Adaptive Superpixel Generation for SAR Images With Linear Feature Clustering and Edge Constraint
IEEE Transactions on Geoscience and Remote Sensing(2019)
摘要
Due to the speckle noise and complex geometric distortions within SAR images, it is still a challenge to develop a stable method that can produce superpixels with both high boundary adherence and visual compactness with low computational costs at the same time. In this paper, we propose an adaptive superpixel generation approach with linear feature clustering and edge constraint for synthetic aperture radar (SAR) images, which consists of three stages. First, the local gradient ratio pattern of each pixel in SAR imagery is extracted as features, which was previously proposed by us for SAR target recognition and has been proven to be insensitive to speckle noise. Second, we propose to use the feature-ratio-based edge detector with Gauss-shaped window instead of the traditional rectangle-shaped window to obtain the edge strength map and final edges for SAR images. Finally, a modified normalized cut (Ncut)-based superpixel generation strategy is adopted using a distance metric that simultaneously measures both the feature similarity and space proximity. In this strategy, we approximate the similarity measure through a positive semidefinite kernel function rather than directly using the traditional eigen-based algorithm. Therefore, the objective functions of weighted local
K-
means and Ncuts can achieve the same optimum point by appropriately weighting each point in this feature space, which greatly reduces the computation cost. During the linear feature clustering, the coefficient of variation is used to automatically determine the tradeoff factor between the feature similarity and space proximity, which helps change the superpixel shape and size adaptively according to the image homogeneity. Furthermore, the edge information is also introduced to constrain the clustering for the sake of high boundary adherence. By bridging the local K-means clustering and Ncuts, as well as the benefits of edge constraint, our method not only produces superpixels with good boundary adherence but also captures the global image structure information. Experimental results with simulated and real SAR images demonstrate the effectiveness of our proposed method, which performs better than other state-of-the-art algorithms.
更多查看译文
关键词
Image edge detection,Synthetic aperture radar,Feature extraction,Speckle,Detectors,Shape,Microsoft Windows
AI 理解论文
溯源树
样例
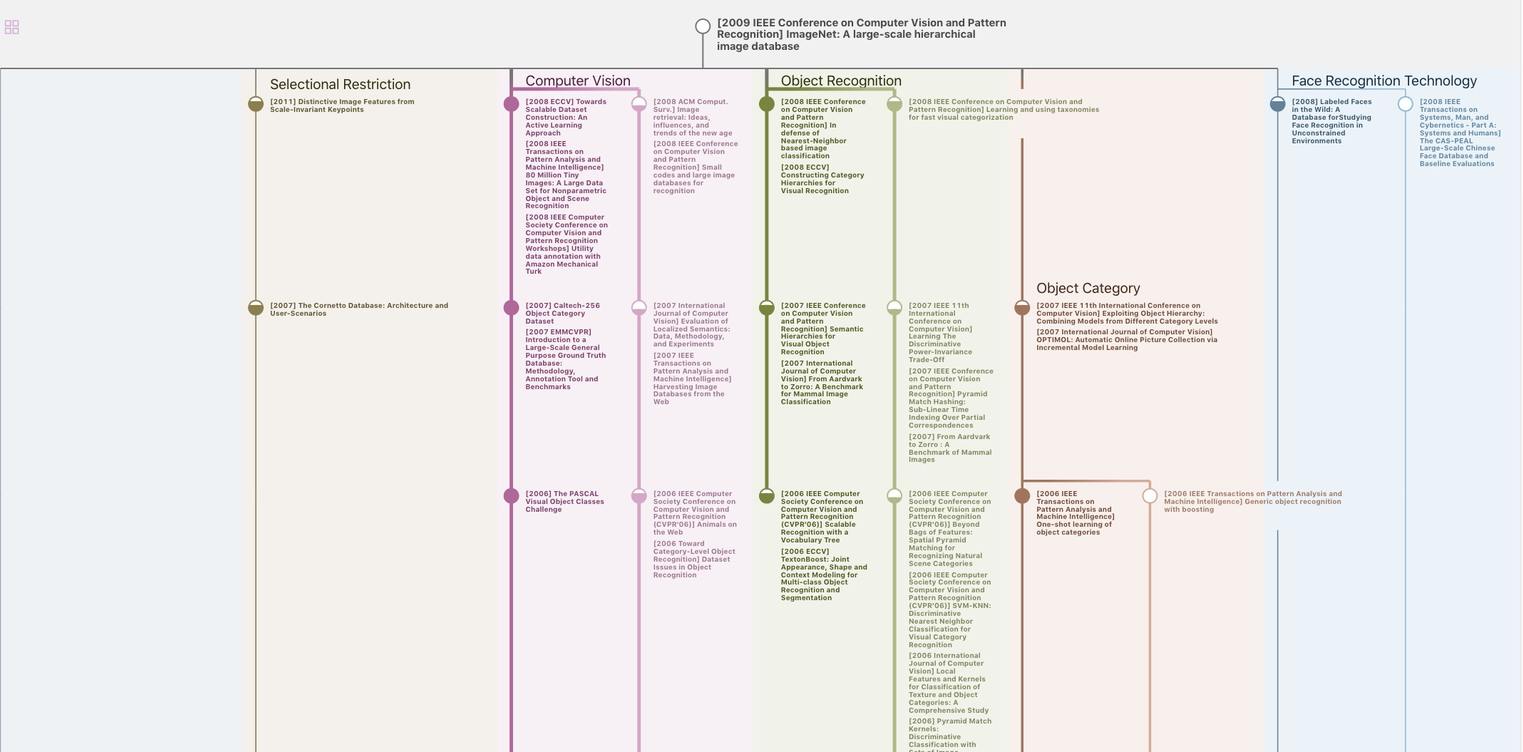
生成溯源树,研究论文发展脉络
Chat Paper
正在生成论文摘要