Dichotomize and Generalize: PAC-Bayesian Binary Activated Deep Neural Networks.
ADVANCES IN NEURAL INFORMATION PROCESSING SYSTEMS 32 (NIPS 2019)(2019)
摘要
We present a comprehensive study of multilayer neural networks with binary activation, relying on the PAC-Bayesian theory. Our contributions are twofold: (i) we develop an end-to-end framework to train a binary activated deep neural network, (ii) we provide nonvacuous PAC-Bayesian generalization bounds for binary activated deep neural networks. Our results are obtained by minimizing the expected loss of an architecture-dependent aggregation of binary activated deep neural networks. Our analysis inherently overcomes the fact that binary activation function is non-differentiable. The performance of our approach is assessed on a thorough numerical experiment protocol on real-life datasets.
更多查看译文
关键词
bayesian theory
AI 理解论文
溯源树
样例
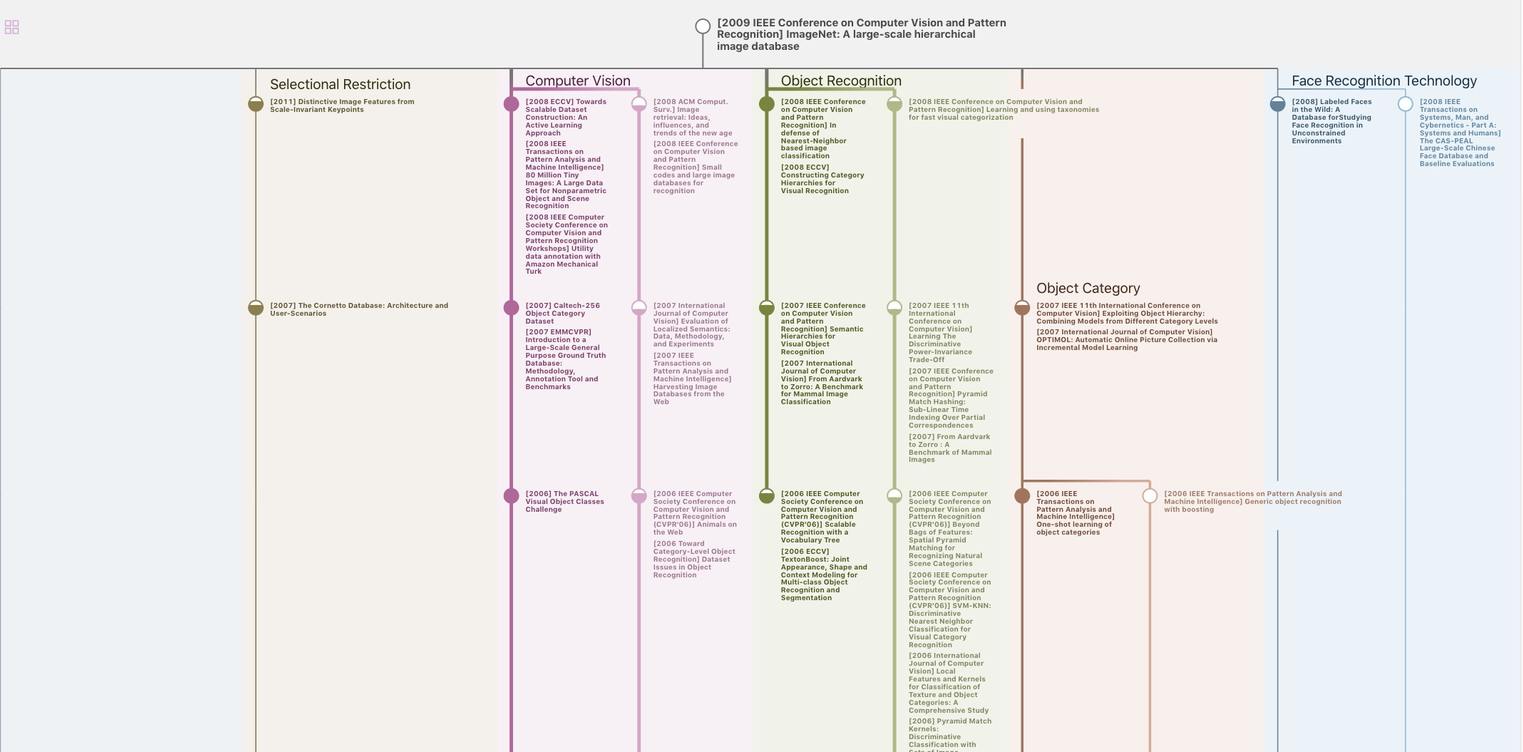
生成溯源树,研究论文发展脉络
Chat Paper
正在生成论文摘要