LODESTAR: Creating Locally-Dense CNNs for Efficient Inference on Systolic Arrays
Proceedings of the 56th Annual Design Automation Conference 2019(2019)
摘要
The performance of sparse problems suffers from lack of spatial locality and low memory bandwidth utilization. However, the distribution of non-zero values in the data structures of a class of sparse problems, such as matrix operations in neural networks, is modifiable so that it can be matched with an efficient underlying hardware, such as systolic arrays. Such modification helps addressing the challenges coupled with sparsity. To efficiently execute sparse neural network inference on systolic arrays, we propose a structured pruning algorithm that increases the spatial locality in neural network models, while maintaining the accuracy of inference.
更多查看译文
关键词
neural network models,LODESTAR,spatial locality,low memory bandwidth utilization,nonzero values,data structures,matrix operations,neural networks,sparse neural network inference,structured pruning algorithm,locally-dense CNNs,systolic arrays inference
AI 理解论文
溯源树
样例
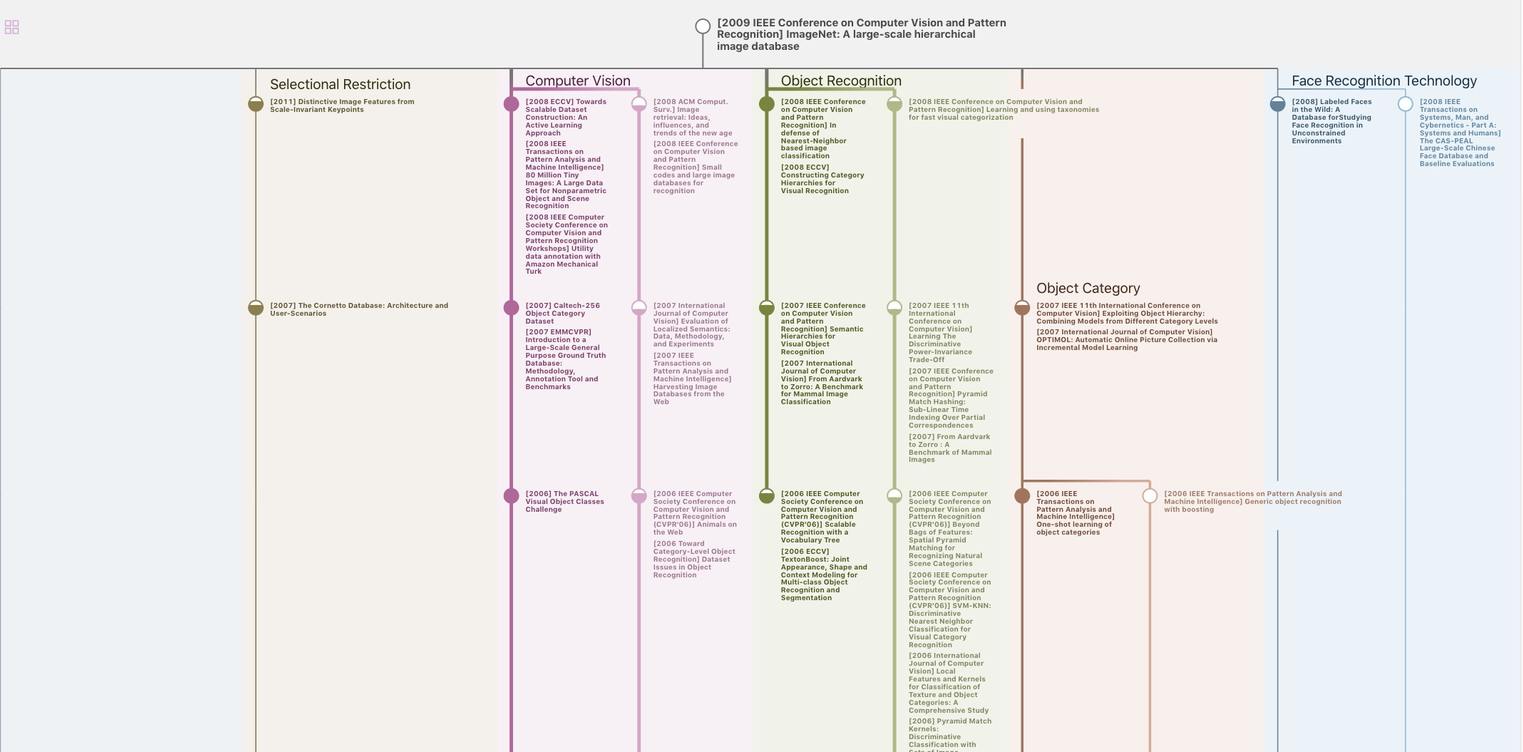
生成溯源树,研究论文发展脉络
Chat Paper
正在生成论文摘要