Harnessing mass spectra data using KNN principle: diagnosing Alzheimer's disease
ACM SIGBioinformatics Record(2018)
摘要
A high level of expertise, rigorous algorithms and methods are needed to adequately mine and harness Mass Spectrometer generated data due to its unique nature and structure. Hitherto, peptide ions are matched with theoretical results and/or public databases in order to identify expressed proteins in analyzed protein source samples, but this is done on a spectrum by spectrum basis. In this study, we present a mechanism that extends the principle of K-nearest neighbor algorithm for mining pools of mass spectrometer saliva data towards discovering and characterizing patterns for diagnosing Alzheimer's disease. The methodology discusses feature selection by correlation matrix, matrix to vector decomposition, an extension of Euclidean distance formula, and successfully classifies donor samples into the three stages of Alzheimer's disease with over 85% accuracy without collaborating clinical records.
更多查看译文
关键词
Alzheimer's disease diagnosis, feature matrix, jackknifing, mass spectra data
AI 理解论文
溯源树
样例
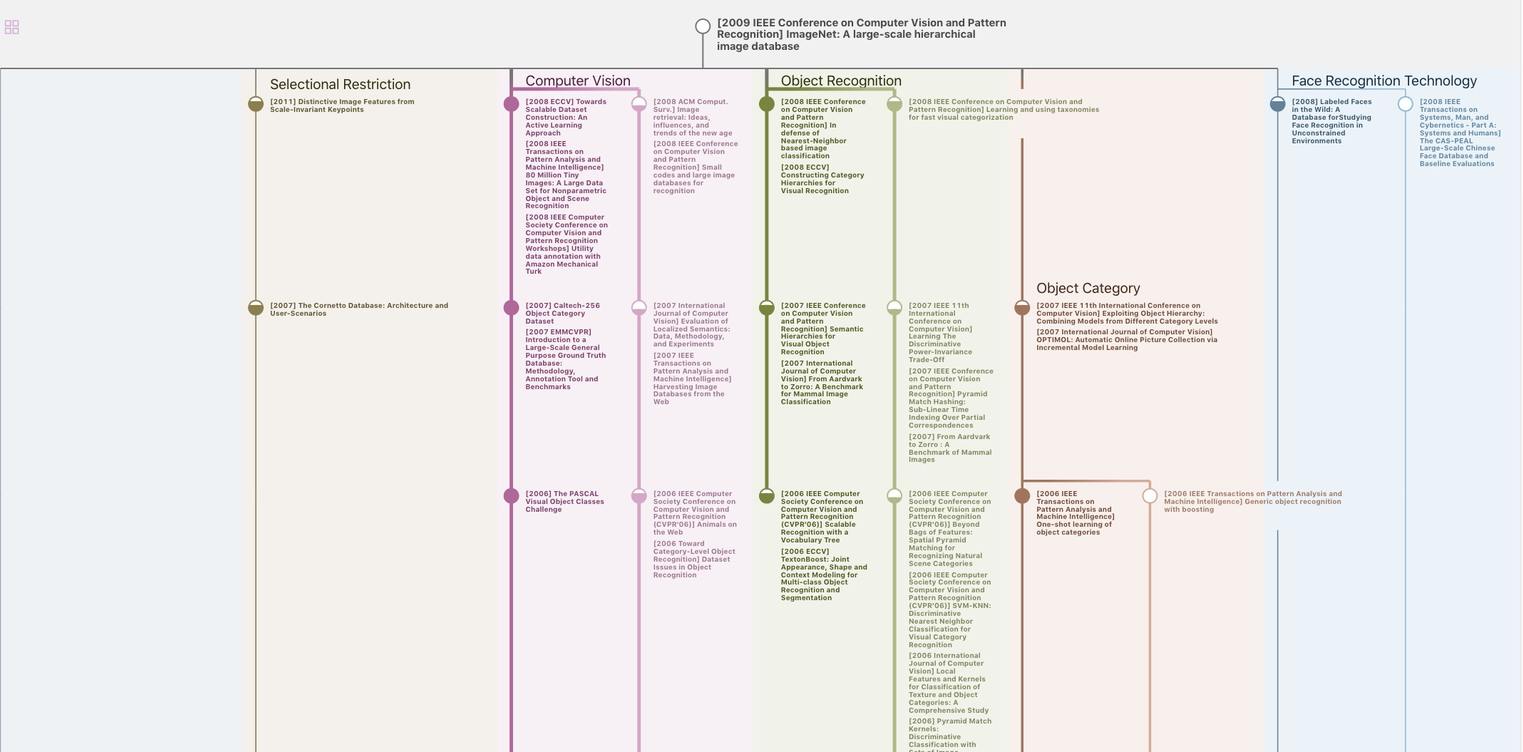
生成溯源树,研究论文发展脉络
Chat Paper
正在生成论文摘要