DEMEA: Deep Mesh Autoencoders for Non-rigidly Deforming Objects
CoRR(2019)
摘要
Mesh autoencoders are commonly used for dimensionality reduction, sampling and mesh modeling. We propose a general-purpose DEep MEsh Autoencoder (DEMEA) which adds a novel embedded deformation layer to a graph-convolutional mesh autoencoder. The embedded deformation layer (EDL) is a differentiable deformable geometric proxy which explicitly models point displacements of non-rigid deformations in a lower dimensional space and serves as a local rigidity regularizer. DEMEA decouples the parameterization of the deformation from the final mesh resolution since the deformation is defined over a lower dimensional embedded deformation graph. We perform a large-scale study on four different datasets of deformable objects. Reasoning about the local rigidity of meshes using EDL allows us to achieve higher-quality results for highly deformable objects, compared to directly regressing vertex positions. We demonstrate multiple applications of DEMEA, including non-rigid 3D reconstruction from depth and shading cues, non-rigid surface tracking, as well as the transfer of deformations over different meshes.
更多查看译文
关键词
Auto-encoding,Embedded deformation,Non-rigid tracking
AI 理解论文
溯源树
样例
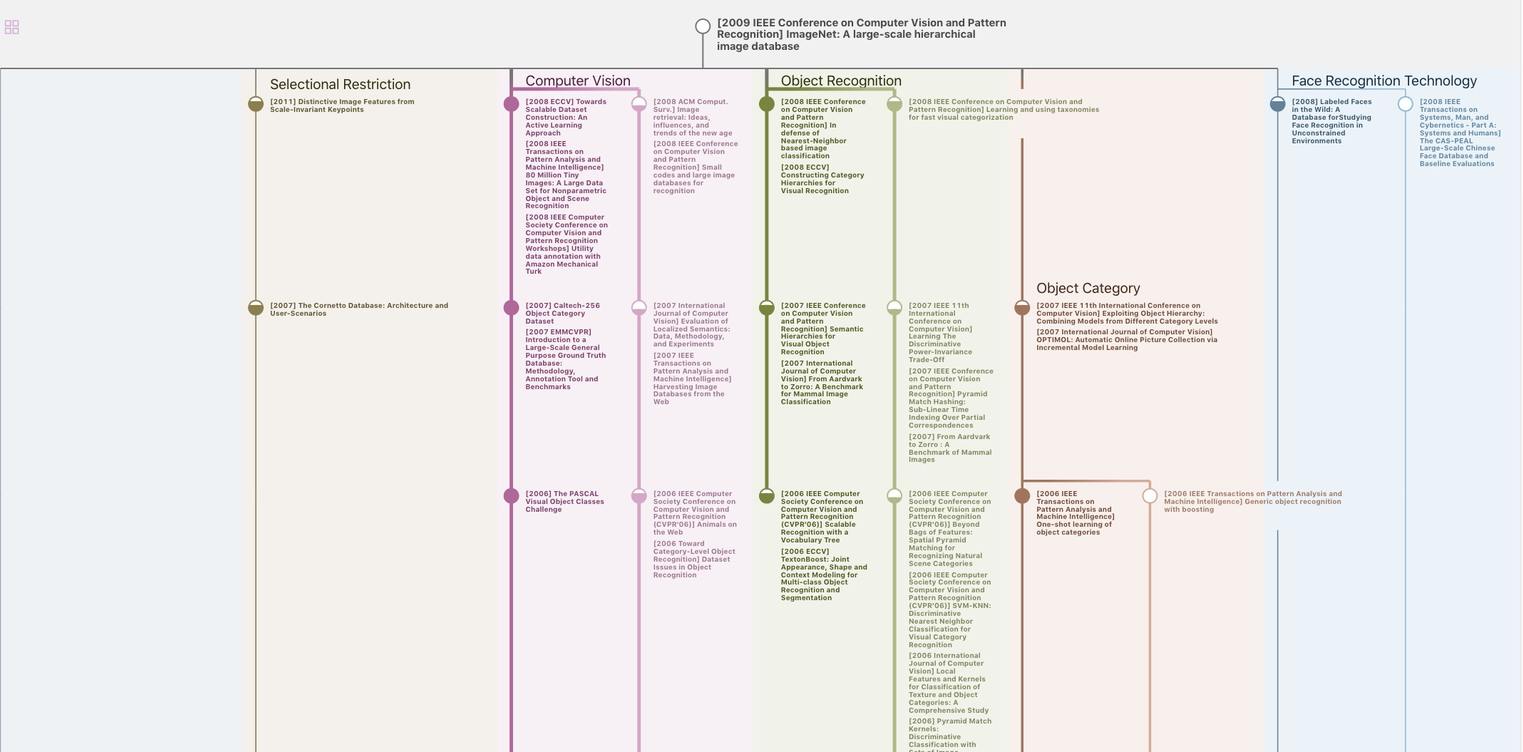
生成溯源树,研究论文发展脉络
Chat Paper
正在生成论文摘要