Data Augmentation for X-Ray Prohibited Item Images Using Generative Adversarial Networks.
IEEE ACCESS(2019)
摘要
Recognizing prohibited items automatically is of great significance for intelligent X-ray baggage security screening. Convolutional neural networks (CNNs), with the support of big training data, have been verified as the powerful models capable of reliably detecting the expected objects in images. Therefore, building a specific CNN model working reliably on prohibited item detection also requires large amounts of labeled item image data. Unfortunately, the current X-ray baggage image database is not big enough in count and diversity for CNN model training. In this paper, we propose a novel method for X-ray prohibited item data augmentation using generative adversarial networks (GANs). The prohibited items are first extracted from X-ray baggage images using a K-nearest neighbor matting scheme. Then, the poses of the obtained item images are estimated using a space rectangular coordinate system and categorized into four or eight classes for constructing a training database. For generating the realistic samples reliably, different GAN models are evaluated using Frechet Inception Distance scores, and some important tips of handling GAN training in X-ray prohibited item image generation are reported. Finally, to verify whether the generated images belong to its corresponding class or not, a cross-validation scheme based on a CNN model is implemented. The experimental results show that most of the generated images can be classified correctly by the CNN model. This implies that the generated prohibited item images can be used as the extended samples to augment an X-ray image database.
更多查看译文
关键词
Data augmentation,generative adversarial networks,image generation,prohibited item,X-ray image
AI 理解论文
溯源树
样例
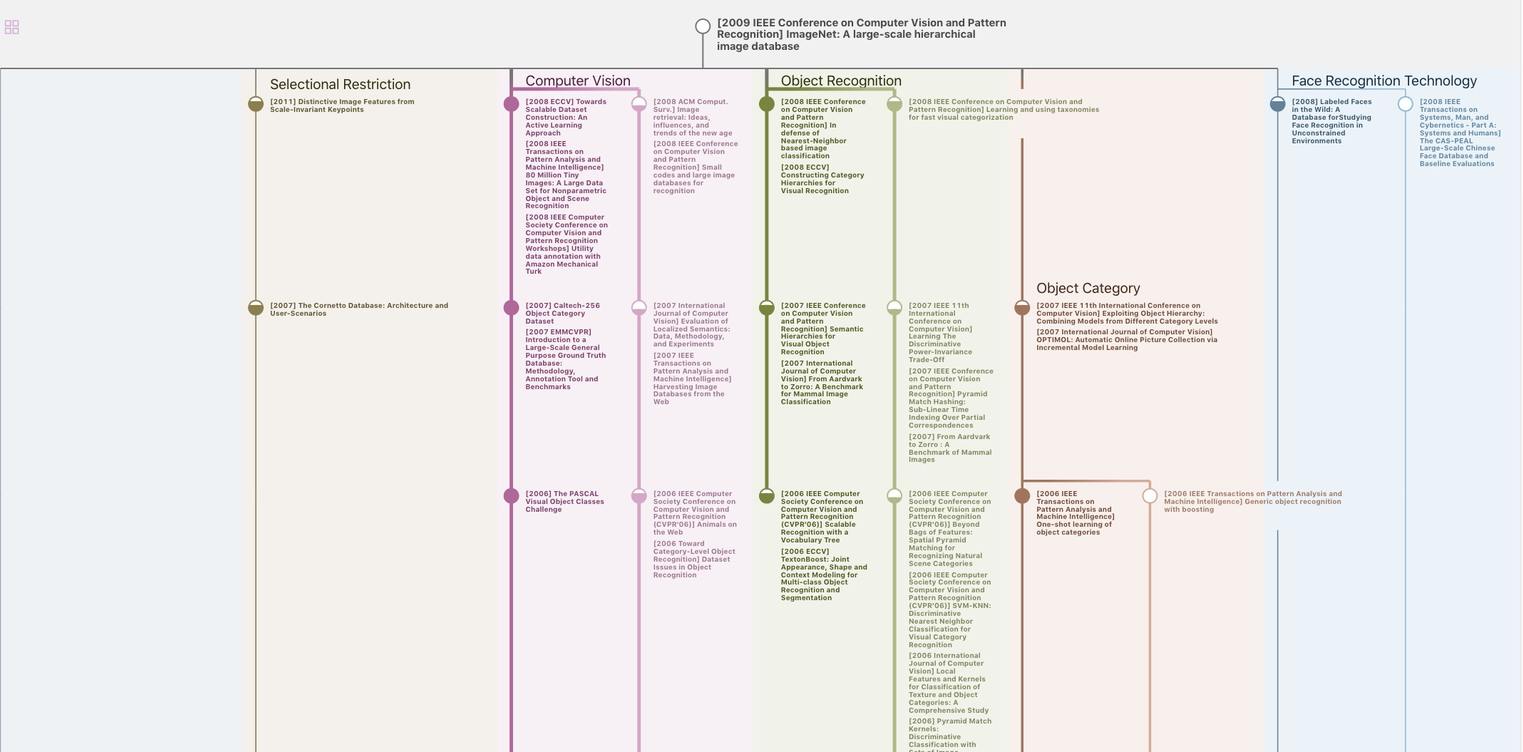
生成溯源树,研究论文发展脉络
Chat Paper
正在生成论文摘要