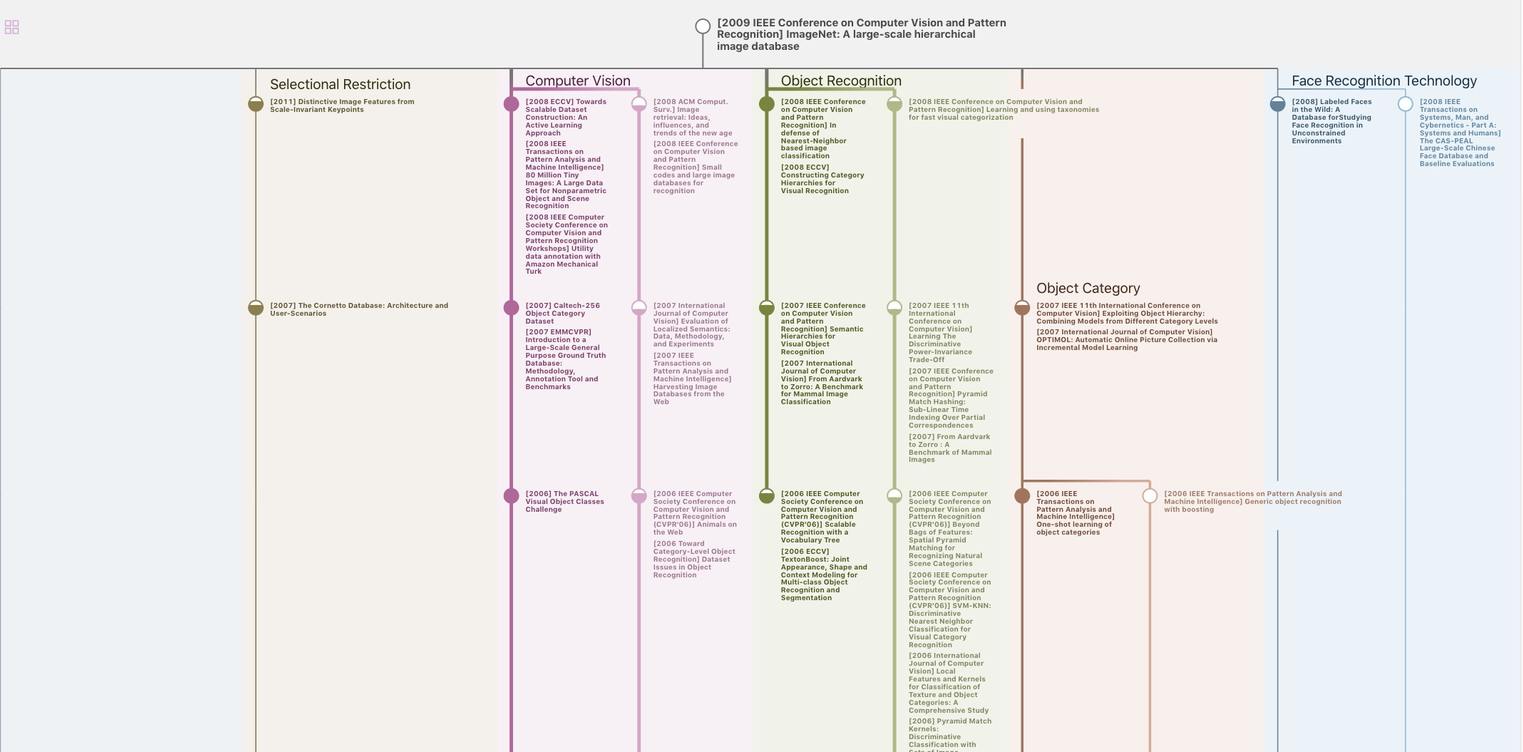
生成溯源树,研究论文发展脉络
Chat Paper
正在生成论文摘要